Open Access
ARTICLE
Load-Aware VM Migration Using Hypergraph Based CDB-LSTM
1 School of Computing, SASTRA Deemed-to-be University, Thanjavur, 613401, Tamilnadu, India
2 Center for Information Super Highway (CISH), School of Computing, SASTRA Deemed-to-be University, Thanjavur, 613401, Tamilnadu, India
* Corresponding Author: V. S. Shankar Sriram. Email:
Intelligent Automation & Soft Computing 2023, 35(3), 3279-3294. https://doi.org/10.32604/iasc.2023.023700
Received 17 September 2021; Accepted 04 November 2021; Issue published 17 August 2022
Abstract
Live Virtual Machine (VM) migration is one of the foremost techniques for progressing Cloud Data Centers’ (CDC) proficiency as it leads to better resource usage. The workload of CDC is often dynamic in nature, it is better to envisage the upcoming workload for early detection of overload status, underload status and to trigger the migration at an appropriate point wherein enough number of resources are available. Though various statistical and machine learning approaches are widely applied for resource usage prediction, they often failed to handle the increase of non-linear CDC data. To overcome this issue, a novel Hypergrah based Convolutional Deep Bi-Directional-Long Short Term Memory (CDB-LSTM) model is proposed. The CDB-LSTM adopts Helly property of Hypergraph and Savitzky–Golay (SG) filter to select informative samples and exclude noisy inference & outliers. The proposed approach optimizes resource usage prediction and reduces the number of migrations with minimal computational complexity during live VM migration. Further, the proposed prediction approach implements the correlation co-efficient measure to select the appropriate destination server for VM migration. A Hypergraph based CDB-LSTM was validated using Google cluster dataset and compared with state-of-the-art approaches in terms of various evaluation metrics.
Keywords
Cite This Article
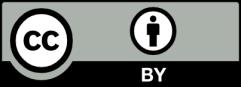