Open Access
ARTICLE
A Nonlinear Spatiotemporal Optimization Method of Hypergraph Convolution Networks for Traffic Prediction
1 Hangzhou Innovation Institute, Beihang University, Hangzhou, 310051, China
2 Yuanpei College, Shaoxing University, Shaoxing, 312000, China
3 Department of Computer Science, Zhijiang College of Zhejiang University of Technology, Shaoxing, 312000, China
4 School of Economics, Fudan University, Shanghai, 200433, China
* Corresponding Authors: Chao Li. Email: ; Jingjing Chen. Email:
Intelligent Automation & Soft Computing 2023, 37(3), 3083-3100. https://doi.org/10.32604/iasc.2023.040517
Received 21 March 2023; Accepted 17 June 2023; Issue published 11 September 2023
Abstract
Traffic prediction is a necessary function in intelligent transportation systems to alleviate traffic congestion. Graph learning methods mainly focus on the spatiotemporal dimension, but ignore the nonlinear movement of traffic prediction and the high-order relationships among various kinds of road segments. There exist two issues: 1) deep integration of the spatiotemporal information and 2) global spatial dependencies for structural properties. To address these issues, we propose a nonlinear spatiotemporal optimization method, which introduces hypergraph convolution networks (HGCN). The method utilizes the higher-order spatial features of the road network captured by HGCN, and dynamically integrates them with the historical data to weigh the influence of spatiotemporal dependencies. On this basis, an extended Kalman filter is used to improve the accuracy of traffic prediction. In this study, a set of experiments were conducted on the real-world dataset in Chengdu, China. The result showed that the proposed method is feasible and accurate by two different time steps. Especially at the 15-minute time step, compared with the second-best method, the proposed method achieved 3.0%, 11.7%, and 9.0% improvements in RMSE, MAE, and MAPE, respectively.Keywords
Cite This Article
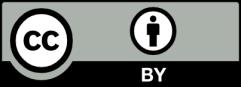