Open Access
ARTICLE
Enhanced Feature Fusion Segmentation for Tumor Detection Using Intelligent Techniques
1 Department of Information and Communication Engineering, Anna University, Chennai, 600025, Tamilnadu, India
2 Department of Electrical and Electronics Engineering, K. S. Rangasamy College of Technology, Tiruchengode, 637215, Tamilnadu, India
* Corresponding Author: R. Radha. Email:
Intelligent Automation & Soft Computing 2023, 35(3), 3113-3127. https://doi.org/10.32604/iasc.2023.030667
Received 30 March 2022; Accepted 09 May 2022; Issue published 17 August 2022
Abstract
In the field of diagnosis of medical images the challenge lies in tracking and identifying the defective cells and the extent of the defective region within the complex structure of a brain cavity. Locating the defective cells precisely during the diagnosis phase helps to fight the greatest exterminator of mankind. Early detection of these defective cells requires an accurate computer-aided diagnostic system (CAD) that supports early treatment and promotes survival rates of patients. An earlier version of CAD systems relies greatly on the expertise of radiologist and it consumed more time to identify the defective region. The manuscript takes the efficacy of coalescing features like intensity, shape, and texture of the magnetic resonance image (MRI). In the Enhanced Feature Fusion Segmentation based classification method (EEFS) the image is enhanced and segmented to extract the prominent features. To bring out the desired effect the EEFS method uses Enhanced Local Binary Pattern (EnLBP), Partisan Gray Level Co-occurrence Matrix Histogram of Oriented Gradients (PGLCMHOG), and iGrab cut method to segment image. These prominent features along with deep features are coalesced to provide a single-dimensional feature vector that is effectively used for prediction. The coalesced vector is used with the existing classifiers to compare the results of these classifiers with that of the generated vector. The generated vector provides promising results with commendably less computatio nal time for pre-processing and classification of MR medical images.Keywords
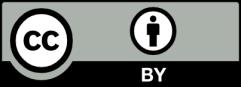