Open Access
ARTICLE
A Deep Learning Model of Traffic Signs in Panoramic Images Detection
1
International University, Ho Chi Minh City, Vietnam-Vietnam National University, Ho Chi Minh City, 700000, Vietnam
2 Department of Computer Science, The Superior University, Lahore, Pakistan
3 Ho Chi Minh City University of Foreign Languages and Information Technology, Ho Chi Minh City, 700000, Vietnam
* Corresponding Author: Thien Khai Tran. Email:
Intelligent Automation & Soft Computing 2023, 37(1), 401-418. https://doi.org/10.32604/iasc.2023.036981
Received 18 October 2022; Accepted 13 January 2023; Issue published 29 April 2023
Abstract
To pursue the ideal of a safe high-tech society in a time when traffic accidents are frequent, the traffic signs detection system has become one of the necessary topics in recent years and in the future. The ultimate goal of this research is to identify and classify the types of traffic signs in a panoramic image. To accomplish this goal, the paper proposes a new model for traffic sign detection based on the Convolutional Neural Network for comprehensive traffic sign classification and Mask Region-based Convolutional Neural Networks (R-CNN) implementation for identifying and extracting signs in panoramic images. Data augmentation and normalization of the images are also applied to assist in classifying better even if old traffic signs are degraded, and considerably minimize the rates of discovering the extra boxes. The proposed model is tested on both the testing dataset and the actual images and gets 94.5% of the correct signs recognition rate, the classification rate of those signs discovered was 99.41% and the rate of false signs was only around 0.11.Keywords
Cite This Article
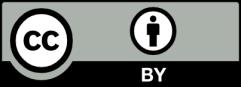