Open Access
ARTICLE
Using Digital Twin to Diagnose Faults in Braiding Machinery Based on IoT
1 Chengyi University College, Jimei University, Xiamen, 361021, China
2 College of Harbor and Coastal Engineering, Jimei University, Xiamen, 361021, China
* Corresponding Author: Huangbin Lin. Email:
(This article belongs to the Special Issue: Neutrosophic Theories in Intelligent Decision Making, Management and Engineering)
Intelligent Automation & Soft Computing 2023, 37(2), 1363-1379. https://doi.org/10.32604/iasc.2023.038601
Received 20 December 2022; Accepted 10 March 2023; Issue published 21 June 2023
Abstract
The digital twin (DT) includes real-time data analytics based on the actual product or manufacturing processing parameters. Data from digital twins can predict asset maintenance requirements ahead of time. This saves money by decreasing operating expenses and asset downtime, which improves company efficiency. In this paper, a digital twin in braiding machinery based on IoT (DTBM-IoT) used to diagnose faults. When an imbalance fault occurs, the system gathers experimental data. After that, the information is sent into a digital win model of the rotor system to see whether it can quantify and locate imbalance for defect detection. It is possible to anticipate asset maintenance requirements with DT technology by IoT (Internet of Things) sensors, XR(X-Ray) capabilities, and AI-powered analytics. A DT model’s appropriate design and flexibility remain difficult because of the nonlinear dynamics and unpredictability inherent in the degrading process of equipment. The results indicate that the DT in braiding machinery developed allows for precise diagnostic and dynamic deterioration analysis. At least there is 37% growth in efficiency over conventional approaches.Keywords
As for maintenance and advancement, the smart factory has been a hot issue in industrial data analysis. The notion of smart factories has been the subject of research and application in the latest days. Smart factory encompasses smart goods, smart production, digitalization, and other features and their improvement and interaction [1]. Although there are variances in these diverse elements from the standpoint of technological process, they are the same, such as the unification of the human-information-physical system. The thinking, developing, building, monitoring, updating, and retooling phases of a smart company’s life cycle are included [2,3]. Each stage has its own set of technological difficulties.
Employees, technology, supplies, procedures, the atmosphere, and information in manufacturing must all be linked and engaged in various ways throughout the entire life cycle. As a result, several approaches and models must be employed to integrate information skills and technical processes [4]. A younger generation of data technology and smart assembly lines, which incorporate multiattribute, multifunctional, and multi-application mechanics, are driving the development of digital twins. Physical objects’ characteristics, behaviour, creation process, and efficiency are described and modelled using digital twin technologies [5,6]. Modern sensors, industrial networks, the Internet of Things (IoT), advanced analytics, deep learning, and other innovations are employed simultaneously to consider and fulfil production chain tracking, prediction, data gathering, and other services.
The digital representation can test system efficiency in a semi-physical simulator and locate and resolve faults quickly [7]. Constructing a digital copy of the production line, electronically portraying the industrial manufacturing atmosphere, and undertaking device prognosis, simulation techniques, and other modelling forecasts in the simulated digital world can help deter grave ramifications from on-site mistakes and output anomalies in industrial output [8]. Not only can manufacturing equipment be observed for defect prediction and prompt maintenance using digital twin technologies, but it can still be controlled and maintained remotely, lowering operational costs, and increasing safety.
This research integrates digital twin innovation with artificial intelligence (AI) technology. It uses the controller of aircraft truss smart construction in double motion simultaneous optimization while analysing the digital twin network of the smart manufacturing line [9]. The algorithm improved the quality of truss linking rod failure rate detection, and the digital twin concept contributes to the improvement and speed of the smart assembling controller [10].
The current study’s findings cantered on the notion and utility of digital twins. There is a shortage of digital twin studies, action plans, and case studies throughout the life span of industrial automation. As a result, this paper builds a physical framework for a micro industrial internet of things based on the requirements of the micro smart manufacturing to confirm the validity and impact of digital twin innovation to realize intelligent collected data, smart process flow, procedural knowledge, and other operations using digital twin innovation. It offers small smart factory businesses with academic and conceptual help.
The following are the contributions of this article:
• It employs a diagnostic step to recognize the importance of proactive risk management maintenance.
• It uses the virtual reference architecture to infer equipment failure. Improved interaction and cooperation with a physical object achieve device synchronization and forecast accuracy.
• In many real settings, the suggested system overcomes the concerns of inadequate data retraining and dissemination. The digital reference architecture uses a deep neural network to deliberately imitate the necessary process at a minimal cost to develop a genuine diagnosis model.
• A real-time situation is chosen to compare the suggested system’s effectiveness to other current methods to achieve critical metrics.
The following shows the rest of the paper: Section 2 illustrates the digital twin’s background and complexity. Section 3 proposed the digital twin in braiding machinery based on IoT (DTBM-IoT) system and its implementation procedure. Section 4 discusses the software outcomes of the proposed system and its effectiveness. Section 5 lists the findings of the proposed system and its limitations.
The breadth of sustainable research and decision-support options has been expanded thanks to smart manufacturing information systems development. Various convergence techniques have emerged to standardize industrial concepts, including artificial intelligence (AI) technology, industrial IoT, neurodevelopmental disorders, cloud services, predictive analytics, and improved reconfiguration systems [11]. Gaikwad et al. uncovered an advanced computer program to begin a digital sense to architectural or biomedical applications [12]. To replicate the machinery operations, it contained numerous classifications like mining, coding, information retrieval, logic, training, text analysis, and formal verification. It has recently emerged as a significant approach in several new fields, such as the share market, banking, medical, education, robotics, and farming. The depiction of numerous data analyses such as training, segmentation, classifying, cooperation, and screening had greatly broadened the AI scope [13].
Smart production includes AI’s technological techniques. The need for environmental protection drove the technical techniques needed to satisfy Industry 4.0 standards. These tactics used a learning process to develop a categorization framework to investigate influential features to comprehend theme regions suggested by Negri et al. [14]. The international market involved data-centric communication to foster performance, adaptability, and efficient digitalization. The production unit analysed driven methodology and operation while developing a business-oriented software, which fitters’ system problems to meet worldwide demand [15]. It has been designed with specific characteristics to consider the adaptation concept, which provided crucial capabilities such as physical and cyber assets for Industry 4.0. Industry 4.0 has recently taken on an electronic copy to handle business difficulties, data stagnation, converging ratio, and ecology suggested by Kaur et al. [16].
Modern engineering altered the engineer’s engagement to portray tangible assets digitally. It supported the digital twin as a service as part of the Industry 4.0 revolution. It was always evolving to distinguish between real and virtual assets, and it employed a variety of paradigms to meet greater international concerns [17]. It had not yet been properly investigated. As a result, it was regarded as a comprehensive guide to Industry 4.0. An emerging body of research and modelling enabled technological change and innovation. Several machines to realize the technological problems suggested by Liu et al. [18]. It is important to adopt automation to check the state of the equipment. The training mechanism analysed the mechanical tools’ status to remedy the limiting issues. The experimental approach examined cutting variables, they examine cutting variables, and corresponding tool procedures were examined in industrial practice, which emerged due to sensors and IoT in the property sector [19]. A substantial solution derived from the industrial industry has emerged: cyber-physical networks (CPS). CPS, also referred to as digital twins (DT), is a ground-breaking digital vision in which innovative technologies were used to generate a virtual design that fits identically to its real twin [20].
Internet of Things (IoT) was a game-changing technological breakthrough that has enabled faces and things to interact on a level and at an unfathomable rate even a generation later. It offered new tactics to improve living conditions, enabling linked instruments to create independent decisions using analytics and deep learning suggested by Ladj et al. [21]. It correctly advised making the best possible options, such as an emergency or significant failure. The techniques to fault prediction of tool systems have improved as technology has progressed, and data-driven techniques to prediction were actively being researched [22]. A new approach for motor bearings monitoring systems and problem diagnostics is adopted to under sample seismic data. The group data management approach proposed the prediction and diagnosis method of milling cutter loss in a processes computer. Methods for diagnosis and prediction rely on empirical processes that have been developed. The model-based fault prediction focused on the model and technique provided above. Current fault forecasting techniques had several distinct benefits suggested by Redelinghuys et al. [23]. However, there are a few drawbacks: 1) some systems were frequently too chaotic to model. 2) the technique heavily relied on professional expertise, and many documents were necessary. 3) the model lacked a strategy to refresh the prototype with fresh test data.
As a result, a Digital Twin comparison model was examined to aid problem identification and prediction research. The material from the detectors was analyzed and processed using large datasets. The central monitoring machine used CPS to take the analogy signals and direct the physical machinery to the following stages [24]. Machine learning provided the model for the failure prediction method. This strategy incorporated both a data-driven and a model-based strategy. It was feasible to create an effective virtual and real-world interaction model that accurately reflected holograms’ motion form and substance composition suggested by Jiang et al. [25]. Moreover, the projected outcomes were easier to visualize in 3 directions, which might aid operators in comprehending the prognosis outcomes.
In general, DT used in detection primarily addresses trade, which presumed that training examples must be distributed similarly. Minimal training information was also a source of worry. The vast databases were utilized to pre-train an initial diagnostic model, fine-tuned with modest approaches’ shortcoming sites. However, neither of them contemplated the digital twin compatible with its physical counterpart and provided a considerable quantity of defect data without incurring breakdowns. As a result, this research presented digital-twin-assisted problem diagnostics. It utilized a combination of both deep neural networks and digital twin technologies to achieve real-time surveillance, rapid response, and maintenance schedules at the end.
3 Digital Twin in Braiding Machinery Based on IoT
A digital twin is a computer simulation of a real-world physical item in use. A model like this is used in real-time to regulate and improve the behaviour of real property by detecting abnormalities, efficiencies, or the potential of certain future occurrences. The development of a computer simulation of the pump can be done in two ways.
• Modeling from first principles
White-box modeling, for example, of the gear pump movements, is a first-principles-driven modeling method that involves constructing a working prototype subject to the laws of nature. SimscapeTM, an acausal physical modeling formalism that provides a good process for constructing these physics-based simulations, is used to create a first-principles forecasting methodology.
• Data-driven modeling
Data-driven modeling methods are on the opposite extreme, relying on deep learning or network detection techniques to train or identify models from measurable data about the tangible commodity in use. Textual modeling languages like MATLAB are ideal for creating data-driven systems. To demonstrate the effectiveness of the techniques, it creates both physics-based and data-driven integration versions of the pump. Simulink and State flow, technologies for schematic diagram and state chart modelling correspondingly, can be used to simulate control system operations in the programming logic circuit (PLC).
The direct tool measuring method is used to cut and hole at high scan rates. Signal to the wire, which connects two connections like standard and measurements, is one of the scanner’s most important features. The measuring (hot) connection is formed by two different metals connecting at one side, while the references (cold) junction is formed by the copper wire and the thermometer metals. Thorough testing using a k-type thermostatic sensor is conducted to examine the high cutting test. Modern based on virtual realities, mixed reality, and public cloud arise to make it much more device-specific in terms of thermocouple position in the product. It uses a digital twin to change the diagnostic problem pattern, allowing for improved online and offline interactions.
The architecture of the proposed DTBM-IoT system is depicted in Fig. 1. It has four units: industry physical space, communication space, digital twin, and user space. It contains a virtual model to investigate physical elements like laws, behaviour, and geometry. It employs a proactive design methodology to ensure that physical components that gather and transmit digital reality in real-time employment are expected. The cooperative operation might ensure the digital model’s cost-effectiveness, which would boost the real organizations’ efficiency. It depicts the suggested method, dividing intellectual growth and maintenance schedules into two distinct phases. The first step creates a virtual engineering and construction platform that uses selected features, information processing, resource utilization, and industry standards. The latter devises a method for testing, validating, and optimizing the digital world.
Figure 1: The architecture of the proposed DTBM-IoT system
As a result, an appropriate fault detection model is created to assess the nonstandard factors embedded in the digital twin to increase efficiency levels and anticipate the real chance of failing. The visual seeks to achieve formulates the following in the analysis software. It creates physical things to link virtual counterparts in a diagnostic model to convey supervised learning. The suggested system properly examines the stages of the machine operation, irrespective of the standard defect detection. The virtualized entities work together to adjust to the operating restrictions.
3.1 Intellectual Development in Fault Diagnosis
A dynamic detection framework model is constructed to represent the physical things in this step. It investigates probable breakdowns to mimic manufacturing under regular conditions. The volume of data must be monitored closely, gathered, and analyzed accordingly, with feature sets extracted via unlabeled data. An uncontrolled system called Autoencoder is recommended to imitate the enormous volume of unsupervised learning. It learns the knowing characteristics from the input nodes using hidden units. A nonlinear encoding function reduces the model complexity to increase precision. The Autoencoder implements the sparsity restrictions to deactivate the concealed inputs. It could load the input form in reading the number of control inputs, which is bigger than the number of input neurons available. Variables of this paper are shown in Table 1.
The stacking sparsed encoder pulls the many characteristics of a sparse image input to improve the network’s skills in a deep neural network (DNN). It uses a greedy technique to train the hidden units. It uses the appropriate value to construct the continuous incremental function
where R(.) denotes the punishment for mistake restoration, and
3.2 Proactive Maintenance in Fault Diagnosis
Improper functioning, aging materials, and climate changes arise during development. As a result, accurate surveillance and failure forecasting are critical for improving the manufacturing process. A machine learning technique is used to infer the state of the control process, which infers the hierarchy characteristics of any data collection. When any commercial process tries to discover operational flaws, the production method can store high amounts of information for DNN. The domain adaptation process is used to produce an efficient solution. It creates an appropriate learning model to recover the targeted domain’s high-level abstraction, which transfers various data distributions.
The fault detection model of the proposed DTBM-IoT system is shown in Fig. 2. It uses virtual space from the physical space using a digital twin. The trained DNN model is used, and deep transfer learning is used to reduce the error at the output. It tries to share data gathering and a disastrous condition that extracts data accurately using the learning approach. In addition, this phase includes maintenance schedules to transfer inaccurate data from simulated results. It is possible to transfer information from the digital to the material realm.
Figure 2: Fault detection model of the proposed DTBM-IoT system
Consequently, the precise data needed to enhance prediction performance can be efficiently taught. It’s not the same as a small materials autoencoder with an adopting layer between extracting features. To achieve a strong categorization, it reduces the dispersion length from sender to receiver domain. A good categorization using a labelled set of statistics from the online and offline space could overcome the fitting problem issue. The labelled data framework represents
where
G is the global ability to foster the Hilbert field, and
3.3 The Synthetic Nature of the Digital Twin Model
A Digital Twin is a live study of the physical environment supported by smart systems such as multi-physics modelling, deep learning, virtual reality, and cloud platform. It enables constant adaptation to climatic changes or processes while delivering the greatest possible commercial results. It has much promise for improving ongoing maintenance and speeding up the development of new products.
A new conceptual framework associated with digital twin technology from library research illustrates a scheme data integration model in six innovation features, four business software, four benefits, and three factors that drove the scheme data integration model.
Various features of technologies (networked embedded networks, IoT, identification number), allocation of resources (clean energy), and procedures are among the features of the data integration concept (grid computing, diverse data, connectivity, and physical connection are all examples of cloud systems). Cyber-Physical technologies, as a younger breed of processes with both cognitive and mechanical abilities, can help humans connect to the digital environment. To accomplish the tasks of identifying risks, fault detection, and safety practices, the IoT analyses vast volumes of data of various sorts through sensing devices (like radio frequency identity (RFID) tags, infrared induction, and global positioning system (GPS) navigation, among others). The new suggested digital twin architecture of tool systems offers some security mechanisms (process control/monitoring, clinical fault diagnosis, alarm handling, etc.).
Digital Twin can communicate with humans and machines and respond to the environment and actual objects in real-time. It has a one-of-a-kind identity that is solely linked to the utility system. The data integration approach incorporates AI (ontologies, computer vision, and learning techniques, among other things). The feedback loop of the digital twin concept effectively assists in fixing faults in the tool design phase, as per the model’s feedback controller. Digital Twin creates a model of the tool mechanism, defect awareness and can pinpoint if the feed scheme or the blade fails. Creative education encourages individuals to set higher standards for smart factories and expands their horizons. The more precise data processing technology is required to accommodate a huge volume of data handling. The code of behavior assures that the digital twin is accurate and consistent.
3.4 Data Analytics Process of Tool System
Deep learning algorithms play a crucial role in dynamically gathering and analyzing data, finding patterns, and passing judgment by mining information from data collected. Advanced statistics, diagnostics analytics, prescriptive modeling, and predictive analysis are all types of information analytics that can be done. Advanced statistics tries to capture the product’s circumstances, surroundings, and operational data to describe what occurs. The predictive analysis studies the underlying cause and reveals why a product’s efficiency is diminished, or an equipment breakdown occurs. With past data, machine learning uses statistical design to identify forecasts about the potential of future output or asset deterioration.
Because observational evidence of tool state is impossible, the observational model creates a regression analysis forecast function based on in-process data and machined surface state. Based on retrieved local characteristics, a bi-directional system is used to build an observational model to forecast tool, described in Eq. (6).
The concealed state matrix of the final units during left-to-right transmission is denoted by
The data in the intermediate range of the loop is lost. A weighted features average layer is added function, and a fully-connected level makes up most of the weighed element average layer. The averaging characteristics are created using consecutive characteristics and equivalent to the overall average of consecutive characteristics as the source of the weighed average additional relevant. The extracted features for weighted average are written in Eqs. (7)–(10).
where
The pictorial representation of the function
Figure 3: The pictorial representation of the function
The projected tool diameter value is denoted by
A simulation environment describes the fundamental tool of life development behavior as it evolves. This article views the real system change as a time information autoregression procedure. The network gets one scalar indicating the current wear rate and creates the other scalar reflecting subsequent tool wear. The human brain is then given a convolutional layer for forecasting. This technique is summed up and expressed in Eq. (12)
where
Most previous research on digital twins was restricted to three-dimensional structures, focusing solely on the physical model, digital prototype, and relationships. This work offers a new version called the five-dimensional data integration model of tool network that has a more precise estimation, more active surveillance, and forecast calculation approach and can communicate the projected outcomes more readily than the previous model. The instrument system’s primary building method is to create a digital model by combining data from various phases in the physical thing. An information environment and communication system are included in the new vehicle, based on a prior three-dimensional study. The digital twin instrument system is founded on the item tool network, which comprises several functional components (mechanics, computer, supplementary, and sensing devices) and their functioning state and operating circumstances. The virtual toolset system has a high level of realism.
The implementation flow of the proposed DTBM-IoT system is depicted in Fig. 4. It uses sensor data and programmable logic devices to process and analyze data in the digital domain. The local machine environment is implemented, the rotor movement is analyzed using the proposed method, and the outcomes are visualized to the remote client. In the five-dimensional digital twin concept, the data area consists mostly of design limits, process information, production process information, and feedback analysis information. It encourages the advancement of the tool operating system and improves the overall intelligence and accuracy of the core service.
Figure 4: The implementation flow of the proposed DTBM-IoT system
The new service in this design encompasses alarm monitoring, control activities, problem clinical diagnosis, and repair. Data mapped connects the virtual-entity modelling approach. In a wide sense, data mapping is the transformation of spatial data into the virtual realm, with knowledge and analysis acquired or sensed from the physical environment eventually being stored in the IoT device cloud computing system. Users’ encounters in the core service provide feedback and improve product manufacturing and design.
3.6 Digital Twin Model Construction
The digital twin designer’s design phases are as follows:
Gather essential data on the manufacturing process, such as the structure of the workshop, logistical routes, input materials, intermediary buffering, and finished goods output.
Adjust the starting model, build a simulation environment of the supply chain operating based on the mini-intelligent factory’s past data, and produce the simulation model.
The governing equations relating to the production process are changed based on recent data from the micro smart building, and the model creation and optimization are accomplished on the network simulator.
The final simulation must study the physical smart company’s success metrics. The load status of every station/buffer/transport line and the position of the production level problem are all included. Finally, the estimated values are updated based on the statistical analysis to maintain their compatibility with the micro intelligent company’s project status.
• Scheme design and implementation
Obtain data collected from the microscale smart manufacturing, download files, and compute the simulator inputs incorporating the micro intelligent industrial manufacturing line’s total output capability, the load condition of a specific station, the activity of the transit line, and so on. The smart mini manufacturing constantly works for 250 h over the prescribed production cycle of 15 days, producing 3000 goods for assessment.
A huge amount of precise data is required to build a digital twin technology with its functionalities. Micro smart manufacturing information is collected utilizing programmable logic controllers (PLCs), sensors, computer vision, and other devices.
The simulation’s output results would be used to determine the micro-physical clever company’s overall key metrics, like the complete production route’s power rating, modeling cycle terminal updates, buffer and public transit line success factors, as well as robotic systems, handling, upkeep, and knowledge facts of different operating conditions, like manufacturing line transmitting, handling standing, and material management condition. The content size, top-notch information, production plant batch, product cost, central processing unit (CPU) cycles, and production plant quantity of the purchased items can all be seen in specifics.
The proposed DTBM-IoT system is designed with the Internet of Things and deep neural network modules. The user visualizes the outcomes of the rotor moment in the remote location based on digital twin and virtualization software. The effectiveness of the proposed DTBM-IoT system is verified using the software in the next section.
Compared with technologies and tools for the digital twin by Qi et al. [26], this paper offers real-time simulation to study the machinery operation, including the end milling circumstances. It constructs a process architect and simulation models by connecting the equipment platform via a programmable logic controller. It recognizes situations like contact and connectivity. The tests suggest that the implemented new testbed can withstand machine speeds of up to 30,000 revolutions per minute. Furthermore, why can this smart tool holder assist drilling and grinding processes and maintain internal lubricating? It integrates communication information from the sensors and mechanical floors to investigate the workpiece’s state.
Figs. 5a and 5b show the accuracy analysis of the existing CPS system and the proposed DTBM-IoT system, respectively. The simulation analysis of both existing and proposed systems is done using the Matlab simulation tool under the same simulation environment. The outcomes are monitored concerning the epoch size, and the simulation accuracy is directly related to epoch size. The higher epoch leads. To higher processing of the same data and produces better simulation results with lower error with the help of the digital twins.
Figure 5: (a) Accuracy analysis of the existing CPS system. (b) Accuracy analysis of the proposed DTBM-IoT system
The execution time of the existing system and the proposed DTBM-IoT system are depicted by theoretical calculation in Table 3. The number of IoT devices are varied from a minimum of 10 to a maximum of 100 with a step size of 10 devices. The respective simulation execution time of the existing and proposed DTBM-IoT systems is evaluated and tabulated. The IoT devices enhance the connectivity and show better visualization effects to the remote user by the digital twin model. Still, the computation complexity of the entire system increases as the number of IoT devices is increased.
Table 2 shows the theoretical simulation results, which are different from Fig. 6. Fig. 6 shows the actual application test results. The execution time analysis of the existing CPS system and the proposed DTBM-IoT system are depicted by application simulation in Figs. 6a and 6b. The simulation analysis of the existing and proposed DTBM-IoT system is computed using the same software condition and simulation parameters. The respective time taken by the system is computed concerning the number of IoT devices. As the IoT devices increase, the system outcome increases using accurate results, and the execution time increases. The lesser number of IoT devices achieves a lower execution time.
Figure 6: (a) Execution time analysis of the existing CPS system by actual application test. (b) Execution time analysis of the proposed DTBM-IoT system by actual application test
Table 4 indicates the correlation analysis of the proposed DTBM-IoT system. The existing CPS system and the proposed DTBM-IoT system are evaluated under particular simulation test conditions. The number of IoT devices are varied from a smaller size to a higher size with a step size of 10 devices. The correlation between the actual physical space and the virtual space by the digital twin is considered and compared. The proposed DTBM-IoT system produces better correlation with the help of a deep neural network, and the mathematical model varies the outcomes.
The correlation analysis of the existing CPS system and proposed DTBM-IoT system are expressed in Figs. 7a and 7b. The simulation findings of the proposed DTBM-IoT system are evaluated using the given database in the simulation tool. The simulation outcomes are compared with each other. The correlation is computed as the ratio of the visualization results proportional to actual physical space. As the number of IoT devices increases, the proposed DTBM-IoT system’s accuracy increases, and the correlation results increase. These results are achieved by the multiple processing of the same data in the deep neural network. Comparison results between completed work and proposed work is shown in Table 5.
Figure 7: (a) Correlation analysis of the existing CPS system. (b) Correlation analysis of the proposed DTBM-IoT system
The proposed DTBM-IoT system is implemented, analyzed and the outcomes are compared with the existing CPS system in this section. The simulation outcomes such as accuracy, execution time, and correlation are computed, and the simulation findings show that the effectiveness of the proposed DTBM-IoT system over the existing CPS system.
5 Conclusions and Future Scope
Fault identification is experiencing a significant transition, moving away from rectifying failure after it happens and toward forecasting it when decay tendencies appear. More modern technologies are being introduced to make surveillance and care coordination more proactive, dependable, and cost-effective. A digital twin in braiding machinery based on IoT (DTBM-IoT) was presented in this research to improve fault detection by making it more clear, adaptable, and efficient.
DTBM-IoT abstractly and graphically mimics failure development and restoration. The significance is as follows. Firstly, it handles the trained data inadequately at the start of the production process, which also processed varied data dispersion in shifting operating conditions. Furthermore, the diagnostic servicing cycle extends from the production process throughout the product lifespan. As in the testing stages, the digital twin’s goal is to detect and rectify probable design flaws and construct a well-trained diagnostic model. The learned information from the simulations is then transmitted from the simulated space to the spatial context without training the generative model in the operational stage. The chance of unintended failure considerably decreases because of dual fault detection in online and offline space, making intelligent production more ecological, dependable, and economical.
Funding Statement: This research was supported by the Fujian Province Natural Science Foundation(Grant No. 2019J01711), Fujian Province Middle-aged Teachers Project (Grant No. JAT210670), Fujian Province Educational Reform Project (Grant No. FBJG2020316).
Conflicts of Interest: The authors declare that they have no conflicts of interest to report regarding the present study.
References
1. A. Fuller, Z. Fan, C. Day and C. Barlow, “Digital Twin: Enabling technologies, challenges and open research,” IEEE Access, vol. 8, pp. 108952–108971, 2020. [Google Scholar]
2. H. Mohapatra, B. K. Mohanta, M. R. Nikoo, M. Daneshmand and A. H. Gandomi, “MCDM based routing for IoT enabled smart water distribution network,” IEEE Internet of Things Journal, vol. 10, no. 5, pp. 4271–4280, 2022. [Google Scholar]
3. M. S. Ibrahim, J. J. Fan, W. K. C. Yung, A. Prisacaru, W. van Driel et al., “Machine learning and digital twin driven diagnostics and prognostics of light-emitting diodes,” Laser and Photonics Reviews, vol. 14, no. 12, pp. 2000254, 2020. [Google Scholar]
4. H. Huang, L. Yang, X. Wang, Y. Xu and Y. Lu, “Digital twin-driven online anomaly detection for an automation system based on edge intelligence,” Journal of Manufacturing Systems, vol. 59, pp. 138–150, 2021. [Google Scholar]
5. M. Zhang, F. Tao and A. Y. C. Nee, “Digital twin enhanced dynamic job-shop scheduling,” Journal of Manufacturing Systems, vol. 58, no. 58, pp. 146–156, 2021. [Google Scholar]
6. S. Venkatesan, K. Manickavasagam, N. Tengenkai and N. Vijayalakshmi, “Health monitoring and prognosis of electric vehicle motor using intelligent-digital twin,” IET Electric Power Applications, vol. 13, no. 9, pp. 1328–1335, 2019. [Google Scholar]
7. M. Liu, S. Fang, H. Dong and C. Xu, “Review of digital twin about concepts, technologies, and industrial applications,” Journal of Manufacturing Systems, vol. 58, no. September, pp. 346–361, 2021. [Google Scholar]
8. F. Tao, H. Zhang, A. Liu and A. Y. Nee, “Digital twin in the industry: State-of-the-art,” IEEE Transactions on Industrial Informatics, vol. 15, no. 4, pp. 2405–2415, 2018. [Google Scholar]
9. Y. He, J. Guo and X. Zheng, “From surveillance to digital twin: Challenges and recent advances of signal processing for the industrial internet of things,” IEEE Signal Processing Magazine, vol. 35, no. 5, pp. 120–129, 2018. [Google Scholar]
10. R. B. Roy, D. Mishra, S. K. Pal, T. Chakravarty, S. Panda et al., “Digital twin: Current scenario and a case study on a manufacturing process,” The International Journal of Advanced Manufacturing Technology, vol. 107, no. 9, pp. 3691–3714, 2020. [Google Scholar]
11. Y. Wang, F. Tao, M. Zhang, L. Wang and Y. Zuo, “Digital twin enhanced fault prediction for the autoclave with insufficient data,” Journal of Manufacturing Systems, vol. 60, no. 1, pp. 350–359, 2021. [Google Scholar]
12. A. Gaikwad, R. Yavari, M. Montazeri, K. Cole, L. K. Bian et al., “Toward the digital twin of additive manufacturing: Integrating thermal simulations, sensing, and analytics to detect process faults,” IISE Transactions, vol. 52, no. 11, pp. 1204–1217, 2020. [Google Scholar]
13. R. S. Kenett and J. Bortman, “The digital twin in Industry 4.0: A wide-angle perspective,” Quality and Reliability Engineering International, vol. 38, no. 3, pp. 1357–1366, 2022. [Google Scholar]
14. E. Negri, V. Pandhare, L. Cattaneo, J. Singh, M. Macchi et al., “Field-synchronized digital twin framework for production scheduling with uncertainty,” Journal of Intelligent Manufacturing, vol. 32, no. 4, pp. 1207–1228, 2021. [Google Scholar]
15. T. Kong, T. Hu, T. Zhou and Y. Ye, “Data construction method for the applications of workshop digital twin system,” Journal of Manufacturing Systems, vol. 58, no. 2, pp. 323–328, 2021. [Google Scholar]
16. M. J. Kaur, V. P. Mishra and P. Maheshwari, “The convergence of digital twin, IoT, and machine learning: Transforming data into action,” in Digital Twin Technologies and Smart Cities. Cham: Springer, pp. 3–17, 2020. [Google Scholar]
17. W. Luo, T. Hu, Y. Ye, C. Zhang and Y. Wei, “A hybrid predictive maintenance approach for CNC machine tool driven by digital twin,” Robotics and Computer-Integrated Manufacturing, vol. 65, no. 1, pp. 101974, 2020. [Google Scholar]
18. Z. Liu, W. Chen, C. Zhang, C. Yang and Q. Cheng, “Intelligent scheduling of a feature-process-machine tool super network based on digital twin workshop,” Journal of Manufacturing Systems, vol. 58, no. 4, pp. 157–167, 2021. [Google Scholar]
19. J. Wang, L. Ye, R. X. Gao, C. Li and L. Zhang, “Digital Twin for rotating machinery fault diagnosis in smart manufacturing,” International Journal of Production Research, vol. 57, no. 12, pp. 3920–3934, 2019. [Google Scholar]
20. F. Mostafa, L. Tao and W. Yu, “An effective architecture of digital twin system to support human decision making and AI-driven autonomy,” Concurrency and Computation: Practice and Experience, vol. 33, no. 19, pp. e6111, 2021. [Google Scholar]
21. A. Ladj, Z. Q. Wang, O. Meski, F. Belkadi, M. Ritou et al., “A knowledge-based Digital Shadow for the machining industry in a Digital Twin perspective,” Journal of Manufacturing Systems, vol. 58, no. 18, pp. 168–179, 2021. [Google Scholar]
22. E. B. Priyanka, S. Thangavel, X. Z. Gao and N. S. Sivakumar, “Digital twin for oil pipeline risk estimation using prognostic and machine learning techniques,” Journal of Industrial Information Integration, vol. 26, pp. 100272, 2021. [Google Scholar]
23. A. J. H. Redelinghuys, A. H. Basson and K. Kruger, “A six-layer architecture for the digital twin: A manufacturing case study implementation,” Journal of Intelligent Manufacturing, vol. 31, no. 6, pp. 1383–1402, 2020. [Google Scholar]
24. J. F. Liu, H. G. Zhou, X. J. Liu, G. Z. Tian, M. F. Wu et al., “Dynamic evaluation method of machining process planning based on digital twin,” IEEE Access, vol. 7, pp. 19312–19323, 2019. [Google Scholar]
25. F. Jiang, L. Ma, T. Broyd and K. Chen, “Digital twin and its implementations in the civil engineering sector,” Automation in Construction, vol. 130, no. 2, pp. 103838, 2021. [Google Scholar]
26. Q. L. Qi, F. Tao, T. L. Hu, N. Anwer, A. Liu et al., “Enabling technologies and tools for the digital twin,” Journal of Manufacturing Systems, vol. 58, no. 1, pp. 3–21, 2021. [Google Scholar]
Cite This Article
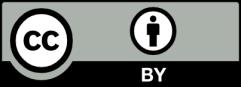