Open Access
ARTICLE
A Sensor Network Coverage Planning Based on Adjusted Single Candidate Optimizer
1 Fujian Provincial Key Laboratory of Big Data Mining and Applications, Fujian University of Technology, Fuzhou, 350118, China
2 University of Information Technology, Ho Chi Minh City, Vietnam
3 Vietnam National University, Ho Chi Minh City, 700000, Vietnam
* Corresponding Author: Thi-Kien Dao. Email:
(This article belongs to the Special Issue: Artificial Intelligence Algorithm for Industrial Operation Application)
Intelligent Automation & Soft Computing 2023, 37(3), 3213-3234. https://doi.org/10.32604/iasc.2023.041356
Received 19 April 2023; Accepted 17 June 2023; Issue published 11 September 2023
Abstract
Wireless sensor networks (WSNs) are widely used for various practical applications due to their simplicity and versatility. The quality of service in WSNs is greatly influenced by the coverage, which directly affects the monitoring capacity of the target region. However, low WSN coverage and uneven distribution of nodes in random deployments pose significant challenges. This study proposes an optimal node planning strategy for network coverage based on an adjusted single candidate optimizer (ASCO) to address these issues. The single candidate optimizer (SCO) is a metaheuristic algorithm with stable implementation procedures. However, it has limitations in avoiding local optimum traps in complex node coverage optimization scenarios. The ASCO overcomes these limitations by incorporating reverse learning and multi-direction strategies, resulting in updated equations. The performance of the ASCO algorithm is compared with other algorithms in the literature for optimal WSN node coverage. The results demonstrate that the ASCO algorithm offers efficient performance, rapid convergence, and expanded coverage capabilities. Notably, the ASCO achieves an archival coverage rate of 88%, while other approaches achieve coverage rates below or equal to 85% under the same conditions.Keywords
Cite This Article
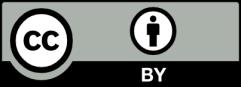