Open Access
ARTICLE
A Modeling Method for Predicting the Strength of Cemented Paste Backfill Based on a Combination of Aggregate Gradation Optimization and LSTM
1
School of Civil and Resource Engineering, University of Science and Technology Beijing, Beijing, 100083, China
2
Key Laboratory of Ministry of Education of China for Efficient Mining and Safety of Metal Mines, University of Science and
Technology Beijing, Beijing, 100083, China
* Corresponding Author: Bin Han. Email:
(This article belongs to the Special Issue: Circular Economy in the Development of Eco-Friendly Materials for Construction)
Journal of Renewable Materials 2022, 10(12), 3539-3558. https://doi.org/10.32604/jrm.2022.021845
Received 08 February 2022; Accepted 20 March 2022; Issue published 14 July 2022
Abstract
Cemented paste backfill (CPB) is a sustainable mining technology that is widely used in mines and helps to improve the mine environment. To investigate the relationship between aggregate grading and different affecting factors and the uniaxial compressive strength (UCS) of the cemented paste backfill (CPB), Talbol gradation theory and neural networks is used to evaluate aggregate gradation to determine the optimum aggregate ratio. The mixed aggregate ratio with the least amount of cement (waste stone content river sand content = 7:3) is obtained by using Talbol grading theory and pile compactness function and combined with experiments. In addition, the response surface method is used to design strength-specific ratio experiments. The UCS prediction model which uses the LSTM and considers the aggregates gradation have high accuracy. The root mean square error (RMSE) of the prediction results is 0.0914, the coefficient of determination (R2 ) is 0.9973 and the variance account for (VAF) is 99.73. Compared with back propagation neural network (BP-ANN), extreme learning machine (ELM) and radial basis function neural network (RBF-ANN), LSTM can effectively characterize the nonlinear relationship between UCS and individual affecting factors and predict UCS with high accuracy. The sensitivity analysis of different affecting factors on UCS shows that all 4 factors have significant effect on UCS and sensitivity is in the following ranking: cement content (0.9264) > slurry concentration (0.9179) > aggregate gradation (waste rock content) (0.9031) > curing time (0.9031).Graphic Abstract
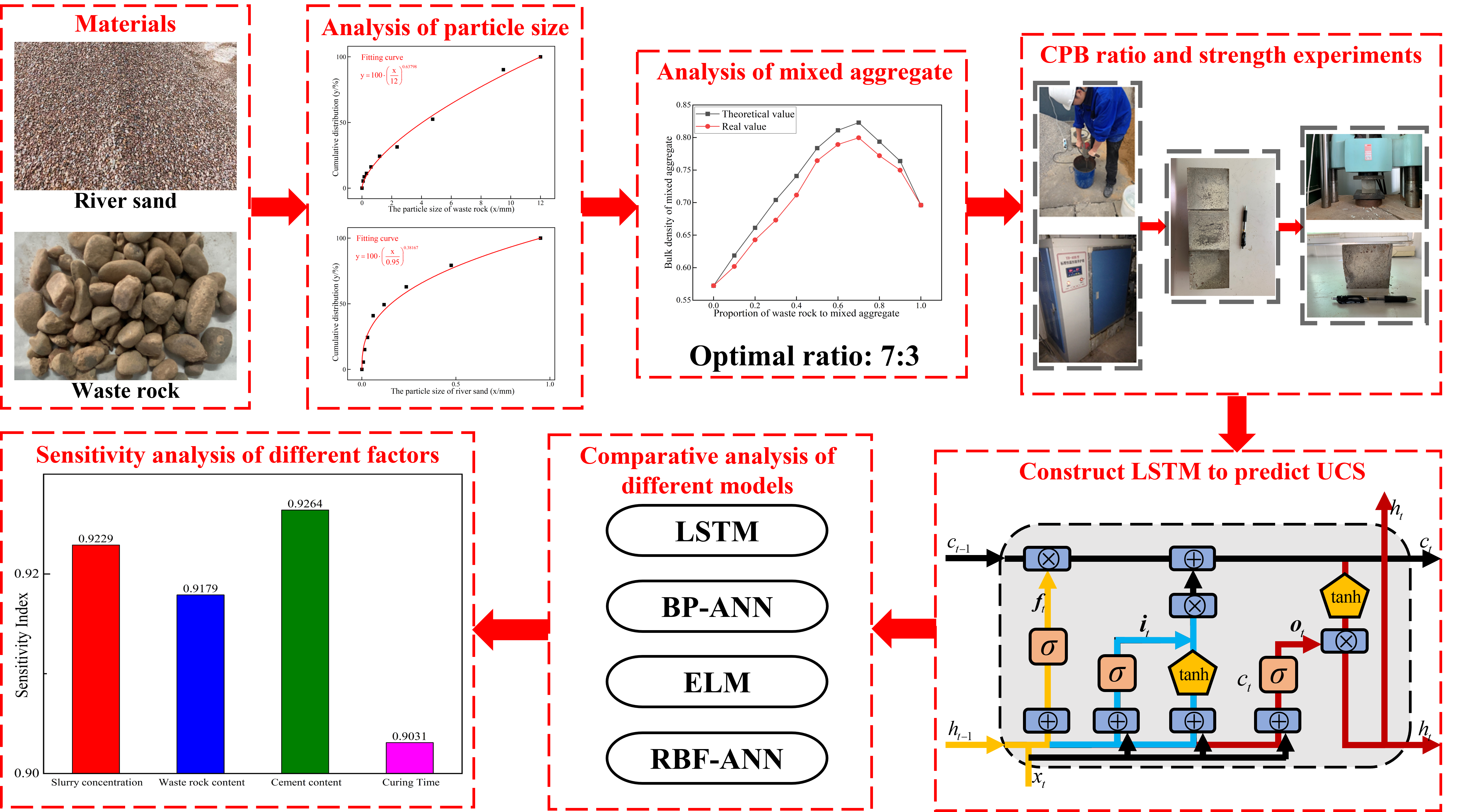
Keywords
Cite This Article
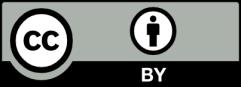
This work is licensed under a Creative Commons Attribution 4.0 International License , which permits unrestricted use, distribution, and reproduction in any medium, provided the original work is properly cited.