Open Access
ARTICLE
Comprehensive Analysis of the Expression and Clinical Significance of a Ferroptosis-Related Genome in Ovarian Serous Cystadenocarcinoma: A Study Based on TCGA Data
Department of Gynecology, The Fifth Affiliated Hospital of Sun Yat-sen University, Zhuhai, 519000, China
* Corresponding Author: Hua Yang. Email:
(This article belongs to the Special Issue: Ferroptosis in the Occurrence and Treatment of Cancer)
Oncologie 2022, 24(4), 835-863. https://doi.org/10.32604/oncologie.2022.026447
Received 06 September 2022; Accepted 17 November 2022; Issue published 31 December 2022
Abstract
Background: Epithelial ovarian cancer (EOC) is the deadliest malignancy among the gynecologic tumors, and ovarian serous cystadenocarcinoma (OV) is the dominant histological type. Ferroptosis is a novel iron-dependent, programmed form of cell death, and agents that trigger ferroptosis may constitute potential anti-cancer therapies. Materials and Methods: We herein extracted the genes that participate in the process of ferroptosis from the online FerrDb database to create a ferroptosis-related genome (FRG), and then comprehensively analyzed the relationship between the mRNA expression of each gene and the clinicopathologic features of The Cancer Genome Atlas (TCGA)-OV cohort. Results: We found that most of the FRG genes were differently expressed between OV and normal ovarian tissue and were significantly related to the prognosis of OV. In addition, gene ontology (GO) analysis revealed that the candidate genes of the FRG were primarily associated with responses to nutrient levels, oxidative stress, oxygen levels, and neuronal death. The Kyoto Encyclopedia of Genes and Genomes (KEGG) analysis indicated that candidate genes of the FRG were primarily associated with ferroptosis, hepatitis B, mitophagy, prostate cancer, and bladder cancer. The protein–protein interaction (PPI) network exhibited 132 nodes, 565 edges, an average node degree of 9.94, and an average local clustering coefficient of 0.539, indicating that the proteins were closely interrelated with one another and constitute a biological cluster. A comprehensive analysis of the top 5 genes involved in promoting and preventing ferroptosis revealed that these genes were differently expressed between OV and normal ovarian tissue, were significantly related to the prognosis of OV, reflected excellent diagnostic value, and were significantly correlated with immune cell infiltration in the tumor microenvironment (TME), polarization of macrophages, and with immune checkpoints. The protein levels of top 5 genes involved in promoting ferroptosis were differentially expressed between OV and normal ovarian tissue, and the top 5 genes involved in preventing ferroptosis were constitutively expressed in most of the normal tissues and tumors. Conclusions: We herein ascertained that the majority of the FRG was differentially expressed between OV and normal ovarian tissue, that the genes were significantly related to prognosis, and that the dysregulation of ferroptosis appeared to influence the development and progression of OV. The FRG showed an excellent diagnostic value for OV, and we postulate that it is significantly correlated with immune cell infiltration and immune checkpoints in the TME. We posit that targeting ferroptosis might comprise a novel anti-cancer therapy in OV.Keywords
Abbreviations
EOC | Epithelial ovarian cancer |
OV | Ovarian serous cystadenocarcinoma |
FRG | Ferroptosis-related genome |
TCGA | The cancer genome altas |
PFS | Progression-free survival |
OS | Overall survival |
STRING | Search tool for the retrieval of interaction genes/proteins |
PPI | Protein-protein interaction network |
HPA | Human protein altas |
TIMER | Tumor immune estimation resource |
ICKs | Immune checkpoints |
GO | Gene Ontology |
KEGG | Kyoto Encyclopedia of Genes and Genomes |
TME | Tumor microenvironment |
WHO | World Health Organization |
PARP | Poly (adenosine diphosphate-ribose) polymerase |
BRCA | Breast cancer gene |
HRD | Homologous recombination deficiency |
L-ROS | Lipid reactive oxygen species |
TFR | Transferrin receptor |
FPN | Ferroportin |
GEO | Gene expression omnibus |
EGA | European Genome-Phenome Archive |
EMBL | European Molecular Biology Laboratory |
GEPIA | The Gene Expression Profiling Interactive Analysis |
GTEx | Genotype-tissue expression |
PD-1 | Programmed cell death protein-1 |
PD-L1 | programmed death ligand 1 |
EOC is the deadliest disease among gynecological malignancies, and according to the World Health Organization (WHO), there were 295,400 new cases and 184,800 deaths of ovarian cancer worldwide in 2021 [1]. OV is the dominant histological type, and the standard treatment is still optimal cytoreduction (primarily debulking surgery or interval debulking surgery) combined with platinum-based chemotherapy. Most patients emerge with recurrent, chemoresistant, and ultimately terminal disease, and the median progression-free survival (mPFS) is only 12–18 months [2]. However, targeted therapy has progressed markedly in recent years. In the clinical trials ICON7 [3] and GOG0218 [4], the investigators evaluated the efficacy of combined bevacizumab as first-line chemotherapy and then maintained chemotherapy for EOC; however, these chemotherapeutic agents only extended PFS by 2.4 months and 3.8 months, respectively. Poly (adenosine diphosphate ribose) polymerase (PARP) inhibitors have significantly extended PFS and overall survival (OS) of women with EOC and who harbor the breast cancer gene (BRCA) mutations and homologous recombination deficiency (HRD). However, an epidemiological survey revealed that only 43% of patients carried BRCA mutations and/or HRD and that over half of patients did not benefit from PARP inhibitors [5]. While immunotherapeutic targeting of programmed cell death protein-1 (PD-1)/programmed death ligand 1 (PD-L1) shows limited benefit in EOC [6], there remains an urgent need to develop novel and efficient therapies to overcome the challenges inherent to existing therapies.
Ferroptosis is a novel iron-dependent, programmed form of cell death that is characterized by an overwhelming accumulation of cytotoxic lipid reactive oxygen species (L-ROS) and perturbations to the integrity and permeability of bio-membranes and that is significantly distinct from other known forms of cell death such as parthanatos, pyroptosis, apoptosis, and necroptosis [7]. Ferroptosis is involved in the progression of multiple diseases, including neurodegeneration [8], Parkinson’s disease, Alzheimer’s disease, acute renal failure [9], ischemia/reperfusion injury of the intestine and myocardial infarction [10], and cancers [11–15]. Recent studies revealed a number of genes that have been implicated in ferroptosis, including involvement in pathways of L-ROS metabolism, iron metabolism, and redox reactions. For example, glutathione peroxidase 4 (GPX4) is considered to be a critical regulator in preventing ferroptosis [16], while dysregulation of ferroptosis might influence the development and progression of cancers. Some clinical anti-tumor compounds such as sorafenib [17] and traditional Chinese medicines such as artesunate [18] and piperlongumine [19] manifested anti-cancer effects via the ferroptotic pathway. Due to its non-apoptotic properties, triggering ferroptosis may sensitize resistant cancer cells, and pioneering studies have reported that erastin sensitized resistant acute myeloid leukemia cells [20], glioma cells [21], and head and neck cancer cells [22] to re-administer chemotherapeutic intervention. Metabolic changes constitute a common feature in tumors, and high levels of intracellular iron have now been reported for a number of cancers, including glioblastoma [23], and breast [24] and prostate cancers [25]. Recent studies showed that iron metabolism was dramatically perturbed in OV, characterized by the up-regulation of the iron transporter transferrin receptor (TFR)1 and the iron-storage protein ferritin, and down-regulation of the iron efflux pump ferroportin (FPN) [26]. Such vulnerabilities might be able to be exploited therapeutically, and therefore, triggering ferroptosis is emerging as an attractive anti-cancer therapy in OV.
Emerging experimental studies have shown the potential effect of triggering ferroptosis in a variety of tumors such as colorectal cancer [27], breast cancer [28], lung cancer [29], thyroid cancer [30], bladder cancer [31], and glioma [32]. Due to its non-apoptotic nature, the use of anti-cancer therapy in triggering ferroptosis was expected to overcome the disadvantages of traditional chemotherapy and radiotherapy, and, intriguingly, it was found that chemotherapy-resistant cancer cells were still sensitive to ferroptosis inducers [33–35]. Thus, triggering ferroptosis has developed into an effective strategy for treating cancer cells resistant to traditional therapies. However, the effects of triggering ferroptosis in OV remain unexplored. Elucidating the molecular regulation of ferroptosis has undergone rapid progress, and investigators have identified numerous genes that participate in the process of ferroptosis, including those promoting and preventing ferroptosis. In addition to regulating ferroptosis, these genes form a complex regulatory network that is closely associated with metabolic and other cellular functions. Ferroptosis is also regulated by a variety of genes and signaling pathways, and these appear to interact with each other to collectively affect ferroptotic sensitivity. Since the functions and effects of single genes may be compensated by other genes, the value of a single gene in ferroptosis remains limited. Therefore, comprehensively analyzing the expression and clinical significance of a ferroptosis-related genome (FRG) in OV would facilitate the evaluation of susceptibility to ferroptosis and also assist in the development of therapeutic strategies that target ferroptosis.
In the present study, we compared the mRNA and protein levels of FRG genes between OV and normal ovarian tissue and evaluated the correlations between the FRG and clinical characteristics, survival prognosis, immune cell infiltration, macrophage polarization, and immune checkpoints in OV.
The TCGA database [36] (https://portal.gdc.cancer.gov) was initiated in 2006 by the National Cancer Institute and the National Human Genome Research Institute, USA. The database contained a variety of data from more than 20,000 samples of 33 types of cancer, including transcriptomic expression, genomic variation, methylation, and clinical pathologic information. As the largest cancer gene database, TCGA has become the first choice for cancer research due to its large sample size, diverse data types, and standardized data formats. The data from OV patients—along with the expression of mRNAs and matching clinical data—were obtained by the TCGA cancer browser.
2.2 Building a Ferroptosis-Related Genome
We extracted the genes that participate in the process of ferroptosis from the online FerrDb V2 beta database to create an FRG. FerrDb (http://www.zhounan.org/ferrdb/current/) [37] was the world’s first manually curated database for regulators and ferroptosis-disease associations. The data in FerrDb are free to download and use for academic and research purposes only and are routinely updated to support long-term service. V2 beta was released on 2022-05-24.
The Human Protein Atlas (https://www.proteinatlas.org/) [38] is based upon proteomics, transcriptomics, and biologic data, and it offers expression information on distinct human samples that include tissues, cells, and organs. This online database currently provides information on 44 normal tissues and 20 of the most typical cancers and allows consultation on the survival curves of tumor patients.
According to the median expression of target genes of the FRG, patients were divided into high-expression and low-expression categories. To investigate the relationships between the expression levels of target gene and clinical outcomes of OV, we adopted R statistical software (version 3.6.3) and the survival R package (version 3.2–10) to assess the correlation between the expression of a target gene and prognosis in OV, and the ggplot2 R package (version 3.3.3) was implemented to assess the diagnostic value of a target gene for OV. To compensate for the lack of normal TCGA samples, we used the online tool KM plotter (http://www.kmplot.com/analysis/) [39]. The KM plotter is a meta-analysis-based discovery and validation tool of survival biomarkers and data sources that include the Gene Expression Omnibus (GEO), European Genome-phenome Archive (EGA), and TCGA, and it is able to assess the correlation between the expression of 30 k genes (mRNAs, miRNAs, proteins) and survival in 21 tumor types.
2.5 Comprehensive Analysis of Protein–Protein Interactions
The STRING database (https://cn.string-db.org/) [40] was created by the European Molecular Biology Laboratory (EMBL) and draws functional associations between genes. After importing the FRG into the online STRING database, we obtained the PPI network information, and a confidence score >0.7 was considered to be significant. Cytoscape software was utilized to map and analyze protein-interaction relationships, and the Network Analyzer was applied to calculate node degree of the PPI. We expected the proteins in the central nodes to be key proteins that reflected important regulatory functions.
The TIMER database (http://cistrome.org/TIMER/) [41] is a public online database that comprises a comprehensive resource for systematical analysis of immune infiltrates across 32 diverse cancer types. The correlation between FRG expression and 24 types of immune cell infiltration (i.e., by macrophages, NK cells, cytotoxic cells, dendritic cells, and neutrophils) in the TME of OV was thus evaluated via the TIMER database. We additionally displayed the relationship between the expression of a target gene and M1/M2 macrophages.
The Gene Expression Profiling Interactive Analysis (GEPIA) (http://gepia.cancer-pku.cn/index.html) [42] is an online database that consists of 9736 tumors and 8587 normal samples from TCGA and Genotype-Tissue Expression (GTEx) data. It focuses on the analysis of the relevance of two RNA sequences. Using the GEPIA database, we investigated the relationship between a target gene and three important ICKs: PD-1, PD-L1, and CTLA-4. The x-axis is presented with the level of the target gene, and the y-axis is plotted with immune checkpoints.
2.8 GO/KEGG-Enrichment Analyses
For this study, we conducted correlation analysis of candidate genes in ovarian cancer using TCGA data and calculated the Pearson correlation coefficient. GO analysis was performed using enriched GO functions in the cluster Profiler R package (version 3.14.3), and we exploited KEGG analysis using the Enrich KEGG function of the cluster Profiler R package (version 3.14.3). |ES| > 1, P < 0.05, and FDR < 0.25 were considered to be statistically significant.
A total of 379 OV samples and 88 normal ovarian samples from the TCGA database were enrolled in our research, and the details of RNA expression profiles, clinical data, pathological data, and prognostic information were extracted for further analysis. In Table 1, we summarize patient clinical information that includes age at diagnosis, race, FIGO stage, histologic grade, primary therapeutic outcome, anatomical neoplasm subdivision, venous invasion, lymphatic invasion, residual tumors, tumor status, OS events, disease-specific survival (DSS) events, and platinum-free interval (PFI) events.
3.2 The Expression and Prognostic Value of the FRG in OV
We initially extracted the genes participating in the process of ferroptosis from the FerrDb V2 beta online database to create the FRG. FerrDb was the world’s first manually curated database for regulators and ferroptotic-disease associations. A total of 455 genes that regulate ferroptosis were enrolled in the FRG, including 226 genes involved in promoting ferroptosis, 193 genes involved in preventing ferroptosis, and 18 genes involved in the bidirectional regulation of ferroptosis (Fig. 1A). Regarding genes that promoted and showed bidirectional regulation of ferroptosis and compared with normal ovarian samples, 72 genes were more highly expressed, 134 genes showed attenuated expression, seven genes were not expressed, and the expression of 31 genes was unchanged (Fig. 1B). With respect to genes that prevented and exhibited bidirectional regulation of ferroptosis, and compared with normal ovarian samples, 92 genes showed higher expression, the expression of 99 genes was reduced, seven genes were not expressed, and 13 genes remained unchanged (Fig. 1C). Seventy-eight genes involved in promoting ferroptosis were differentially expressed between normal ovarian samples and OV, and these were significantly related to PFS and OS in women with OV (Fig. 1D). In addition, 63 genes were differentially expressed between normal ovarian samples and OV and significantly related to PFS and OS in women with OV (Fig. 1E). We thereby selected these 141 candidates for further analysis. Our results revealed that the majority of the FRG was differentially expressed between OV and normal ovarian tissues and that the genes were significantly related to the prognosis of OV. We postulate that dysregulation of ferroptosis therefore influences the development and progression of OV.
Figure 1: The expression and prognostic value of FRG in OV. (A) Venn diagram showed the ferroptosis related genome. (B) The expression level of promoting ferroptosis in OV. (C) The expression level of preventing ferroptosis in OV. (D) Venn diagram showed the genes differently expressed between normal ovarian samples and OV, meanwhile significantly related with PFS and OS of OV for promoting ferroptosis. (E) Venn diagram showed the genes differently expressed between normal ovarian samples and OV, meanwhile significantly related with PFS and OS of OV for preventing ferroptosis. (F) GO analysis of candidates in cellular component. (G) GO analysis of candidates in biological process. (H) GO analysis of candidates in molecular function. (I) KEGG analysis of candidates. (J) PPI network of candidates
3.3 Gene Functional-Enrichment Analysis of Candidates in the FRG
We selected 141 candidates in the FRG that were differentially expressed and significantly related to PFS and OS in women with OV and applied them to enrichment analysis. Functional enrichment and Gene Ontology (GO) [43] analyses revealed functional enrichment of candidates in the three main functional groups: cellular component, biological process, and molecular function. In the cellular component category, 141 candidates genes were mainly involved in autophagosome membrane, NADPH oxidase complex, autophagosome, mitochondrial outer membrane, and apical part of cell (Table 2, Fig. 1G). In the biological process category, 141 candidates were primarily involved in response to nutrient levels, oxidative stress, oxygen levels, and neuron death (Table 2, Fig. 1F). In the molecular function category, 141 candidates were principally involved in ubiquitin protein ligase binding, superoxide-generating NADPH oxidase activity, disordered domain-specific binding, DNA-binding transcription activator activity, and RNA polymerase II-specific (Table 2, Fig. 1H). KEGG analysis indicated that the candidate genes were primarily associated with ferroptosis, hepatitis B, mitophagy, prostate cancer, and bladder cancer (Table 2, Fig. 1I). The functional interaction between proteins reflected the interrelationships and molecular underpinnings of the FRG in tumor cells, and we used STRING, Cytoscape, and Network Analyzer to assess the PPI network of the 141 candidates in the FRG to determine their interactions in OV. The PPI network exhibited 132 nodes, 565 edges, an average node degree of 9.94, and an average local clustering coefficient of 0.539. This indicated that candidates in the FRG interacted closely among themselves, the proteins closely interrelated with one another, and they constituted a biological cluster (Fig. 1J). These results revealed that in addition to regulating ferroptosis, these genes formed a complex regulatory network that was closely correlated with metabolism and various cellular functions. Ferroptosis was also regulated by a variety of genes and signaling pathways, and these molecular indices interacted with one another to collectively affect the sensitivity of ferroptosis. As the functions and effects of a single gene might be compensated by others, the value of a single gene in ferroptosis is limited. Multi-target or pan-target therapies may therefore comprise more ideal anti-tumor strategies.
3.4 The Expression and Prognostic Value of the Top 5 Genes Involved in Promoting Ferroptosis in OV
FerrDb provided the relevant gene scores as evidence according to the number of original research studies where the genes were verified as to their relation with ferroptosis, with higher scores representing more supporting evidence. We extracted the top 5 scores of genes (ACSL4, ALOX15, HMOX1, IREB2, and TP53) that promoted ferroptosis from the online FerrDb V2 beta for comprehensive analysis, and the results revealed that the expression of ALOX15, HMOX1, and IREB2 was significantly lower in women with OV than in normal ovarian tissue, that the expression of TP53 was significantly higher in OV (Fig. 2A), that the expression of ALOX15 and IREB2 was significantly related to the stage of OV (Fig. 2B), and that patients with attenuated expression of ALOX15 manifested a better survival outcome in the TCGA cohort (Fig. 2C). Kaplan–Meier analysis revealed that patients with attenuated expression of ACSL4 and IREB2 manifested a longer PFS, but that those with augmented expression of HMOX1 and TP53 showed longer PFS with OV (Fig. 2D). Reduced expression of ACSL4 and HMOX1 was related to longer OS, but elevated expression of ALOX15 and TP53 was associated with longer OS in OV (Fig. 2E). The expression of HOMX1 and IREB2 generated an excellent diagnostic value for OV, with AUC values of 0.898 (CI, 0.859–0.938) and 0.895 (CI, 0.866–0.923), respectively (Fig. 2F).
Figure 2: The expression and prognostic value of top 5 genes of promoting ferroptosis in OV. (A) The expression level of top 5 genes of promoting ferroptosis in OV and ovary. (B) The relation between expression level of top 5 genes of promoting ferroptosis and stages of OV. (C) The relation between expression level of top 5 genes of promoting ferroptosis and OS of OV patients in the TCGA cohort.(D) The relation between expression level of top 5 genes of promoting ferroptosis and PFS of OV patients by Kaplan-Meier analysis. (E) The relation between expression level of top 5 genes of promoting ferroptosis and OS of OV patients by Kaplan-Meier analysis. (F) The diagnostic value of top 5 genes of promoting ferroptosis of OV
3.5 The Protein Levels of the Top 5 Genes Involved in Promoting Ferroptosis in the HPA Database
Proteins are the direct executor of biological functions, as mRNAs are required for protein translation to perform their functions. We therefore analyzed the protein levels of the top 5 genes involved in promoting ferroptosis in the HPA database and noted that ACSL4 protein was expressed in most tissues at a high level, including cerebral cortex, lung, hippocampus, colorectal cancer, and testicular cancer. However, ACLS4 expression was reduced in ovary and OV (Figs. 3A, 3F, 3G), while expression of ALOX15 protein was elevated in OV but lower in ovary (Figs. 3B, 3H, 3I). HMOX1 protein was notably expressed in most tissues, including lung, duodenum, small intestine, skin, spleen, renal cancer, and urothelial cancer. However, its expression was lower in ovary and OV (Figs. 3C, 3J, 3K). IREB2 protein was highly expressed in most tissues, including cerebellum, parathyroid gland, adrenal gland, and lung cancer, but less expressed in ovary and OV (Figs. 3D, 3L, 3M). TP53 protein expression was higher in OV than in ovary (Figs. 3E, 3N, 3O). These results revealed that the protein levels of the top 5 genes involved in promoting ferroptosis were differentially expressed between OV and normal ovarian tissue.
Figure 3: The protein level of top 5 genes of promoting ferroptosis in HPA database. (A) The protein level of ACSL4 in 44 normal human tissues and 17 forms of human cancer. (B) The protein level of ALOX15 in 44 normal human tissues and 17 forms of human cancer. (C) The protein level of HMOX1 in 44 normal human tissues and 17 forms of human cancer. (D) The protein level of IREB2 in 44 normal human tissues and 17 forms of human cancer. (E) The protein level of TP53 in 44 normal human tissues and 17 forms of human cancer. (F) The immunohistochemical images of ACSL4 in ovarian tissue. (G) The immunohistochemical images of ACSL4 in ovarian cancer. (H) The immunohistochemical images of ALOX15 in ovarian tissue. (I) The immunohistochemical images of ALOX15 in ovarian cancer. (J) The immunohistochemical images of HMOX1 in ovarian tissue. (K) The immunohistochemical images of HMOX1 in ovarian cancer. (L) The immunohistochemical images of IREB2 in ovarian tissue. (M) The immunohistochemical images of IREB2 in ovarian cancer. (N) The immunohistochemical images of TP53 in ovarian tissue. (O) The immunohistochemical images of TP53 in ovarian cancer
3.6 Correlations between Immune Cell Infiltration and the Top 5 Genes Involved in Promoting Ferroptosis in OV
The significance of the TME (in addition to the cancer cells themselves) has received much attention [44]. The TME is the environment relied upon by tumor cells for survival and development. It includes various cells and non-cellular components as well as the capillaries, lymphatic networks, and bio-molecules that infiltrate around the tumor. Immune cells are vital components in the TME and closely related to tumor progression, outcome, and treatment response [45]. The analysis of immune cells is therefore expected to uncover novel therapeutic targets that would improve the treatment of this deadly disease. As shown in Figs. 4A–4E, all of the top 5 genes (ACSL4, ALOX15, HMOX1, IREB2, and TP53) were significantly correlated with immune cell infiltration in the TME. Macrophages were the principal immune cells that we observed in the TME of ovarian cancer and were closely correlated with proliferation, invasion, metastasis, drug resistance, and immune response. Macrophages that infiltrate into the TME are called tumor-associated macrophages (TAMs) and can be divided into M1-TAMs (carrying anti-tumor activity) and M2-TAMs (with pro-tumor activity) according to their relevant activation pathways. TAMs constitute a “double-edged sword” in the development of tumors and are associated with differential polarization. M1-TAMs are activated by interferon-γ (IFN-γ) and lipopolysaccharide (LPS), primarily secrete a series of pro-inflammatory and immune-stimulating molecules such as interleukin-1 β (IL-1β) and tumor necrosis factor α (TNF-α), and can inhibit tumor progression. M2-TAMs are activated by IL-4, IL-13, and immune complexes and mainly secrete a variety of anti-inflammatory molecules such as interleukin-10 (IL-10) and tumor necrosis factor β (TNF-β), thus promoting tumor progression. As shown in Figs. 4F–4O, ACSL4 and HMOX1 were significantly positively correlated with M1 macrophage polarization, ALOX15 and IREB2 were significantly negatively correlated with M1 macrophage polarization, and ACSL4, ALOX15, and HMOX1 were significantly positively correlated with M2 polarization of macrophages. These results revealed that the top 5 genes involved in promoting ferroptosis were closely related to immune cell infiltration and polarization of macrophages in the TME of OV.
Figure 4: The correlation between immune cell infiltration and top 5 genes of promoting ferroptosis in OV. (A) The correlation between immune cell infiltration and ACSL4 in OV. (B) The correlation between immune cell infiltration and ALOX15 in OV. (C) The correlation between immune cell infiltration and HMOX1 in OV. (D) The correlation between immune cell infiltration and IREB2 in OV. (E) The correlation between immune cell infiltration and TP53 in OV. (F) The correlation between M1-macrophage infiltration and ACSL4 in OV. (G) The correlation between M1-macrophage infiltration and ALOX15 in OV. (H) The correlation between M1-macrophage infiltration and HMOX1 in OV. (I) The correlation between M1-macrophage infiltration and IREB2 in OV. (J) The correlation between M1-macrophage infiltration and TP53 in OV. (K) The correlation between M2-macrophage infiltration and ACSL4 in OV. (L) The correlation between M2-macrophage infiltration and ALOX15 in OV. (M) The correlation between M2-macrophage infiltration and HMOX1in OV. (N) The correlation between M2-macrophage infiltration and IREB2 in OV. (O) The correlation between M1-macrophage infiltration and TP53 in OV
3.7 Correlations between Immune Checkpoints and the Top 5 Genes Involved in Promoting Ferroptosis in OV
Immune-checkpoint inhibitors comprise a novel immunotherapy and operate by activating immune cells to achieve the purpose of anti-tumorigenicity. At present, immune-checkpoint inhibitors show excellent anti-tumor effects in lung cancer, lymphoma, melanoma, and bowel cancer. The expression levels of immune checkpoint-related genes are closely correlated with the treatment responses to immune-checkpoint inhibitors [46]. We therefore examined three important ICKs (PD-1, PD-L1, and CTAL-4), and as shown in Fig. 5, ACSL4 and HMOX1 were significantly positively correlated with PD-1, PD-L1, and CTLA-4, and IREB2 was significantly positively correlated with PD-L1. These results showed that the top 5 genes promoting ferroptosis were closely related to ICKs and might also be related to responses to immunotherapy in OV.
Figure 5: The correlation between immune checkpoints and top 5 genes of promoting ferroptosis in OV. (A) The correlation between CTLA-4 and ACSL4. (B) The correlation between CTLA-4 and ALOX15. (C) The correlation between CTLA-4 and HMOX1. (D) The correlation between CTLA-4 and IREB2. (E) The correlation between CTLA-4 and TP53. (F) The correlation between PD-1 and ACSL4. (G) The correlation between PD-1 and ALOX15. (H) The correlation between PD-1 and HMOX1. (I) The correlation between PD-1 and IREB2. (J) The correlation between PD-1 and TP53. (K) The correlation between PD-L1 and ACSL4. (L) The correlation between PD-L1 and ALOX15. (M) The correlation between PD-L1 and HMOX1. (N) The correlation between PD-L1 and IREB2. (O) The correlation between PD-L1 and TP53
3.8 The Expression and Prognostic Value of the Top 5 Genes Involved in Preventing Ferroptosis in OV
We extracted the top 5 genes (FTH1, GPX4, NFE2L2, SCD, and SLC7A11) involved in preventing ferroptosis from the online FerrDb V2 beta database for comprehensive analysis and demonstrated that the expression of NFE2L2 and SCD was significantly lower in OV than in the ovary, that the expression of FTH1, GPX4, and SLC7A11 was significantly higher in OV (Fig. 6A), that the expression of NFE2L2 was significantly related to the stage of OV (Fig. 6B), and that patients showing higher expression levels of SLC7A11 manifested a better survival outcome in the TCGA cohort (Fig. 6C). Kaplan–Meier analysis revealed that patients with attenuated expression of FTH1 and SCD revealed a longer PFS, but that elevated expression of NFE2L2 and SLC7A11 was associated with longer PFS in OV (Fig. 6D). Reduced expression of NFE2L2 and SLC7A11 was related to longer OS (Fig. 6E), and the expression of FTH1, GPX4, NFE2L2, and SLC7A11 showed excellent diagnostic value for OV. The AUC values were 0.870 (CI, 0.839–0.901), 0.877 (CI, 0.845–0.908), 0.926 (CI, 0.901–0.951), and 0.836 (CI, 0.789–0.882) (Fig. 6F).
Figure 6: The expression and prognostic value of top 5 genes of preventing ferroptosis in OV. (A) The expression level of top 5 genes of preventing ferroptosis in OV and ovary. (B) The relation between expression level of top 5 genes of preventing ferroptosis and stages of OV. (C) The relation between expression level of top 5 genes of preventing ferroptosis and OS of OV patients in the TCGA cohort. (D) The relation between expression level of top 5 genes of preventing ferroptosis and PFS of OV patients by Kaplan-Meier analysis. (E) The relation between expression level of top 5 genes of preventing ferroptosis and OS of OV patients by Kaplan-Meier analysis. (F) The diagnostic value of top 5 genes of preventing ferroptosis of OV
3.9 The Protein Levels of the Top 5 Genes Involved in Preventing Ferroptosis from the HPA Database
We also analyzed the protein levels of the top 5 genes involved in preventing ferroptosis from the HPA database and showed that FTH1 protein was highly expressed in the majority of the 44 normal human tissues, including cerebral cortex, caudate nucleus, endometrium, and ovary. In addition, FTH1 was highly expressed in most of the 17 forms of human cancer, including glioma, thyroid cancer, renal cancer, and OV (Figs. 7A, 7E, 7F), GPX4 protein showed elevated expression in most of the 44 normal human tissues, including cerebral cortex, testis, duodenum, and ovary, and GPX4 protein was expressed in a few tumors such as thyroid cancer, renal cancer, prostate cancer, and melanoma, but its expression was attenuated in OV (Figs. 7B, 7G, 7H). We observed augmented NFE2L2 protein expression in virtually all of the 44 normal human tissues, including cerebral cortex, hippocampus, thyroid gland, stomach, and ovary. We noted that NFE2L2 was expressed in almost all of the 17 forms of human cancer, including glioma, thyroid cancer, liver cancer, renal cancer, and OV (Figs. 7C, 7I, 7G). SCD protein was highly expressed in almost all 44 normal human tissues, including cerebral cortex, hippocampus, thyroid gland, stomach, and ovary, and in almost all 17 forms of human cancer, including glioma, thyroid cancer, liver cancer, renal cancer, and OV (Figs. 7D, 7K, 7L). SLC7A11 protein was not found in the HPA database. These results revealed that the protein levels encoded by the top 5 genes involved in preventing ferroptosis were constitutively expressed in the majority normal tissues and tumors.
Figure 7: The protein level of top 5 genes of preventing ferroptosis in HPA database. (A) The protein level of FTH1 in 44 normal human tissues and 17 forms of human cancer. (B) The protein level of GPX4 in 44 normal human tissues and 17 forms of human cancer. (C) The protein level of NFE2L2 in 44 normal human tissues and 17 forms of human cancer. (D) The protein level of SCD in 44 normal human tissues and 17 forms of human cancer. (E) The immunohistochemical images of FTH1 in ovarian tissue. (F) The immunohistochemical images of FTH1 in ovarian cancer. (G) The immunohistochemical images of GPX4 in ovarian tissue. (H) The immunohistochemical images of GPX4 in ovarian cancer. (I) The immunohistochemical images of NFE2L2 in ovarian tissue. (J) The immunohistochemical images of NFE2L2 in ovarian cancer. (K) The immunohistochemical images of SCD in ovarian tissue. (L) The immunohistochemical images of SCD in ovarian cancer
3.10 Correlations between Immune Cell Infiltration and the Top 5 Genes Involved in Preventing Ferroptosis in OV
As shown in Figs. 8A–8E, all of the top 5 genes (FTH1, GPX4, NFE2L2, SCD, and SLC7A11) were significantly correlated with immune cell infiltration in the TME of OV. We further analyzed the relationship between the top 5 genes and macrophage polarization. As shown in Figs. 8F–8O, FTH1, GPX, and NFE2L2 were significantly positively correlated with M1 polarization of macrophages, and NFE2L2 was significantly positively correlated with M2 polarization. These results showed that the top 5 genes involved in preventing ferroptosis were closely related to immune cell infiltration and macrophage polarization in the TME of OV.
Figure 8: The correlation between immune cell infiltration and top 5 genes of preventing ferroptosis in OV. (A) The correlation between immune cell infiltration and FTH1 in OV. (B) The correlation between immune cell infiltration and GPX4 in OV. (C) The correlation between immune cell infiltration and NFE2L2 in OV. (D) The correlation between immune cell infiltration and SCD in OV. (E) The correlation between immune cell infiltration and SLC7A11 in OV. (F) The correlationbetween M1-macrophage infiltration and FTH1 in OV. (G) The correlation between M2-macrophage infiltration and FTH1 in OV. (H) The correlation between M1-macrophage infiltration and GPX4 in OV. (I) The correlation between M2-macrophage infiltration and GPX4 in OV. (J) The correlation between M2-macrophage infiltration and NFE2L2 in OV. (K) The correlation between M1-macrophage infiltration and NFE2L2 in OV. (L) The correlation between M1-macrophage infiltration and SCD in OV. (M) The correlation between M2-macrophage infiltration and SCDin OV. (N) The correlation between M1-macrophage infiltration and SLC7A11 in OV. (O) The correlation between M2-macrophage infiltration and SLC7A11 in OV
3.11 Correlations between Immune Checkpoints and the Top 5 Genes Involved in Preventing Ferroptosis in OV
We ultimately analyzed the relationships between the top 5 genes involved in preventing ferroptosis and three important ICKs that included PD-1, PD-L1, and CTAL4. As shown in Fig. 9, FTH1 and SCD were significantly positively correlated with PD-1, PD-L1, and CTLA4; NFE2L2 and SLC7A11 were significantly positively correlated with PD-L1; and GPX4 was significantly negatively correlated with PD-L1. These results signified that the top 5 genes involved in preventing ferroptosis were closely associated with ICKs and that they might be related to responses of immune-checkpoint inhibitors in OV.
Figure 9: The correlation between immune checkpoints and top 5 genes of preventing ferroptosis in OV. (A) The correlation between CTLA4 and FTH1. (B) The correlation between CTLA4 and GPX4. (C) The correlation between CTLA4 and NFE2L2. (D) The correlation between CTLA4 and SCD. (E) The correlation between CTLA4 and SLC7A11. (F) The correlation between PD-1 and FTH1. (G) The correlation between PD-1 and GPX4. (H) The correlation between PD-1 and NFE2L2. (I) The correlation between PD-1 and SCD. (J) The correlation between PD-1 and SLC7A11. (K) The correlation between PD-L1 and FTH1. (L) The correlation between PD-L1 and GPX4. (M) The correlation between PD-L1 and NFE2L2. (N) The correlation between PD-L1 and SCD. (O) The correlation between PD-L1 and SLC7A1
Ferroptosis is a novel iron-dependent, programmed form of cell death that is characterized by an overwhelming accumulation of cytotoxic L-ROS, disturbances in the integrity and permeability of bio-membranes, and is significantly distinct from other known forms of cell death such as parthanatos, pyroptosis, apoptosis, and necroptosis [7]. Ferroptosis can be inhibited by key regulators of redox homeostasis such as iron chelators, free radical-trapping antioxidants, and glutathione peroxidase 4. The principal cytological changes related to ferroptosis are concentrated on mitochondria, including smaller size, increased membrane density, and loss of mitochondrial cristae [47]. Recent studies have depicted ferroptosis as related to the onset and development of a variety of tumors, and our results also showed that most of the FRG was differentially expressed between OV and normal ovary, suggesting that dysregulation of ferroptosis exists in the development of OV.
Due to their exaggerated metabolic characteristics, most tumors are in a high oxidative-stress state, and these tumors (such as hepatocellular carcinoma [48], osteosarcoma [49], prostate cancer [50], cervical cancer [51], and diffuse large B cell lymphoma [52]) are more vulnerable to ferroptosis. Investigators recently demonstrated that iron metabolism is dramatically perturbed in a number of cancers that include breast cancer [53], glioblastoma [54], prostate cancer [55], and OV [56], and are characterized by the upregulation of the iron importer TFR1 and iron-storage protein ferritin and the downregulation of the iron efflux pump FPN. Such an “iron addiction” phenotype is inherently vulnerable to ferroptosis, and this can be exploited therapeutically. Triggering ferroptosis greatly reduced the viability and colony formation of non-small cell lung cancer [57], thyroid cancer [58], bladder cancer [59], glioma [60], head and neck squamous cell carcinoma [61], OV [62], and colon cancer [63] in vitro. Due to its non-apoptotic nature, anti-cancer therapy that triggers ferroptosis is expected to overcome the disadvantages of traditional chemotherapy and radiotherapy. Thus, the induction of ferroptosis has emerged as a therapeutic strategy for cancer cells that are resistant to traditional therapies. However, the effects of triggering ferroptosis in OV are currently unexplored. Our comprehensive analysis of the expression and clinical significance of a ferroptosis-related genome in OV facilitated the development of therapeutic strategies targeting ferroptosis and assisted in the evaluation of susceptibility to ferroptosis, and our results revealed that most of the FRG was significantly related to the prognosis of OV, suggesting that dysregulation of ferroptosis may participate in the development and progression of OV. We posit that OV might also be a ferroptosis-sensitive tumor and postulate that triggering ferroptosis would thereby constitute a novel therapeutic strategy in overcoming treatment challenges in OV.
The execution of ferroptosis involves a complex chain of biochemical reactions and a number of genes that are involved in ferroptosis. According to the FerrDb V2 beta online database, we enrolled a total of 455 genes in the FRG, including 226 genes that promoted ferroptosis, 193 genes that prevented ferroptosis, and 18 genes involved in the bidirectional regulation of ferroptosis; these genes then formed a complex regulatory network that related closely to cellular metabolism and other cellular functions besides ferroptosis. Ferroptosis is also regulated by a variety of genes and signaling pathways, and these may interact with one another with the functions and effects of a single gene compensated by others, but since the value of single gene in ferroptosis is limited, we screened differentially expressed genes between the ovary and OV and analyzed their relationships with PFS and OS in OV, enrolling 141 candidates. Enrichment analyses suggested that these candidate genes were primarily involved in ubiquitin protein ligase binding, superoxide-generating NADPH oxidase activity, disordered domain-specific binding, DNA-binding transcription activator activity, and RNA polymerase II-specific. KEGG analysis indicated that the candidate genes were primarily associated with ferroptosis, hepatitis B, mitophagy, prostate cancer, and bladder cancer. The PPI network exhibited 132 nodes, 565 edges, an average node degree of 9.94, and an average local clustering coefficient of 0.539. This indicated that these candidate genes were biologically connected as a group and suggested that they were closely related to each other in co-regulating a variety of biological functions and biochemical metabolic pathways. They were also closely related to ferroptosis and cancer, and therefore a multi-target or pan-target therapy might constitute a more ideal anti-tumor strategy for ferroptosis-based therapy.
To comprehensively explore the role and significance of the FRG in OV, we extracted for comprehensive analysis the top 5 genes involved in promoting ferroptosis (ACSL4, ALOX15, HMOX1, IREB2, and TP53) and in preventing ferroptosis (FTH1, GPX4, NFE2L2, SCD, and SLC7A11) from the online FerrDb V2 beta database. Acyl-CoA synthetase long-chain family member 4 (ACSL4), cysteine-glutamate antiporter system (system Xc-), and glutathione peroxidase 4 (GPx4) are the three primary components that regulate ferroptosis [64]. ACSL4 [65] is a member of the long-chain family of acyl-CoA synthetase proteins and considered a vital regulator of ferroptosis, and recent studies have shown that ACSL4 promotes the formation of phytosterol esters esterified from arachidonic acid (AA) and adrenaline as primary substrates for peroxidation reactions of ROS. SLC7A11 [66] is the functional component of system Xc-that imports extracellular cystine for the synthesis of glutathione (GSH), and is a critical regulator of cellular redox homeostasis and ferroptosis. GPX4 [67] converts lipid hydroperoxides to lipid alcohols and prevents the accumulation of toxic L-ROS, and the inhibition of GPX4 function is sufficient to lead to the induction of ferroptosis. We applied bioinformatics technology to verify the correlation between the top 5 genes involved in promoting/preventing ferroptosis and the clinicopathological features and prognosis in multiple databases, including TCGA, GEO, GTEx, HPA, KM plotter, and TIMER. Our results confirmed that the mRNAs and proteins generated by the top 5 genes involved in promoting/preventing ferroptosis were differentially expressed between OV and normal ovarian tissue, were closely related to the prognosis of OV, and showed high diagnostic value in OV. This further confirmed that ferroptosis participates in the onset, development, and progression of OV.
Previous studies have focused on the biological behaviors and regulatory mechanisms underlying tumor cells themselves. However, workers in the field have demonstrated that the TME plays a key role in tumor progression, invasion, and metastasis. The TME is a specific biological microenvironment composed of infiltrating immune cells, stromal cells, blood vessels, extracellular matrix, and exosomes. The infiltrating immune cells are pivotal functional elements, and a plethora of studies have shown that the level of T cell immune infiltration [68–70] is related to the efficacy of immunotherapy; this is therefore the focus of current research in immunotherapeutics. Elevated concentrations of B cells and T cells are associated with an improved OS in many types of cancers [71–73], and NK cells [74–76] are important mediators of anti-tumor immunity. Macrophages in the TME are defined as tumor-associated macrophages (TAMs) and comprise a topic of intense scientific scrutiny in recent years. TAMs are also the largest group of infiltrating leukocytes in solid tumors [77–79], affecting the development and outcome of cancers at multiple levels. These actions include modulating the TME, affecting tumor invasion and metastasis, regulating angiogenesis, and inducing drug resistance, and they are closely associated with the prognosis of various types of cancer. Although the exact mechanism(s) governing the progression and recurrence of ovarian cancer is still unelucidated, extant studies suggest that augmented infiltration levels of TAMs accelerate the progression of ovarian cancer. We herein demonstrated that the top 5 genes involved in promoting/preventing ferroptosis were closely associated with immune cell infiltration with respect to TAMs, B cells, and T cells, and we suggest that they are related to the progression of OV.
TAMs can be divided into M1-TAMs (with anti-tumor activity) and M2-TAMs (with pro-tumor activity) according to their activation pathways. Macrophages comprise a “double-edged sword” in the development of tumors, and this is related to differential polarization. M1-TAMs mainly secrete a series of pro-inflammatory and immune-stimulating molecules and can inhibit tumor progression. M2-TAMs chiefly secrete a variety of anti-inflammatory molecules and might promote tumor progression. Recent studies have found that higher levels of M2-TAMs [80] and a higher M2/M1 ratio [81] were related to a worse prognosis for OV. We analyzed the relationships between the top 5 genes involved in promoting/preventing ferroptosis and macrophage polarization employing the TIMRE online database. Our results showed that FTH1, GPX4, NFE2L2, ACSL4, and HMOX1 were significantly positively correlated with M1 polarization, that ALOX15 and IREB2 were significantly negatively correlated with M1 polarization, and that NFE2L2, ACSL4, ALOX15, and HMOX1 were significantly positively correlated with M2 macrophage polarization. These results showed that the top 5 genes involved in promoting/preventing ferroptosis were closely correlated with macrophage polarization in the TME and might be related to the prognosis of OV.
Locally immunosuppressed microenvironments constitute a common phenomenon in solid tumors and are usually mediated by CTLA-4 and PD-1/PD-L1 signaling. CTLA-4 [82] regulates T cell activation within secondary lymphoid organs, is expressed on regulatory T cells, and serves as a negative immune regulator. Programmed death protein-1 (PD-1) [83], a cell surface molecule expressed on activated T and B cells, interacts with the programmed death ligand 1 (PD-L1) [84], is present on leukocytes, non-hematopoietic cells, non-lymphoid tissues, and tumor cells via over-expression of PD-L1, and acquires the ability to activate PD-1 signaling in tumor-infiltrating T cells—all of which result in a locally immunosuppressed microenvironment. Immunotherapy has in recent years significantly improved the prognosis of several malignancies, including melanoma and lung and urogenital cancers, and an increasing number of exploratory trials involving ICKs in heavily treated recurrent OV (even in newly diagnosed settings) [85–87] have revealed that the expression levels of immune checkpoint-related genes were related to treatment responses to immune-checkpoint inhibitors. We therefore analyzed the correlation between the top 5 genes involved in promoting/preventing ferroptosis and immune-checkpoint-related genes and demonstrated that ACSL4, HMOX1, FTH1, and SCD were significantly positively correlated with PD-1, PD-L1, and CTLA4, that IREB2, NFE2L2, and SLC7A11 were significantly positively correlated with PD-L1, and that GPX4 was significantly negatively correlated with PD-L1. These results revealed that the top 5 genes involved in promoting/preventing ferroptosis were closely related to ICKs, and we posit that they are related to the responses of immune-checkpoint inhibitors in OV.
Overall, the present study revealed that most of the FRG genes were differently expressed between OV and normal ovarian tissues and that they were significantly associated with the prognosis of OV. However, there were some limitations to this study. First, individual variations in patients might influence ferroptotic sensitivity and the clinical value of the FRG in OV, and we herein only utilized TCGA and other public databases. We expect that additional biological evidence is needed to establish whether our FRG can be applied to clinical patients. Second, although we validated the expression and prognostic value of the FRG in TCGA and other public databases to a degree, we did not consider other significant genes and clinicopathological characteristics. Third, we only possessed a limited understanding of the signaling pathways involved in ferroptosis, and the specific molecular mechanisms underlying the FRG in OV—as well as their interactions with metabolism and cell death—remain unknown. In addition, the roles of the uncovered genes must be investigated both in vivo and in vitro in the future.
Most FRG genes were differently expressed between OV and normal ovarian tissue at the mRNA and protein levels and were significantly related to OV prognosis. We hypothesize that dysregulation of ferroptosis might influence the development and progression of OV. The FRG exhibited excellent diagnostic value with respect to OV and may be significantly correlated with immune cell infiltration and immune checkpoints in the TME. We posit that targeting ferroptosis might therefore constitute a novel anti-cancer therapy in OV.
Acknowledgement: The results shown here are wholly or partially based upon data generated by the TCGA Research Network at http://cancergenome.nih.gov/. The author thanks the library personnel of The Fifth Affiliated Hospital of Sun Yat-sen University for their assistance with literature searches. We also thank LetPub (www.letpub.com) for its linguistic assistance during the preparation of this manuscript.
Author’s Contributions: HY wrote, discussed, and reviewed the manuscript, and designed and created the figures.
Ethics Approval and Consent to Participate: Approval was obtained from the Institutional Review Board (IRB) of The Fifth Affiliated Hospital of Sun Yat-sen University for the publication of this literature review.
Availability of Data and Materials: All data generated or analyzed during this study were included in this published article.
Consent for Publication: Informed written consent was provided by the author.
Funding Statement: The author received no specific funding for this study.
Conflicts of Interest: The author declares that they have no conflicts of interest to report regarding the present study.
References
- Siegel, R. L., Miller, K. D., Fuchs, H. E., & Jemal, A. (2021). Cancer statistics. CA: A Cancer Journal for Clinicians, 71(1), 7-33. [Google Scholar] [CrossRef]
- Markham, M. J., Wachter, K., Agarwal, N., Bertagnolli, M. M., & Chang, S. M. (2020). Clinical cancer advances 2020: Annual report on progress against cancer from the American society of clinical oncology. Journal of Clinical Oncology, 38(10), 1081. [Google Scholar] [CrossRef]
- Oza, A. M., Cook, A. D., Pfisterer, J., Embleton, A., & Ledermann, J. A. (2015). Standard chemotherapy with or without bevacizumab for women with newly diagnosed ovarian cancer (ICON7): Overall survival results of a phase 3 randomised trial. Lancet Oncology, 16(8), 928-936. [Google Scholar] [CrossRef]
- Duska, L. R., Java, J. J., Cohn, D. E., & Burger, R. A. (2015). Risk factors for readmission in patients with ovarian, fallopian tube, and primary peritoneal carcinoma who are receiving front-line chemotherapy on a clinical trial (GOG 218): An NRG oncology/gynecologic oncology group study (ADS-1236). Gynecologic Oncology, 139(2), 221-227. [Google Scholar] [CrossRef]
- Konstantinopoulos, P. A., Ceccaldi, R., Shapiro, G. I., & D’Andrea, A. D. (2015). Homologous recombination deficiency: Exploiting the fundamental vulnerability of ovarian cancer. Cancer Discovery, 5, 1137. [Google Scholar] [CrossRef]
- Martin de la Fuente, L., Westbom-Fremer, S., Arildsen, N. S., Hartman, L., & Malander, S. (2020). PD-1/PD-L1 expression and tumor-infiltrating lymphocytes are prognostically favorable in advanced high-grade serous ovarian carcinoma. Virchows Archiv, 477(1), 83-91. [Google Scholar] [CrossRef]
- Stockwell, B. R., Friedmann Angeli, J. P., Bayir, H., Bush, A. I., & Conrad, M. (2017). Ferroptosis: A regulated cell death nexus linking metabolism, redox biology, and disease. Cell, 171(2), 273-285. [Google Scholar] [CrossRef]
- Abdalkader, M., Lampinen, R., Kanninen, K. M., Malm, T. M., & Liddell, J. R. (2018). Targeting Nrf2 to suppress ferroptosis and mitochondrial dysfunction in neurodegeneration. Frontiers in Neuroscience, 10(12), 466. [Google Scholar] [CrossRef]
- Müller, T., Dewitz, C., Schmitz, J., Schröder, A. S., & Bräsen, J. H. (2017). Necroptosis and ferroptosis are alternative cell death pathways that operate in acute kidney failure. Cellular and Molecular Life Sciences, 74(19), 3631-3645. [Google Scholar] [CrossRef]
- Li, X., Ma, N., Xu, J., Zhang, Y., & Yang, P. (2021). Targeting ferroptosis: Pathological mechanism and treatment of ischemia-reperfusion injury. Oxidative Medicine and Cellular Longevity, 2021, 1587922. [Google Scholar] [CrossRef]
- Guo, J., Xu, B., Han, Q., Zhou, H., & Xia, Y. (2018). Ferroptosis: A novel anti-tumor action for cisplatin. Cancer Research and Treatment, 50(2), 445-460. [Google Scholar] [CrossRef]
- Ma, S., Dielschneider, R. F., Henson, E. S., Xiao, W., & Choquette, T. R. (2017). Ferroptosis and autophagy induced cell death occur independently after siramesine and lapatinib treatment in breast cancer cells. PLoS One, 12(8), e0182921. [Google Scholar] [CrossRef]
- Yamaguchi, Y., Kasukabe, T., & Kumakura, S. (2018). Piperlongumine rapidly induces the death of human pancreatic cancer cells mainly through the induction of ferroptosis. International Journal of Oncology, 52(3), 1011-1022. [Google Scholar] [CrossRef]
- Chen, L., Li, X., Liu, L., Yu, B., & Xue, Y. (2015). Erastin sensitizes glioblastoma cells to temozolomide by restraining xCT and cystathionine-γ-lyase function. Oncology Reports, 33(3), 1465. [Google Scholar] [CrossRef]
- Roh, J. L., Kim, E. H., Jang, H., & Shin, D. (2017). Aspirin plus sorafenib potentiates cisplatin cytotoxicity in resistant head and neck cancer cells through xCT inhibition. Free Radical Biology and Medicine, 104, 1-9. [Google Scholar] [CrossRef]
- Ursini, F., & Maiorino, M. (2020). Lipid peroxidation and ferroptosis: The role of GSH and GPx4. Free Radical Biology and Medicine, 152, 175-185. [Google Scholar] [CrossRef]
- Sun, J., Zhou, C., Zhao, Y., Zhang, X., & Chen, W. (2021). Quiescin sulfhydryl oxidase 1 promotes sorafenib-induced ferroptosis in hepatocellular carcinoma by driving EGFR endosomal trafficking and inhibiting Nrf2 activation. Redox Biology, 41, 101942. [Google Scholar] [CrossRef]
- Li, Z. J., Dai, H. Q., Huang, X. W., Feng, J., & Deng, J. H. (2021). Artesunate synergizes with sorafenib to induce ferroptosis in hepatocellular carcinoma. Acta Pharmacologica Sinica, 42(2), 301-310. [Google Scholar] [CrossRef]
- Yang, Y., Sun, S., Xu, W., Zhang, Y., & Yang, R. (2022). Piperlongumine inhibits thioredoxin reductase 1 by targeting selenocysteine residues and sensitizes cancer cells to erastin. Antioxidants, 11(4), 710. [Google Scholar] [CrossRef]
- Cheng, L., Jin, X., Lu, W. Y., Sun, R., & Cao, Y. Q. (2021). Effect and involved mechanism of RSL3-induced ferroptosis in acute leukemia cells MOLM13 and drug-resistant cell lines. Zhongguo Shi Yan Xue Ye Xue Za Zhi, 29(4), 1109-1118. [Google Scholar]
- Wang, Z., Ding, Y., Wang, X., Lu, S., & Wang, C. (2018). Pseudolaric acid B triggers ferroptosis in glioma cells via activation of Nox4 and inhibition of xCT. Cancer Letter, 428, 21-33. [Google Scholar] [CrossRef]
- Lee, J., You, J. H., Shin, D., & Roh, J. L. (2020). Inhibition of glutaredoxin 5 predisposes cisplatin-resistant head and neck cancer cells to ferroptosis. Theranostics, 10(17), 7775-7786. [Google Scholar] [CrossRef]
- Thompson, H. J., Neil, E. S., & McGinley, J. N. (2021). Pre-clinical insights into the iron and breast cancer hypothesis. Biomedicines, 9(11), 1652. [Google Scholar] [CrossRef]
- Vela, D. (2018). Iron metabolism in prostate cancer; from basic science to new therapeutic strategies. Frontiers in Oncology, 7(8), 547. [Google Scholar] [CrossRef]
- Park, K. J., Kim, J., Testoff, T., Adams, J., & Poklar, M. (2019). Quantitative characterization of the regulation of iron metabolism in glioblastoma stem-like cells using magnetophoresis. Biotechnology and Bioengineering, 116(7), 1644-1655. [Google Scholar] [CrossRef]
- Rockfield, S., Raffel, J., Mehta, R., Rehman, N., & Nanjundan, M. (2017). Iron overload and altered iron metabolism in ovarian cancer. Biological Chemistry, 398(9), 995-1007. [Google Scholar] [CrossRef]
- Shen, L. D., Qi, W. H., Bai, J. J., Zuo, C. Y., & Bai, D. L. (2021). Resibufogenin inhibited colorectal cancer cell growth and tumorigenesis through triggering ferroptosis and ROS production mediated by GPX4 inactivation. Anatomical Record-Advances in Integrative Anatomy and Evolutionary Biology, 304(2), 313-322. [Google Scholar] [CrossRef]
- Du, X., Zhang, J., Liu, L., Xu, B., & Han, H. (2022). A novel anticancer property of lycium barbarum polysaccharide in triggering ferroptosis of breast cancer cells. Journal of Zhejiang University Science B, 23(4), 286-299. [Google Scholar] [CrossRef]
- Freire Boullosa, L., van Loenhout, J., Flieswasser, T., de Waele, J., & Hermans, C. (2021). Auranofin reveals therapeutic anticancer potential by triggering distinct molecular cell death mechanisms and innate immunity in mutant p53 non-small cell lung cancer. Redox Biology, 42, 101949. [Google Scholar] [CrossRef]
- Wang, X., Xu, S., Zhang, L., Cheng, X., & Yu, H. (2021). Vitamin C induces ferroptosis in anaplastic thyroid cancer cells by ferritinophagy activation. Biochemical and Biophysical Research Communications, 551, 46-53. [Google Scholar] [CrossRef]
- Sun, Y., Berleth, N., Wu, W., Schlütermann, D., & Deitersen, J. (2021). Fin56-induced ferroptosis is supported by autophagy-mediated GPX4 degradation and functions synergistically with mTOR inhibition to kill bladder cancer cells. Cell Death Disease, 12(11), 1028. [Google Scholar] [CrossRef]
- Lu, S., Wang, X. Z., He, C., Wang, L., & Liang, S. P. (2021). ATF3 contributes to brucine-triggered glioma cell ferroptosis via promotion of hydrogen peroxide and iron. Acta Pharmacologica Sinica, 42(10), 1690-1702. [Google Scholar] [CrossRef]
- Huang, R., Chen, H., Liang, J., Li, Y., & Yang, J. (2021). Dual role of reactive oxygen species and their application in cancer therapy. Journal of Cancer, 12(18), 5543-5561. [Google Scholar] [CrossRef]
- Gao, M., Deng, J., Liu, F., Fan, A., & Wang, Y. (2019). Triggered ferroptotic polymer micelles for reversing multidrug resistance to chemotherapy. Biomaterials, 223, 119486. [Google Scholar] [CrossRef]
- Guan, D., Li, C., Li, Y., Li, Y., & Wang, G. (2021). The DpdtbA induced EMT inhibition in gastric cancer cell lines was through ferritinophagy-mediated activation of p53 and PHD2/hif-1α pathway. Journal of Inorganic Biochemistry, 218, 111413. [Google Scholar] [CrossRef]
- Blum, A., Wang, P., & Zenklusen, J. C. (2018). SnapShot: TCGA-analyzed tumors. Cell, 173(2), 530. [Google Scholar] [CrossRef]
- Zhou, N., & Bao, J. (2020). FerrDb: A manually curated resource for regulators and markers of ferroptosis and ferroptosis-disease associations. Database, 2020, baaa021. [Google Scholar] [CrossRef]
- Uhlen, M., Zhang, C., Lee, S., Sjöstedt, E., & Fagerberg, L. (2017). A pathology atlas of the human cancer transcriptome. Science, 357(6352), eaan2507. [Google Scholar] [CrossRef]
- Lanczky, A., & Gyorffy, B. (2021). Web-based survival analysis tool tailored for medical research (KMplot): Development and implementation. Journal of Medical Internet Research, 23(7), e27633. [Google Scholar] [CrossRef]
- Szklarczyk, D., Gable, A. L., Nastou, K. C., Lyon, D., & Kirsch, R. (2021). The STRING database in 2021: Customizable protein-protein networks, and functional characterization of user-uploaded gene/measurement sets. Nucleic Acids Research, 49(D1), D605-D612. [Google Scholar] [CrossRef]
- Li, T., Fan, J., Wang, B., Traugh, N., & Chen, Q. (2017). TIMER: A web server for comprehensive analysis of tumor-infiltrating immune cells. Cancer Research, 77(21), e108-e110. [Google Scholar] [CrossRef]
- Li, C., Tang, Z., Zhang, W., Ye, Z., & Liu, F. (2021). GEPIA2021: Integrating multiple deconvolution-based analysis into GEPIA. Nucleic Acids Research, 49(W1), W242-W246. [Google Scholar] [CrossRef]
- Zhu, F., Li, J., Liu, J., & Min, W. (2021). Network-based cancer genomic data integration for pattern discovery. BMC Genomic Data, 22(Suppl 1), 54. [Google Scholar] [CrossRef]
- Bejarano, L., Jordāo, M. J. C., & Joyce, J. A. (2021). Therapeutic targeting of the tumor microenvironment. Cancer Discovery, 11(4), 933-959. [Google Scholar] [CrossRef]
- Roma-Rodrigues, C., Mendes, R., Baptista, P. V., & Fernandes, A. R. (2019). Targeting tumor microenvironment for cancer therapy. International Journal of Molecular Sciences, 20(4), 840. [Google Scholar] [CrossRef]
- Darvin, P., Toor, S. M., Sasidharan Nair, V., & Elkord, E. (2018). Immune checkpoint inhibitors: Recent progress and potential biomarkers. Experimental and Molecular Medicine, 50(12), 1-11. [Google Scholar] [CrossRef]
- Jiang, X., Stockwell, B. R., & Conrad, M. (2021). Ferroptosis: Mechanisms, biology and role in disease. Nature Reviews Molecular Cell Biology, 22(4), 266-282. [Google Scholar] [CrossRef]
- Chang, W. T., Bow, Y. D., Fu, P. J., Li, C. Y., & Wu, C. Y. (2021). A marine terpenoid, heteronemin, induces both the apoptosis and ferroptosis of hepatocellular carcinoma cells and involves the ROS and MAPK pathways. Oxidative Medicine and Cellular Longevity, 2021, 7689045. [Google Scholar] [CrossRef]
- Liu, Q., & Wang, K. (2019). The induction of ferroptosis by impairing STAT3/Nrf2/GPx4 signaling enhances the sensitivity of osteosarcoma cells to cisplatin. Cell Biology International, 43(11), 1245-1256. [Google Scholar] [CrossRef]
- Ghoochani, A., Hsu, E. C., Aslan, M., Rice, M. A., & Nguyen, H. M. (2019). Ferroptosis inducers are a novel therapeutic approach for advanced prostate cancer. Cancer Research, 81(6), 1583-1594. [Google Scholar] [CrossRef]
- Xiaofei, J., Mingqing, S., Miao, S., Yizhen, Y., & Shuang, Z. (2012). Oleanolic acid inhibits cervical cancer hela cell proliferation through modulation of the ACSL4 ferroptosis signaling pathway. Biochemical and Biophysical Research Communications, 545, 81-88. [Google Scholar] [CrossRef]
- Hong, Y., Ren, T., Wang, X., Liu, X., & Fei, Y. (2022). APR-246 triggers ferritinophagy and ferroptosis of diffuse large B-cell lymphoma cells with distinct TP53 mutations. Leukemia, 36(9), 2269-2280. [Google Scholar] [CrossRef]
- Von Holle, A., O’Brien, K. M., Sandler, D. P., Janicek, R., & Weinberg, C. R. (2021). Association between serum iron biomarkers and breast cancer. Cancer Epidemiology. Biomarkers and Prevention, 30(2), 422-425. [Google Scholar] [CrossRef]
- Chitambar, C. R., Al-Gizawiy, M. M., Alhajala, H. S., Pechman, K. R., & Wereley, J. P. (2018). Gallium maltolate disrupts tumor iron metabolism and retards the growth of glioblastoma by inhibiting mitochondrial function and ribonucleotide reductase. Molecular Cancer Therapeutics, 17(6), 1240-1250. [Google Scholar] [CrossRef]
- Wang, F., Liu, A., Bai, R., Zhang, B., & Jin, Y. (2017). Hepcidin and iron metabolism in the pathogenesis of prostate cancer. Journal of BUON, 22(5), 1328-1332. [Google Scholar]
- Bajbouj, K., Shafarin, J., & Hamad, M. (2019). Estrogen-dependent disruption of intracellular iron metabolism augments the cytotoxic effects of doxorubicin in select breast and ovarian cancer cells. Cancer Management and Research, 11, 4655-4668. [Google Scholar] [CrossRef]
- Gai, C., Yu, M., Li, Z., Wang, Y., & Ding, D. (2020). Acetaminophen sensitizing erastin-induced ferroptosis via modulation of Nrf2/heme oxygenase-1 signaling pathway in non-small-cell lung cancer. Journal of Cellular Physiology, 235(4), 3329-3339. [Google Scholar] [CrossRef]
- Wang, L., Zhang, Y., Yang, J., Liu, L., & Yao, B. (2021). The knockdown of ETV4 inhibits the papillary thyroid cancer development by promoting ferroptosis upon SLC7A11 downregulation. DNA and Cell Biology, 40(9), 1211-1221. [Google Scholar] [CrossRef]
- Kong, N., Chen, X., Feng, J., Duan, T., & Liu, S. (2021). Baicalin induces ferroptosis in bladder cancer cells by downregulating FTH1. Acta Pharmaceutica Sinica B, 11(12), 4045-4054. [Google Scholar] [CrossRef]
- Zhang, Y., Kong, Y., Ma, Y., Ni, S., & Wikerholmen, T. (2021). Loss of COPZ1 induces NCOA4 mediated autophagy and ferroptosis in glioblastoma cell lines. Oncogene, 40(8), 1425-1439. [Google Scholar] [CrossRef]
- Lu, T., Zhang, Z., Pan, X., Zhang, J., & Wang, X. (2022). Caveolin-1 promotes cancer progression via inhibiting ferroptosis in head and neck squamous cell carcinoma. Journal of Oral Pathology & Medicine, 51(1), 52-62. [Google Scholar] [CrossRef]
- Cheng, Q., Bao, L., Li, M., Chang, K., & Yi, X. (2021). Erastin synergizes with cisplatin via ferroptosis to inhibit ovarian cancer growth and . Journal of Obstetrics and Gynaecology Research, 47(7), 2481-2491. [Google Scholar] [CrossRef]
- Ye, S., Xu, M., Zhu, T., Chen, J., & Shi, S. (2021). Cytoglobin promotes sensitivity to ferroptosis by regulating p53-YAP1 axis in colon cancer cells. Journal of Cellular and Molecular Medicine, 25(7), 3300-3311. [Google Scholar] [CrossRef]
- Chen, X., Li, J., Kang, R., Klionsky, D. J., & Tang, D. (2021). Ferroptosis: Machinery and regulation. Autophagy, 17(9), 2054-2081. [Google Scholar] [CrossRef]
- Kuwata, H., & Hara, S. (2019). Role of acyl-CoA synthetase ACSL4 in arachidonic acid metabolism. Prostaglandins other Lipid Mediat, 144, 106363. [Google Scholar] [CrossRef]
- Koppula, P., Zhuang, L., & Gan, B. (2021). Cystine transporter SLC7A11/xCT in cancer: Ferroptosis, nutrient dependency, and cancer therapy. Protein Cell, 12(8), 599-620. [Google Scholar] [CrossRef]
- Jia, M., Qin, D., Zhao, C., Chai, L., & Yu, Z. (2020). Redox homeostasis maintained by GPX4 facilitates STING activation. Nature Immunology, 21(7), 727-735. [Google Scholar] [CrossRef]
- Farhood, B., Najafi, M., & Mortezaee, K. (2019). CD8+ cytotoxic T lymphocytes in cancer immunotherapy: A review. Journal of Cellular Physiology, 234(6), 8509-8521. [Google Scholar] [CrossRef]
- Fu, C., & Jiang, A. (2018). Dendritic cells and CD8 T cell immunity in tumor microenvironment. Frontiers in Immunology, 9, 3059. [Google Scholar] [CrossRef]
- Zhang, J., Endres, S., & Kobold, S. (2019). Enhancing tumor T cell infiltration to enable cancer immunotherapy. Immunotherapy, 11(3), 201-213. [Google Scholar] [CrossRef]
- Kim, S. S., Shen, S., Miyauchi, S., Sanders, P. D., & Franiak-Pietryga, I. (2020). B cells improve overall survival in HPV-associated squamous cell carcinomas and Are activated by radiation and PD-1 blockade. Clinical Cancer Research, 26(13), 3345-3359. [Google Scholar] [CrossRef]
- Patil, N. S., Nabet, B. Y., Müller, S., Koeppen, H., & Zou, W. (2022). Intratumoral plasma cells predict outcomes to PD-L1 blockade in non-small cell lung cancer. Cancer Cell, 40(3), 289-300.e4. [Google Scholar] [CrossRef]
- Kuroda, H., Jamiyan, T., Yamaguchi, R., Kakumoto, A., & Abe, A. (2021). Prognostic value of tumor-infiltrating B lymphocytes and plasma cells in triple-negative breast cancer. Breast Cancer, 28(4), 904-914. [Google Scholar] [CrossRef]
- Xie, G., Dong, H., Liang, Y., Ham, J. D., & Rizwan, R. (2020). CAR-NK cells: A promising cellular immunotherapy for cancer. EBioMedicine, 59, 102975. [Google Scholar] [CrossRef]
- Shimasaki, N., Jain, A., & Campana, D. (2020). NK cells for cancer immunotherapy. Nature Reviews Drug Discovery, 19(3), 200-218. [Google Scholar] [CrossRef]
- Wu, S. Y., Fu, T., Jiang, Y. Z., & Shao, Z. M. (2020). Natural killer cells in cancer biology and therapy. Molecular Cancer, 19(1), 120. [Google Scholar] [CrossRef]
- Pan, Y., Yu, Y., Wang, X., & Zhang, T. (2020). Tumor-associated macrophages in tumor immunity. Frontiers in Immunology, 11, 583084. [Google Scholar] [CrossRef]
- Xia, Y., Rao, L., Yao, H., Wang, Z., & Ning, P. (2020). Engineering macrophages for cancer immunotherapy and drug delivery. Advanced Materials, 32(40), e2002054. [Google Scholar] [CrossRef]
- Nowak, M., & Klink, M. (2020). The role of tumor-associated macrophages in the progression and chemoresistance of ovarian cancer. Cells, 9(5), 1299. [Google Scholar] [CrossRef]
- Le, F., Yang, L., Han, Y., Zhong, Y., & Zhan, F. (2021). TPL inhibits the invasion and migration of drug-resistant ovarian cancer by targeting the PI3K/AKT/NF-κB-signaling pathway to inhibit the polarization of M2 TAMs. Frontiers in Oncology, 11, 704001. [Google Scholar] [CrossRef]
- Yuan, X., Zhang, J., Li, D., Mao, Y., & Mo, F. (2017). Prognostic significance of tumor-associated macrophages in ovarian cancer: A meta-analysis. Gynecologic Oncology, 147(1), 181-187. [Google Scholar] [CrossRef]
- Van Coillie, S., Wiernicki, B., & Xu, J. (2020). Molecular and cellular functions of CTLA-4. Advances in Experimental Medicine and Biology, 1248, 7-32. [Google Scholar] [CrossRef]
- Datar, I., Sanmamed, M. F., Wang, J., Henick, B. S., & Choi, J. (2019). Expression analysis and significance of PD-1, LAG-3, and TIM-3 in human non-small cell lung cancer using spatially resolved and multiparametric single-cell analysis. Clinical Cancer Research, 25(15), 4663-4673. [Google Scholar] [CrossRef]
- Ai, L., Xu, A., & Xu, J. (2020). Roles of PD-1/PD-L1 pathway: Signaling, cancer, and beyond. Advances in Experimental Medicine and Biology, 1248, 33-59. [Google Scholar] [CrossRef]
- Demircan, N. C., Boussios, S., Tasci, T., & Öztürk, M. A. (2020). Current and future immunotherapy approaches in ovarian cancer. Annals of Translational Medicine, 8(24), 1714. [Google Scholar] [CrossRef]
- Yang, C., Xia, B. R., Zhang, Z. C., Zhang, Y. J., & Lou, G. (2020). Immunotherapy for ovarian cancer: Adjuvant, combination, and neoadjuvant. Frontiers in Immunology, 11, 577869. [Google Scholar] [CrossRef]
- Maiorano, B. A., Maiorano, M. F. P., Lorusso, D., & Maiello, E. (2021). Ovarian cancer in the era of immune checkpoint inhibitors: State of the art and future perspectives. Cancers, 13(17), 4438. [Google Scholar] [CrossRef]
Cite This Article
Yang, H. (2022). Comprehensive Analysis of the Expression and Clinical Significance of a Ferroptosis-Related Genome in Ovarian Serous Cystadenocarcinoma: A Study Based on TCGA Data. Oncologie, 24(4), 835–863. https://doi.org/10.32604/oncologie.2022.026447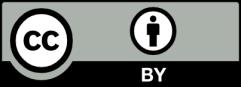