Open Access
ARTICLE
Modelling an Efficient Clinical Decision Support System for Heart Disease Prediction Using Learning and Optimization Approaches
Department of Computer Science and Engineering, J K K Munirajah College of Technology, Erode, 638506, India
* Corresponding Author: Sridharan Kannan. Email:
Computer Modeling in Engineering & Sciences 2022, 131(2), 677-694. https://doi.org/10.32604/cmes.2022.018580
Received 04 August 2021; Accepted 11 November 2021; Issue published 14 March 2022
Abstract
With the worldwide analysis, heart disease is considered a significant threat and extensively increases the mortality rate. Thus, the investigators mitigate to predict the occurrence of heart disease in an earlier stage using the design of a better Clinical Decision Support System (CDSS). Generally, CDSS is used to predict the individuals’ heart disease and periodically update the condition of the patients. This research proposes a novel heart disease prediction system with CDSS composed of a clustering model for noise removal to predict and eliminate outliers. Here, the Synthetic Over-sampling prediction model is integrated with the cluster concept to balance the training data and the Adaboost classifier model is used to predict heart disease. Then, the optimization is achieved using the Adam Optimizer (AO) model with the publicly available dataset known as the Stalog dataset. This flow is used to construct the model, and the evaluation is done with various prevailing approaches like Decision tree, Random Forest, Logistic Regression, Naive Bayes and so on. The statistical analysis is done with the Wilcoxon rank-sum method for extracting the p-value of the model. The observed results show that the proposed model outperforms the various existing approaches and attains efficient prediction accuracy. This model helps physicians make better decisions during complex conditions and diagnose the disease at an earlier stage. Thus, the earlier treatment process helps to eliminate the death rate. Here, simulation is done with MATLAB 2016b, and metrics like accuracy, precision-recall, F-measure, p-value, ROC are analyzed to show the significance of the model.Keywords
Cite This Article
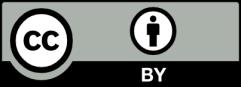