Open Access
ARTICLE
Prediction of Geopolymer Concrete Compressive Strength Using Convolutional Neural Networks
1 Department of Civil Engineering, VNR Vignana Jyothi Institute of Engineering and Technology, Hyderabad, Telangana, 500090, India
2 Department of Information Technology, VNR Vignana Jyothi Institute of Engineering and Technology, Hyderabad, Telangana, 500090, India
3 Department of Computer Science, LBEF Campus (Asia Pacific University of Technology & Innovation, Malaysia), Kathmandu, 44600, Nepal
4 Department of Computer Engineering, College of Computer and Information Sciences, King Saud University, P.O. Box 51178, Riyadh, 11543, Saudi Arabia
5 Guizhou Key Laboratory of Intelligent Technology in Power System, College of Electrical Engineering, Guizhou University, Guiyang, 550025, China
6 Operations Research Department, Faculty of Graduate Studies for Statistical Research, Cairo University, Giza, 12613, Egypt
7 Applied Science Research Center, Applied Science Private University, Amman, Jordan
* Corresponding Authors: Kolli Ramujee. Email: ; Ali Wagdy Mohamed. Email:
(This article belongs to the Special Issue: AI-Driven Engineering Applications)
Computer Modeling in Engineering & Sciences 2024, 139(2), 1455-1486. https://doi.org/10.32604/cmes.2023.043384
Received 30 June 2023; Accepted 09 November 2023; Issue published 29 January 2024
Abstract
Geopolymer concrete emerges as a promising avenue for sustainable development and offers an effective solution to environmental problems. Its attributes as a non-toxic, low-carbon, and economical substitute for conventional cement concrete, coupled with its elevated compressive strength and reduced shrinkage properties, position it as a pivotal material for diverse applications spanning from architectural structures to transportation infrastructure. In this context, this study sets out the task of using machine learning (ML) algorithms to increase the accuracy and interpretability of predicting the compressive strength of geopolymer concrete in the civil engineering field. To achieve this goal, a new approach using convolutional neural networks (CNNs) has been adopted. This study focuses on creating a comprehensive dataset consisting of compositional and strength parameters of 162 geopolymer concrete mixes, all containing Class F fly ash. The selection of optimal input parameters is guided by two distinct criteria. The first criterion leverages insights garnered from previous research on the influence of individual features on compressive strength. The second criterion scrutinizes the impact of these features within the model’s predictive framework. Key to enhancing the CNN model’s performance is the meticulous determination of the optimal hyperparameters. Through a systematic trial-and-error process, the study ascertains the ideal number of epochs for data division and the optimal value of k for k-fold cross-validation—a technique vital to the model’s robustness. The model’s predictive prowess is rigorously assessed via a suite of performance metrics and comprehensive score analyses. Furthermore, the model’s adaptability is gauged by integrating a secondary dataset into its predictive framework, facilitating a comparative evaluation against conventional prediction methods. To unravel the intricacies of the CNN model's learning trajectory, a loss plot is deployed to elucidate its learning rate. The study culminates in compelling findings that underscore the CNN model’s accurate prediction of geopolymer concrete compressive strength. To maximize the dataset’s potential, the application of bivariate plots unveils nuanced trends and interactions among variables, fortifying the consistency with earlier research. Evidenced by promising prediction accuracy, the study's outcomes hold significant promise in guiding the development of innovative geopolymer concrete formulations, thereby reinforcing its role as an eco-conscious and robust construction material. The findings prove that the CNN model accurately estimated geopolymer concrete's compressive strength. The results show that the prediction accuracy is promising and can be used for the development of new geopolymer concrete mixes. The outcomes not only underscore the significance of leveraging technology for sustainable construction practices but also pave the way for innovation and efficiency in the field of civil engineering.Keywords
Cite This Article
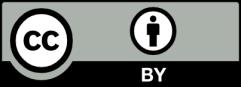