Open Access
ARTICLE
Blood Sample Image Classification Algorithm Based on SVM and HOG
1 Heilongjiang Province Cyberspace Research Center, Harbin, 150001, China
2 Harbin University of Science and Technology, Harbin, 150040, China
3 School of Cyberspace Science, Harbin Institute of Technology, Harbin, 150001, China
4 The Ohio State University, Columbus, 43202, USA
* Corresponding Author: Chao Ma. Email:
Journal of New Media 2022, 4(2), 85-95. https://doi.org/10.32604/jnm.2022.027175
Received 01 January 1970; Accepted 01 January 1970; Issue published 13 June 2022
Abstract
In the medical field, the classification and analysis of blood samples has always been arduous work. In the previous work of this task, manual classification maneuvers have been used, which are time consuming and laborious. The conventional blood image classification research is mainly focused on the microscopic cell image classification, while the macroscopic reagent processing blood coagulation image classification research is still blank. These blood samples processed with reagents often show some inherent shape characteristics, such as coagulation, attachment, discretization and so on. The shape characteristics of these blood samples also make it possible for us to recognize their classification through computer vision algorithms. Blood sample classification focuses on the texture and shape of the picture. HOG feature is a kind of feature descriptor used for object detection in computer vision and image processing. It can better extract the outline and texture features of the image by calculating and counting the histogram of oriented gradient of the local region of the image. Because the medical machines that need to identify and classify blood samples often lack strong calculation power, the current popular machine-learning classification algorithms cannot play a good role in these machines. In addition, the characteristics of blood samples produced by different types of reagents and processing methods are different, and it is difficult to obtain real samples, so the amount of data that can be used for training is small. Combining the above conditions and the experimental comparison of a variety of classification algorithms, we find that the lightweight SVM model has a better performance on this problem, and the combination of HOG and SVM has also been widely used in other research. The experiment demonstrated that the classification algorithm based on SVM and HOG can give a good result of both performance and accuracy in the classification of blood samples problem.Keywords
Cite This Article
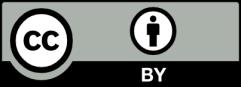