Open Access
Ring finger protein 157 is a prognostic biomarker and is associated with immune infiltrates in human breast cancer
1 School of Life Sciences, Hainan University, Haikou, 570228, China
2 Department of Medical Laboratory, Hainan Cancer Hospital, Affiliated Cancer Hospital of Hainan Medical University, Hainan Tropical Cancer Research Institute, Haikou, 570312, China
3 Department of Laboratory Medicine, The Sixth Affiliated Hospital of Guangzhou Medical University, Qingyuan People’s Hospital, Qingyuan, 511518, China
4 Graduate School, Guangzhou University of Chinese Medicine, Guangzhou, 510006, China
5 School of Pharmaceutical Sciences, Hainan University, Haikou, 570228, China
* Corresponding Authors: BIN XIAO. Email: ; LINHAI LI. Email:
; XINPING CHEN. Email:
# These two authors equally contributed to this work
BIOCELL 2023, 47(10), 2265-2281. https://doi.org/10.32604/biocell.2023.029195
Received 06 February 2023; Accepted 24 May 2023; Issue published 08 November 2023
Abstract
Background: The protein encoded by ring finger protein 157 (RNF157) is known to function as an E3 ubiquitin ligase. However, whether the level of RNF157 expression in breast cancer correlates with prognosis and immune cell infiltration among breast cancer patients remains to be further explored. Methods: In this study, publicly available datasets were used for evaluating RNF157 expression in different tumors compared with normal samples. Several independent datasets were screened for investigating the relationship between RNF157 and breast cancer survival, different mutation profiles, and tumor immune cell infiltration. We conducted a pathway enrichment analysis to identify signaling pathways associated with RNF157. Results: Analysis of public and online databases revealed that RNF157 expression markedly decreased in breast cancer tissue samples compared to non-carcinoma counterparts. Consistently, immunohistochemistry assays also demonstrated this RNF157 down-regulation in breast cancer samples. RNF157 up-regulation could predict the improved survival of breast cancer cases. Further, different RNF157 expression level groups exhibited different mutational profiles. Pathway enrichment profiling of RNF157-related genes suggested its possible involvement in regulating breast cancer via the mitogen-activated protein kinase (MAPK) pathway. RNA sequencing (RNA-seq) data and genomic enrichment analysis showed that RNF157 downregulated several genes positively associated with the MAPK signaling pathway. We also explored RNF157 expression and immune cell infiltration in breast cancer and found that RNF157 mRNA levels were negatively related to non-T immune cell infiltration. Conclusion: According to our work, RNF157 may be a promising diagnostic biomarker and therapeutic target for breast cancer.Keywords
Abbreviation List
AUC | Area Under the Curve |
BRCA | Breast Cancer |
CHDs | Congenital Heart Defects |
CAN | Copy Number Alteration |
CI | Confidence Interval |
CCL7 | C-C motif Chemokine Ligand 7 |
CCL20 | C-C motif Chemokine Ligand 20 |
CXCL5 | C-X-C motif Chemokine Ligand 5 |
DMEM | Dulbecco’s Modified Eagle Medium |
DMFS | Distant Metastasis-free Survival |
DCs | Dendritic Cells |
ER | Estrogen Receptor |
FBS | Fetal Bovine Serum |
GEO | Gene Expression Omnibus |
GO | Gene Ontology |
Her2: | Human epidermal growth factor receptor 2 |
Hh signaling | Hedgehog signaling |
HR | Hazard Ratio |
IQR | Interquartile Range |
KEGG | Kyoto Encyclopedia of Genes and Genomes |
LUMB | Luminal B |
MAPK | Mitogen-activated Protein Kinase |
MEGF8 | Multiple Epidermal Growth Factor-like Domains 8 |
M0 | no distant metastasis |
M1 | M stage I |
N0 | no regional lymph node metastasis |
N1 | N stage I |
N2 | N stage II |
N3 | N stage III |
NK | Natural Killer |
OS | Overall Survival |
PR | Progesterone Receptor |
PAM50 | Prediction Analysis of Microarray 50 |
RNF157 | Ring Finger Protein 157 |
RNA-seq | RNA sequencing |
RNF157-AS1 | RNA 1 of RNF157 |
RMA | Robust Multichip Average |
RFS | Recurrence-free Survival |
ROC | Receiver Operating Characteristic |
SDS | Sodium Dodecyl Sulfate |
TCGA | The Cancer Genome Atlas |
T1 | T stage I |
T2 | T stage II |
T3 | T stage III |
T4 | T stage IV |
TME | Tumor Microenvironment |
Tregs | Regulatory T cells |
In 2020, female breast cancer replaced lung cancer as the cancer type with the highest morbidity globally (Sung et al., 2021). Systemic treatment of early-stage breast cancer includes endocrine therapy, chemotherapy, targeted therapy, and immunotherapy (Duffy et al., 2017; Andre et al., 2022). There is currently no evidence to suggest that drug prevention reduces breast cancer mortality, and all drugs available only have the potential to prevent estrogen receptor (ER)-positive breast cancer (Britt et al., 2020). While radiotherapy remains an important cornerstone of breast cancer treatment, novel combinations of molecular biological markers, including immunohistochemical markers, genomic markers, and immune markers, provide an important foundation for increasingly sophisticated diagnostic approaches (Loibl et al., 2021). As a result, it is urgently needed to identify novel biomarkers for the diagnosis and prognosis of b-reast cancer to facilitate the development of new therapeutic approaches.
Ring Finger Protein 157 (RNF157) is a RING-Type E3 ubiquitin ligase and the gene is located on chromosome 17q25.1. RNF157 acts as a proteasomal degradation mediator involved in protein ubiquitination and degradation, thus affecting cell cycle, cell apoptosis, and gene transcription, among other physiological processes (Matz et al., 2015b; Dogan et al., 2017; Kosacka et al., 2018; Kong et al., 2020; Lin et al., 2021; Qi et al., 2022). In one report, silencing RNF157 expression led to G2/M phase arrest and induced apoptosis in melanoma cells (Dogan et al., 2017). Further, RNF157 could protect HLE-B3 cells from apoptosis by interacting with the tumor antigen p53 to promote its ubiquitination and degradation in human cataract samples (Qi et al., 2022). Knockdown of RNF157 was reported to promote neuronal apoptosis by ubiquitinating APBB1 and reduce its interaction with Tip11 (Matz et al., 2015b). Additionally, RNF157 upregulation was involved in the LY294002-mediated activation of autophagy in adipose tissue and activated apoptosis via the cleaved caspase‑3 signal pathway (Kosacka et al., 2018). A study showed that RNF157 combined with multiple epidermal growth factor-like domains 8 (MEGF8) to form a ubiquitin protease complex that promoted emergence of congenital heart defects (CHDs) by increasing catalyzing smoothened (SMO) ubiquitination and increased the strength of Hedgehog (Hh) signaling (Kong et al., 2020). According to a bioinformatic analysis, antisense RNA 1 of RNF157 (RNF157-AS1) expression was notably lower in ovarian cancer tissues. Furthermore, patients with low RNF157-AS1 expression had a lower survival rate (Lin et al., 2021). Despite such findings, RNF157 expression in breast cancer and its potential significance as a prognostic marker are yet to be established.
This study focused on investigating the relation between RNF157 expression and breast cancer prognosis. For this, we first obtained RNA-seq data for breast cancer samples using a publicly available dataset for analyzing differential RNF157 gene expression within pan-cancer and breast cancer samples. Immunohistochemistry and western blotting were then carried out for probing RNF157 levels in clinical breast cancer tissues and breast cancer cell lines. We also analyzed the clinical significance and gene mutations of RNF157 in breast cancer using several independent datasets. Additionally, functional enrichment analysis and the analysis of immune cell infiltration and migration of RNF157 were carried out to explore its potential diagnostic value in breast carcinogenesis and clinical prognosis.
Public data set processing for breast cancer
RNA-seq data based on The Cancer Genome Atlas (TCGA) database (https://portal.gdc.cancer.gov) was obtained and compiled from The Cancer Genome Atlas Breast Invasive Carcinoma (TCGA-BRCA) (breast invasive cancer) project STAR process. The data was extracted in the TPM format to remove non-clinical and duplicate information and then processed as log2 (value+1). Data was visualized using the ggplot2 package. We downloaded data from two Gene Expression Omnibus (GEO) datasets to obtain more clinical information related to breast cancer patients: GSE42568 (Clarke et al., 2013) (104 breast cancer and 17 normal breast biopsies) and GSE70947 (Barrett et al., 2013) (Age and estrogen-dependent inflammation in 148 breast adenocarcinoma and 148 normal breast tissue). All microarray data were called with the robust multichip average (RMA) method. We used the TIMER2.0 (http://timer.cistrome.org/) database for analyzing RNF157 levels among diverse cancers together with non-carcinoma samples (Li et al., 2020). A probability cutoff of 0.05 was applied.
Correlation between RNF157 and the tumor mutation burden
We employed cBioPortal web to carry out genetic (https://www.cbioportal.org/) alteration analyses (Gao et al., 2013). In addition, a “curated set of non-redundant studies” (184 studies, 10528 samples) from the “query” section was selected, and “RNF157” was imported for queries regarding genetic alteration features related to RNF157. Using the “cancer types summary” module, the alteration frequency, mutation type, and copy number alteration (CAN) results in many cancer types were obtained. Moreover, data on mutated sites of RNF157 were observed in the “mutations” module. We also used the “comparison/s-urvival” module for obtaining information on the differences in the overall survival (OS) and the relapse-free survival of patients with/without RNF157 genetic alterations.
Functional enrichment analysis
We carried out a bulk correlation analysis of RNF157 and all other molecular data in the TCGA database to find the biological pathways enriched by RNF157 using breast cancer transcriptome sequencing. The parametrization settings were |Cor| > 0.3, and p < 0.05. We then conducted an enrichment of the first 50 genes related to RNF157 in breast cancer using the R cluster Profiler package (Yu et al., 2012) for Gene Ontology (GO) and Kyoto Encyclopedia of Genes and Genomes (KEGG) pathways (Liao et al., 2019). Statistical significance was set at the corrected p < 0.05. Finally, we utilized the ggplot2 package for creating a visual representation of the results obtained from the enrichment analysis.
Breast cancer cell lines namely MDA-MB-231, HS578T, UACC812, MCF7, and T47D were cultivated in Dulbecco’s Modified Eagle Medium (DMEM) with 10% fetal bovine serum (FBS) (Gibco, Detroit, MI, USA). HCC1954 cells were cultured with RPMI1640 (Gibco, Detroit, MI, USA) supplemented with 10% FBS. Normal breast epithelial MCF10A cells were cultivated within MEBM (Lonza, Switzerland) that contained 10% FBS. Cells were incubated at 37°C and an atmosphere of 5% CO2.
Total cellular protein extracts were obtained by lysing the cells with concentrated RIPA lysis buffer containing protease inhibitors. The extracted proteins were then separated using 10% sodium dodecyl sulfate (SDS)-polyacrylamide gel electrophoresis (PAGE) and transferred to polyvinylidene difluoride (PVDF) membranes. We added 5% skim milk to the membrane for 1h at room temperature to block non-specific binding. The membranes were then probed using primary antibodies against RNF157 (1:1000, #26189-1-AP, Abnova, Taiwan) and β-actin (1:5000, #3700T, Abcam, USA) for 24 h at 4°C. Western blots were observed and measured for intensity (Guo et al., 2015; Bai et al., 2019).
We used a total of 34 breast cancer tissues and 20 normal breast tissues for immunohistochemical (IHC) staining. The primary antibody against RNF157 (1:300 dilution, #bs-9226R; Bioss, China) was incubated with the samples at 4°C overnight. The sections were then treated with peroxidase-conjugated goat anti-rabbit secondary antibody (1:2,000 dilution, A0181; Beyotime, Shanghai, China) and incubated at room temperature for 2 h. Thereafter, 3,3′-Diaminobenzidine was added for IHC staining, followed by counterstaining with hematoxylin (Beyotime, Shanghai, China). The IHC staining results were analyzed and scored by two pathologists blinded to sample origin. The differences in RNF157 expression between breast cancer tissues and non-carcinoma counterparts were assessed based on the mean H-score.
We employed R version 4.2.1 (R Foundation for Statistical Computing, Vienna, Austria) and GraphPad Prism 8.4 (GraphPad Software, Inc., San Diego, CA) to carry out all statistical analyses. Breast cancer patients were classified into two groups according to the median RNF157 gene expression level based on the TCGA database: low and high RNF157 expression groups. The overall survival between the two groups was analyzed by Kaplan-Meier (KM) curves and Wilcoxon log-rank tests. COX regression models were employed to perform univariate and multivariable analyses, while Spearman correlation was utilized for evaluating the correlation of RNF157 gene expression with other genes. RNF157-related genes were identified using Spearman’s correlation analysis.
Accession numbers of RNA, DNA and protein sequences used in the manuscript should be provided.
Low expression of RNF157 in breast cancer
We have presented a flowchart to demonstrate our analysis methodology (Fig. 1). The analysis of TCGA RNA-seq data in the TIMER database revealed a significant downregulation of RNF157 mRNA expression in breast cancer, glioblastoma multiforme, kidney chromophobe, lung squamous cell carcinoma, skin cutaneous melanoma, and thyroid carcinoma compared to their respective non-cancerous counterparts (Fig. 2A). We employed the TCGA database for assessing RNF157 mRNA expression among breast cancer cases compared to expression in non-carcinoma samples. We used the TCGA database to assess RNF157 mRNA expression in breast cancer cases compared to expression in non-cancerous samples. We found that RNF157 expression markedly decreased within breast cancer samples as compared with para-carcinoma samples (p < 0.01) (Fig. 2B). An identical expression pattern was also confirmed in breast cancer samples and paired paraneoplastic tissues (Fig. 2C). We further analyzed two other independent external GEO datasets, GSE42568 and GSE70947 were analyzed to determine RNF157 mRNA expression within breast cancer tissues and non-carcinoma counterparts. The RNF157 mRNA expression level in breast cancer samples remarkably decreased compared with unpaired and paired samples (p < 0.001) (Figs. 2D and 2E).
Figure 1: The flowchart illustrates our data collection and processing.
Figure 2: The expression level of RNF157 in different human cancers. (A) TIMER was used to detect the expression levels of RNF157 in different tumors in The Cancer Genome Atlas (TCGA) database. (B and C) The expression level of RNF157 in unpaired tissues and paired adjacent tissues. (D and E) The expression level of RNF157 in unpaired tissues and paired adjacent tissues of GSE42568 and GSE70947 datasets. (F–I) The tumor tissues from patients with different clinical characteristics in TCGA [pathologic stage (F), progesterone receptor (PR) stage (G), estrogen receptor (ER) stage (H), and age (I)]. *p < 0.05, **p < 0.01, ***p < 0.001, ns, no significant.
RNF157 mRNA expression was also examined within diverse clinical categories based on the TCGA database to determine the correlation of RNF157 level with clinical characteristics of breast cancer cases. The relation of the RNF157 level with clinical characteristics in breast cancer cases is shown in Table 1. We found that the low expression of RNF157 was significantly related to the histological type (p < 0.001), progesterone receptor (PR) status (p < 0.001), ER status (p < 0.001), PAM50 (p < 0.001) and age (p < 0.001) of these patients (Figs. 2F–2I and Table 1). We then analyzed the correlation of RNF157 expression with the methylation status based on the UALCAN database to elucidate the mechanism underlying the aberrant down-regulation of RNF157 in breast cancer samples. The methylation level of RNF157 in breast cancer samples was evidently decreased compared to their para-carcinoma counterparts (Suppl. Fig. S1), indicating that the abnormally low expression of RNF157 in breast cancer may be related to significantly lower methylation levels.
We examined breast cancer tissues from 34 patients and 20 corresponding adjacent tissue samples using immunohistochemistry for exploring the possible role of RNF157 in breast carcinogenesis. The results demonstrated weaker positive staining for RNF157 in breast cancer samples than the non-carcinoma samples, as shown in Figs. 3A and 3B. We further investigated RNF157 protein levels among seven breast cancer cell lines (MCF10A, MCF7, T47D, UACC812, HCC1954, MDAMB231, HS578T) and compared them with that of human breast epithelial cells. We observed a decrease in the abundance of RNF157 protein in cancer cell lines, except for the MDAMB231 cell line (Fig. 3C).
Figure 3: RNF157 expression in clinical specimens of breast cancer and breast cancer cell lines. (A) Immunohisto-chemical staining of RNF157 was performed in tumor tissues (n = 34) and paracarcinoma tissues (n = 20). Representative images are shown. Score bars, 50 mm. (B) Staining was quantified as shown. The dot plot depicts the means and standard deviation of 54 images of tumor tissues and adjacent normal tissues. (C) Western blot detecting the protein expression level of RNF157 in different breast cancer cell lines. **p < 0.01.
Low RNF157 expression predicted poor clinical outcomes of breast cancer and had diagnostic significance
To determine whether RNF157 expression is related to patient prognosis, we divided breast cancer cases derived from the TCGA database into two groups, the high and low RNF157 expression groups. The survival analysis based on the mean expression value of RNF157 was then performed. Overall survival analysis by the Kaplan Meier method suggested that low expression levels of RNF157 might predict poor OS (HR = 0.69, p = 5.7e-03) (Fig. 4A), distant metastasis-free survival (DMFS) (HR = 0.61, p = 2.9e-04) (Fig. 4B) and recurrence free survival (RFS) (HR = 0.7, p = 2.9e-06) (Fig. 4C).
Figure 4: Kaplan–Meier survival curve analysis of the prognostic significance of RNF157 using the cancer genome atlas (TCGA) database and the diagnostic value of RNF157 expression in breast cancer. (A) Kaplan–Meier estimates of the overall survival (OS) probability of TCGA dataset patients for all breast cancer patients. (B and C) Subgroup analysis for relapse-free survival (RFS) (B) and distant metastasis-free survival (DMFS) (C). (D) Receiver operating characteristic (ROC) curve analysis for RNF157 expression in breast cancer and adjacent tissues. (E) Nomogram inclusive of RNF157 and independent clinical risk factors to predict 1-, 3-, and 5-year breast cancer survival probabilities. (F) Nomograms calibrated to predict the probabilities of 1-, 3- and 5-year survival. The gray line represents the actual survival.
We also analyzed the significance of RNF157 expression in predicting the prognosis of different breast cancer subgroups. The RNF157 down-regulation showed a positive relation with breast cancer patient cases at stage N3 (HR = 0.22, p = 0.016), with luminal B (LUMB) (HR = 0.44, p = 0.033) and with basal (HR = 0.45, p = 0.031) and were significantly associated with poor prognosis (Table 2).
The univariate logistic regression illustrated that the RNF157 level was the categorical dependent variable, which predicted the dismal prognosis-related clinicopathological features (Table 3). Further, the down-regulated RNF157 expression in breast cancer was positively related to the histological type (OR = 2.157 for infiltrating lobular carcinoma vs. infiltrating ductal carcinoma), the PR status (OR = 2.297 for indeterminate and positive vs. negative), the ER status (OR = 3.026 for indeterminate and positive vs. negative), and PAM50 (OR = 0.416 for LumA and luminal B (LUMB) and Her2 and basal vs. normal). All were significant (p < 0.01).
Univariate Cox analysis was applied to evaluate the factors influencing the OS. We found that a low expression RNF157 (HR = 0.640, 95% CI = 0.414–0.989, p = 0.045) was the predictive factor for worse OS. This was also seen for TNM stage (p < 0.001) and the histological type (p < 0.05) (Table 4). We then incorporated these factors were for multivariate Cox regression. It was observed that low RNF157 expression was an independent predictor of poor OS for breast cancer cases.
We next plotted the receiver operating characteristic (ROC) curve for investigating whether RNF157 expression could be used to distinguish breast cancer tissues and non-carcinoma counterparts. As shown in Fig. 4D, an area under the ROC curve (AUC) value of 0.831 was obtained, indicating RNF157 was a potential biomarker for diagnosing breast cancer.
We then constructed nomograms using the RNF157 expression and independent clinical risk factors based on multivariate Cox analysis to better predict the prognosis of breast cancer. The sum of the scores assigned to each clinical risk factor in the model was rescaled to a range of 1 to 100 using a point scale, depending on the degree of contribution of each clinical risk factor to the outcome variable (the magnitude of the regression coefficient). The total score was obtained by adding up the individual scores, and eventually, the survival probability for breast cancer patients at 1-, 3-, and 5-years was predicted by transforming the relation of total score with outcome event probability into a function (Fig. 4E). The calibration curve was close to the ideal curve, which suggested that the predictions were satisfactorily linear (Fig. 4F). Overall, the above data indicated that RNF157 may be a candidate prognostic biomarker of OS for breast cancer patients.
RNF157 mutation characteristics in pan-cancer analysis
We used the cBioPortal network for studying RNF157 genetic alterations in human pan-cancer samples for understanding mutational profile of RNF157 during carcinogenesis. We found that the highest RNF157 “amplification” alteration frequency appeared for patients with breast cancer. Further, the “mutations” type was the least alteration frequency of RNF157 with breast cancer (>4% alteration frequency) (Fig. 5A). The genetic alterations of RNF157 including types, sites, and case numbers have been shown in Fig. 5B. Missense mutation represented the major genetic alteration type of RNF157, and it was detected among 85 cases. Additionally, truncating mutations occurred in six patients. Splice mutations and fusions were detected only in four cases.
Figure 5: Mutational features of RNF157 in pan-cancer analysis. (A) Alteration frequency with the mutation type of RNF157 in human pan-cancer. (B) Sites and case numbers of the RNF157 genetic alteration are presented. (C and D) Association between RNF157 genetic alterations and clinical survival. (C) Overall survival (OS) (D) Relapse-free survival.
The relationship of genetic alterations in RNF157 with the clinical outcome of breast cancer was then analyzed. As shown in Figs. 5C and 5D, breast cancer cases with RNF157 alterations showed poor OS (p = 0.0437) and relapse-free survival (p = 1.202e-4). These results demonstrate that genetic alterations of RNF157 in breast cancer may be involved in altered RNF157 mRNA expression and poor clinical survival.
RNF157 is closely related to key factors in breast cancer and the mitogen-activated protein kinase signal pathway regulation in breast cancer
We analyzed the co-expression pattern of with other genes in breast cancer in the TCGA database for understanding its biological activity. We screened 25 positively correlated genes that displayed the greatest Spearman correlation coefficients along with 25 negatively correlated genes, which were illustrated by the heat map (Fig. 6A). To further understand the functional implications of these 50 genes that were most associated with RNF157 in breast cancer, functional enrichment analysis using GO and KEGG was done with the R cluster Profiler package. The results indicated that the MAPK signaling pathway and several microRNAs in breast cancer were enriched among these genes (Fig. 6B).
Figure 6: Functional clustering and correlation analysis of RNF157 with the mitogen-activated protein kinase (MAPK) pathway regulatory genes in The Cancer Genome Atlas (TCGA). (A) Heatmap showing the top 50 genes in breast cancer that were positively and negatively related to RNF157. Red represents positively related genes and blue represents negatively related genes. (B) Gene Ontology (GO) term and Kyoto Encyclopedia of Genes and Genomes (KEGG) pathway analyses of the RNF157-related genes in breast cancer. (C) Heatmap showing that the MAPK signaling pathway regulatory genes were positively or negatively associated with RNF157. Red represents positively related genes and blue represents negatively related genes. (D–G) Correlation analysis between RNF157 and the MAPK signaling pathway regulation genes in breast cancer in The Cancer Genome Atlas (TCGA). (D) MAP3K12, (E) ACVR1B, (F) MAP2K5 and (G) MAP4K3. ***p < 0.001.
To confirm whether RNF157 in breast cancer is associated with the 245 genes that were shown to be related to the MAPK signaling pathway (https://pathcards.genecards.org/) (Belinky et al., 2015), we constructed another heat map (Fig. 6C). The RNF157 down-regulation in breast cancer patients showed significant correlation with these genes. Moreover, the relationship of RNF157 with MAPK signaling pathway-related genes in breast cancer was further examined. We found that MAPK signaling pathway-related genes MAP3K12, ACVR1B, MAP2K5, and MAP4K3 showed a positive correlation with RNF157 (r > 0.3, p < 0.001) (Figs. 6D–6G). Collectively, RNF157 showed a close association with MAPK signaling pathway regulation in breast cancer.
Association of RNF157 with immune cell infiltration in breast cancer
Immune cells of the adaptive and innate immune systems infiltrate into the tumor microenvironment (TME) and modulate cancer development (Seager et al., 2017). The immune infiltration in breast cancer showing diverse RNF157 expression was analyzed using the TIMER database to explore the link between RNF157 expression and tumor immune response. We found that natural killer (NK) cells, CD8 + T lymphocytes, CD56 bright cells, mast cells, and eosinophils exhibited remarkably decreased infiltration levels in breast cancer cases showing RNF157 down-regulation compared with those showing RNF157 up-regulation. On the contrary, activated dendritic cells (DCs), macrophages, NK CD56 dim cells, B cells, and regulatory T cells (Tregs) displayed significantly increased infiltration levels in breast cancer cases with RNF157 down-regulation compared to those showing RNF157 up-regulation. The infiltration levels of T cells and DC cells were not significantly different in both groups (Fig. 7A). Caption and used consistently thereafter. Accepted abbreviations for statistical parameters are: P, n, SD, SEM, df, ns, ANOVA, t. Naming of chemicals should follow that given in Chemical Abstracts Service.
Figure 7: Correlation analysis of RNF157 expression and immune infiltration in breast cancer. (A) Differential distribution of immune cells in patients with high RNF157 expression and low RNF157 expression. (B–E) The expression of RNF157 in breast cancer was correlated with immune cells infiltration. (B) B cells, (C) macrophages, (D) B cells, and (E) regulatory T cells (Tregs). **p < 0.01, ***p < 0.001.
The relation of RNF157 levels with immune infiltration in breast cancer was also examined. The RNF157 expression showed a positive relation with the infiltration level of mast cells (Fig. 7B, r = 0. 236, p < 0.001). However, RNF157 expression showed a negative relation with macrophage (Fig. 7C, r = 0.288, p < 0.001), B cells (Fig. 7D, r = 0. 279, p < 0.01), and Treg (Fig. 7E, r = 0.227, p < 0.01) infiltration levels.
The heat map displaying significant relations of the RNF157 level and with chemokines and chemokine receptors in breast cancer are shown in Figs. 8A and 8B. We synthetically examined the relation of the RNF157 level with chemokines and chemokine receptors to understand the relationship between the RNF157 level and the migration of immune cells. RNF157 expression showed a negative relation with CCL7 (r = −0.274, p = 3.05e-20), CCL20 (r = −0.28, p = 3.27e-21), and CXCL5 (r = −0.262, p = 1.4e-18) (Figs. 8C–8E). However, RNF157 was not related to other chemokines/chemokine receptors (−0.3 < r < 0.3). Collectively, RNF157 displayed a negative relation with chemokine levels and chemokine receptor levels in breast cancer.
Figure 8: Correlation analysis between RNF157 expression and chemokines and/or chemokine receptors. (A) Heatmap analysis of the correlation between RNF157 and chemokines in pan-cancer analysis. (B) Heatmap analysis of the correlation between RNF157 and chemokine receptors in pan-cancer samples. (C–E) RNF157 expression in breast cancer was negatively correlated with C-C motif chemokine ligand 7 (CCL7), C-C motif chemokine ligand 20 (CCL20), and C-X-C motif chemokine ligand 5 (CXCL5).
Human RNF157 is an E3 ubiquitin ligase that functions in different aspects of neuronal development. Specifically, RNF157 promotes neuronal survival by hydrolyzing the bridging protein amyloid beta precursor protein binding family B member 1 (APBB1) (Matz et al., 2015a). Further, RNF157 expression was found to promote the development of spontaneous hypertension (Ramachandran et al., 2020). RNF157 was found to promote prostate cancer progression by ubiquitinating HDAC1 leading to macrophage M2 polarization (Guan et al., 2022). In another report, RNF157-AS1 enhanced the sensitivity of tumor cells, including EOC cells, to cisplatin (Xu et al., 2023). Interestingly, it appears that RNF157-AS1 may play a role in regulating HCC progression and triggering resistance to adriamycin through metabolic pathways such as fatty acid metabolism and oxidative phosphorylation (Zhang et al., 2022). These findings are particularly intriguing and suggest that RNF157-AS1 may have significant therapeutic potential in cancer treatment. Additionally, RNF157-AS1 down-regulation has been previously suggested to predict a poor prognostic outcome in ovarian cancer (Lin et al., 2021). Notwithstanding these findings, little research regarding RNF157 within breast cancer is available. Our work focused on comprehensively determining the biological activity of RNF157 and its possible regulatory pathways in breast cancer using bioinformatics analysis.
Bioinformatics analysis was conducted in the present work using TCGA-derived high-throughput RNA sequence data. According to our results, RNF157 mRNA expression decreased in breast cancer samples and was related to clinicopathological features. Low RNF157 expression predicted reduced OS, RFS, and DMFS as shown by Kaplan-Meier curves, univariate regression, and multivariate regression. Hence, RNF157 is a candidate biomarker to potentially diagnose and differentiate breast cancer from non-carcinoma tissues. The mutational characterization of RNF157 in pan-cancer samples showed that “amplification” was the most frequent alteration type (>4%) in breast cancer type. Further, the missense mutation of RNF157 represented the main genetic alteration type, and was detected in 18 cases. Genetic alterations of RNF157 in breast cancer may be involved in poor clinical survival.
Some findings show that tumor cell growth-related signaling pathways are often over-activated in cancer (Zhao et al., 2021). The MAPK/Extracellular signal-regulated kinase 1/2 (ERK) signaling pathway was highly activated and plays a pivotal role in promoting tumor growth in breast cancer (Di et al., 2015). GO and KEGG analyses were conducted for analyzing the molecular mechanism underlying RNF157-induced breast cancer development. We found that low expression of RNF157 was related to the MAPK pathway, microRNAs in cancer pathway, mitophagy animal pathway, and the arginine biosynthesis pathway. The MAPK signaling pathway-related gene set showed the highest enrichment in the high-RNF157-expression group. The reported correlation between MAPK signaling pathway regulatory genes and RNF157 reaffirms that high expression of RNF157 may inhibit breast cancer progression mainly through the regulation of the MAPK signaling pathway. Further, we found that RNF157 showed a positive correlation with the MAPK signaling pathway regulatory genes, such as MAP3K12, ACVR1B, MAP2K5, and MAP4K3. According to a report, MAP3K12 triggered apoptosis in breast cancer cells by interacting with TG2 to inhibit calmodulin C (Robitaille et al., 2008). Further, MAP2K5 up-regulates ZEB1 and SNAI2 levels to accelerate the epithelial–mesenchymal transition (EMT) phenotype of breast cancer cells (Zhou et al., 2008). MAP2K5 contributes to the consistent phosphorylation of ERK5, which then enhances its nuclear translocation and activates the MAP2K5-ERK5 pathway toincrease thyroid epithelial cell malignancy (Ye et al., 2019). Based on high-throughput data on knocking down MAP4K3-induced cell viability, MAP4K3 was a new therapeutic target for breast cancer (Park et al., 2015). Thus, RNF157 may have the potential to suppress cell proliferation and migration of breast cancer by downregulating specific genes involved in the MAPK signaling pathway.
The TME has an important impact in diagnosing and predicting tumor prognosis and estimating tumor sensitivity to clinical treatments (Ciavarella et al., 2018). As a major component of the TME, immune infiltration has been exhibited to contribute to tumor progression and immunotherapeutic response (Balkwill et al., 2012; Zhang and Zhang, 2020).
On these lines, our results showed that there were fewer NK cells, CD8+ T lymphocytes, CD56 bright cells, mast cells, and eosinophils in the RNF157 down-regulation group compared to the RNF157 up-regulation group. More numbers of activated DCs were revealed in the RNF157 down-regulation cancer group compared to the RNF157 up-regulation group. NK cells and TAM infiltrated in the TME were shown to trigger a strong immunosuppressive activity, which reduces IFN-g secretion and induces T cell dysfunction (Solinas et al., 2009; Platonova et al., 2011). Subgroups with more infiltrated regulatory T cells in breast and ovarian cancers were less likely to develop lymph node metastasis and have a better prognosis, suggesting that regulatory T cells have anti-tumor effects (Punkenburg et al., 2016; Salazar et al., 2020; Qi et al., 2022). Further, PD-L1(+) tumors had greater CD8(+) T-cell infiltrates than PD-L1(−) tumors (688 cells/mm vs. 263 cells/mm; p < 0.0001) (Lee et al., 2022). Additionally, the MEK5 extracellular signal-regulated kinase (Erk)5 crosstalk mediates signals from various extracellular stimuli to the nucleus and regulates most cellular processes (Zhou et al., 1995). This is inclusive of gene expression, proliferation, apoptosis, and motility (Chang and Karin, 2001; Johnson and Lapadat, 2002).
Chemokines exert critical effects on directional immune cell migration (Ley, 2003; Kanemitsu et al., 2005; Pallandre et al., 2008; Phua et al., 2019). They can promote leukocyte subpopulations to specifically migrate to the TME or inflammation sites (Thelen, 2001). The corresponding ligands combine with the receptor’s extracellular N-terminal, causing serine/threonine residue phosphorylation in the cytoplasmic C-terminal, thereby inducing signaling as well as receptor desensitization (Thelen, 2001). The chemokines and their receptor pairs can regulate cell migration and influence cell activities such as growth, survival, migration and invasion by modulating chemokine expression (Baggiolini et al., 1997; Fulton, 2009). According to our results, the relation between RNF157 expression and immune cell chemokine levels within breast cancer was examined using the TISIDB database. Chemokine CCL7 was found to promote the growth and self-renewal of breast cancer cells (Rajaram et al., 2013; Wu et al., 2015).
An intraperitoneal injection of anti-CCL20 antibody could inhibit bone metastasis of osteolytic breast cancer cells in mice (Lee et al., 2017; Chen et al., 2018). The RNF157 expression showed a negative correlation with CCL7, CCL20 and CXCL5 levels, indicating that RNF157 down-regulation might impact immune cell migration to the TME.
Although we combined data on the function of RNF157 in breast cancer from several databases, certain limitations should still be noted in our present work. Firstly, the analysis of immune cell markers may introduce systematic bias as we analyzed tumor tissue data to collect RNFI157 microarray and sequencing data. Secondly, the present work just performed a bioinformatic analysis of the effect of RNF157 on breast cancer using many databases. Both in vitro and in vivo experiments should be performed for demonstrating how RNF157 affects MAPK signaling pathway in tumor cells. Thirdly, we concluded that RNF157 expression was tightly related to immune infiltration as well as the prognostic outcome of breast cancer. However, direct evidence of whether RNF157 could affect prognosis through its involvement in immune infiltration is lacking, which deserves more studies.
To conclude, RNF157 expression was markedly decreased in breast cancer, which strongly predicted the dismal prognostic outcome of breast cancer cases. Further, RNF157 is a potential promising biomarker to diagnose and predict breast cancer prognosis. RNF157 may influence breast cancer progression by regulating the MAPK signaling pathway to influence the breast cancer cell cycle and immune infiltration.
Our study demonstrated that RNF157 might be a promising biomarker used to predict breast cancer prognosis. The effects of low RNF157 expression and its prognostic value need to be verified in more breast cancer clinical samples and patients with long-term follow-up data. The molecular mechanism of how RNF157 affects the MAPK signaling pathway is also a worthwhile avenue for investigation.
Acknowledgement: None.
Funding Statement: The present study was funded by the Innovation Team Project of Hainan Natural Science Foundation (820CXTD446) and the Technology Program of Qingyuan (No. 2022KJJH027 to Linhai Li). We extend our gratitude to all members involved in the present work. Besides, we thank TCGA, Timer and UALCAN databases for their open-access usage facilities.
Author Contributions: The authors confirm contribution to the paper as follows: XPC, LLH, and BX designed this work; XZ, WWZ and XYS collected and analyzed the data; XZ and GW carried out the in vitro experiments; XZ and BX were in charge of the manuscript writing. All authors reviewed the results and approved the final version of the manuscript.
Availability of Data and Materials: The data used in the work appear in the submitted paper and can be obtained from the TCGA and GEO databases.
Ethics Approval: This experiment was authorized by the Animal Ethics Committee of Shanghai Outdo Biotech Company, on January 01, 2021. The ethical approval number is YB M-05-02.
Conflicts of Interest: The authors declare that they have no conflicts of interest to report regarding the present study.
References
Andre F, Ismaila N, Allison KH, Barlow WE, Collyar DE et al. (2022). Biomarkers for adjuvant endocrine and chemotherapy in early-stage breast cancer: ASCO guideline update. Journal of Clinical Oncology 40: 1816–1837. https://doi.org/10.1200/JCO.22.00069 [Google Scholar] [PubMed] [CrossRef]
Baggiolini M, Dewald B, Moser B (1997). Human chemokines: An update. Annual Review of Immunology 15: 675–705. https://doi.org/10.1146/annurev.immunol.15.1.675 [Google Scholar] [PubMed] [CrossRef]
Bai Y, Yang Y, Yan Y, Zhong J, Blee AM et al. (2019). RUNX2 overexpression and PTEN haploinsufficiency cooperate to promote CXCR7 expression and cellular trafficking, AKT hyperactivation and prostate tumorigenesis. Theranostics 9: 3459–3475. https://doi.org/10.7150/thno.33292 [Google Scholar] [PubMed] [CrossRef]
Balkwill FR, Capasso M, Hagemann T (2012). The tumor microenvironment at a glance. Journal of Cell Science 125: 5591–5596. https://doi.org/10.1242/jcs.116392 [Google Scholar] [PubMed] [CrossRef]
Barrett T, Wilhite SE, Ledoux P, Evangelista C, Kim IF et al. (2013). NCBI GEO: Archive for functional genomics data sets—Update. Nucleic Acids Research 41: D991–D995. https://doi.org/10.1093/nar/gks1193 [Google Scholar] [PubMed] [CrossRef]
Belinky F, Nativ N, Stelzer G, Zimmerman S, Iny Stein T, Safran M, Lancet D (2015). PathCards: Multi-source consolidation of human biological pathways. Database 2015: bav006. https://doi.org/10.1093/database/bav006 [Google Scholar] [PubMed] [CrossRef]
Britt KL, Cuzick J, Phillips KA (2020). Key steps for effective breast cancer prevention. Nature Reviews, Cancer 20: 417–436. https://doi.org/10.1038/s41568-020-0266-x [Google Scholar] [PubMed] [CrossRef]
Chang L, Karin M (2001). Mammalian MAP kinase signalling cascades. Nature 410: 37–40. https://doi.org/10.1038/35065000 [Google Scholar] [PubMed] [CrossRef]
Chen W, Qin Y, Wang D, Zhou L, Liu Y et al. (2018). CCL20 triggered by chemotherapy hinders the therapeutic efficacy of breast cancer. PLoS Biology 16: e2005869. https://doi.org/10.1371/journal.pbio.2005869 [Google Scholar] [PubMed] [CrossRef]
Ciavarella S, Vegliante MC, Fabbri M, Summa SD, Melle F et al. (2018). Dissection of DLBCL microenvironment provides a gene expression-based predictor of survival applicable to formalin-fixed paraffin-embedded tissue. Annals of Oncology 29: 2363–2370. https://doi.org/10.1093/annonc/mdy450 [Google Scholar] [PubMed] [CrossRef]
Clarke C, Madden SF, Doolan P, Aherne ST, Joyce H et al. (2013). Correlating transcriptional networks to breast cancer survival: A large-scale coexpression analysis. Carcinogenesis 34: 2300–2308. https://doi.org/10.1093/carcin/bgt208 [Google Scholar] [PubMed] [CrossRef]
Di J, Huang H, Qu D, Tang J, Cao W et al. (2015). Rap2B promotes proliferation, migration, and invasion of human breast cancer through calcium-related ERK1/2 signaling pathway. Scientific Reports 5: 12363. https://doi.org/10.1038/srep12363 [Google Scholar] [PubMed] [CrossRef]
Dogan T, Gnad F, Chan J, Phu L, Young A et al. (2017). Role of the E3 ubiquitin ligase RNF157 as a novel downstream effector linking PI3K and MAPK signaling pathways to the cell cycle. The Journal of Biological Chemistry 292: 14311–14324. https://doi.org/10.1074/jbc.M117.792754 [Google Scholar] [PubMed] [CrossRef]
Duffy MJ, Harbeck N, Nap M, Molina R, Nicolini A, Senkus E, Cardoso F (2017). Clinical use of biomarkers in breast cancer: Updated guidelines from the european group on tumor markers (EGTM). European Journal of Cancer 75: 284–298. https://doi.org/10.1016/j.ejca.2017.01.017 [Google Scholar] [PubMed] [CrossRef]
Fulton AM (2009). The chemokine receptors CXCR4 and CXCR3 in cancer. Current Oncology Reports 11: 125–131. https://doi.org/10.1007/s11912-009-0019-1 [Google Scholar] [PubMed] [CrossRef]
Gao J, Aksoy BA, Dogrusoz U, Dresdner G, Gross B et al. (2013). Integrative analysis of complex cancer genomics and clinical profiles using the cBioPortal. Science Signaling 6: pl1. https://doi.org/10.1126/scisignal.2004088 [Google Scholar] [PubMed] [CrossRef]
Guan H, Mao L, Wang J, Wang S, Yang S, Wu H, Sun W, Chen Z, Chen M (2022). Exosomal RNF157 mRNA from prostate cancer cells contributes to M2 macrophage polarization through destabilizing HDAC1. Frontiers in Oncology 12: 1021270. https://doi.org/10.3389/fonc.2022.1021270 [Google Scholar] [PubMed] [CrossRef]
Guo Y, Fan Y, Zhang J, Lomberk GA, Zhou Z et al. (2015). Perhexiline activates KLF14 and reduces atherosclerosis by modulating ApoA-I production. The Journal of Clinical Investigation 125: 3819–3830. https://doi.org/10.1172/JCI79048 [Google Scholar] [PubMed] [CrossRef]
Johnson GL, Lapadat R (2002). Mitogen-activated protein kinase pathways mediated by ERK, JNK, and p38 protein kinases. Science 298: 1911–1912. https://doi.org/10.1126/science.1072682 [Google Scholar] [PubMed] [CrossRef]
Kanemitsu N, Ebisuno Y, Tanaka T, Otani K, Hayasaka H et al. (2005). CXCL13 is an arrest chemokine for B cells in high endothelial venules. Blood 106: 2613–2618. https://doi.org/10.1182/blood-2005-01-0133 [Google Scholar] [PubMed] [CrossRef]
Kong JH, Young CB, Pusapati GV, Patel CB, Ho S et al. (2020). A membrane-tethered ubiquitination pathway regulates hedgehog signaling and heart development. Developmental Cell 55: 432–449. https://doi.org/10.1016/j.devcel.2020.08.012 [Google Scholar] [PubMed] [CrossRef]
Kosacka J, Nowicki M, Paeschke S, Baum P, Blüher M, Klöting N (2018). Up-regulated autophagy: As a protective factor in adipose tissue of WOKW rats with metabolic syndrome. Diabetology & Metabolic Syndrome 10: 13. https://doi.org/10.1186/s13098-018-0317-6 [Google Scholar] [PubMed] [CrossRef]
Lee JV, Housley F, Yau C, Nakagawa R, Winkler J et al. (2022). Combinatorial immunotherapies overcome MYC-driven immune evasion in triple negative breast cancer. Nature Communications 13: 3671. https://doi.org/10.1038/s41467-022-31238-y [Google Scholar] [PubMed] [CrossRef]
Lee SK, Park KK, Kim HJ, Park J, Son SH, Kim KR, Chung WY (2017). Human antigen R-regulated CCL20 contributes to osteolytic breast cancer bone metastasis. Scientific Reports 7: 9610. https://doi.org/10.1038/s41598-017-09040-4 [Google Scholar] [PubMed] [CrossRef]
Ley K (2003). Arrest chemokines. Microcirculation 10: 289–295. https://doi.org/10.1080/mic.10.3-4.289.295 [Google Scholar] [CrossRef]
Li T, Fu J, Zeng Z, Cohen D, Li J, Chen Q, Li B, Liu XS (2020). TIMER2.0 for analysis of tumor-infiltrating immune cells. Nucleic Acids Research 48: W509–W514. https://doi.org/10.1093/nar/gkaa407 [Google Scholar] [PubMed] [CrossRef]
Liao Y, Wang J, Jaehnig EJ, Shi Z, Zhang B (2019). WebGestalt 2019: Gene set analysis toolkit with revamped UIs and APIs. Nucleic Acids Research 47: W199–W205. https://doi.org/10.1093/nar/gkz401 [Google Scholar] [PubMed] [CrossRef]
Lin N, Lin JZ, Tanaka Y, Sun P, Zhou X (2021). Identification and validation of a five-lncRNA signature for predicting survival with targeted drug candidates in ovarian cancer. Bioengineered 12: 3263–3274. https://doi.org/10.1080/21655979.2021.1946632 [Google Scholar] [PubMed] [CrossRef]
Loibl S, Poortmans P, Morrow M, Denkert C, Curigliano G (2021). Breast cancer. Lancet 397: 1750–1769. https://doi.org/10.1016/S0140-6736(20)32381-3 [Google Scholar] [PubMed] [CrossRef]
Matz A, Lee SJ, Schwedhelm-Domeyer N, Zanini D, Holubowska A, Kannan M, Farnworth M, Jahn O, Göpfert MC (2015a). Regulation of neuronal survival and morphology by the E3 ubiquitin ligase RNF157. Cell Death and Differentiation 22: 626–642. https://doi.org/10.1038/cdd.2014.163 [Google Scholar] [PubMed] [CrossRef]
Matz A, Lee SJ, Schwedhelm-Domeyer N, Zanini D, Holubowska A, Kannan M, Farnworth M, Jahn O, Göpfert MC, Stegmüller J (2015b). Regulation of neuronal survival and morphology by the E3 ubiquitin ligase RNF157. Cell Death and Differentiation 22: 626–642. https://doi.org/10.1038/cdd.2014.163 [Google Scholar] [PubMed] [CrossRef]
Pallandre JR, Krzewski K, Bedel R, Ryffel B, Caignard A et al. (2008). Dendritic cell and natural killer cell cross-talk: A pivotal role of CX3CL1 in NK cytoskeleton organization and activation. Blood 112: 4420–4424. https://doi.org/10.1182/blood-2007-12-126888 [Google Scholar] [PubMed] [CrossRef]
Park J, Lee J, Choi C (2015). Evaluation of drug-targetable genes by defining modes of abnormality in gene expression. Scientific Reports 5: 13576. https://doi.org/10.1038/srep13576 [Google Scholar] [PubMed] [CrossRef]
Phua SC, Chiba S, Suzuki M, Su E, Roberson EC et al. (2019). Dynamic remodeling of membrane composition drives cell cycle through primary cilia excision. Cell 178: 261. https://doi.org/10.1016/j.cell.2019.06.015 [Google Scholar] [PubMed] [CrossRef]
Platonova S, Cherfils-Vicini J, Damotte D, Crozet L, Vieillard V et al. (2011). Profound coordinated alterations of intratumoral NK cell phenotype and function in lung carcinoma. Cancer Research 71: 5412–5422. https://doi.org/10.1158/0008-5472.CAN-10-4179 [Google Scholar] [PubMed] [CrossRef]
Punkenburg E, Vogler T, Büttner M, Amann K, Waldner M et al. (2016). Batf-dependent Th17 cells critically regulate IL-23 driven colitis-associated colon cancer. Gut 65: 1139–1150. https://doi.org/10.1136/gutjnl-2014-308227 [Google Scholar] [PubMed] [CrossRef]
Qi T, Jing R, Ma B, Hu C, Wen C, Shao Y, Pei C (2022). The E3 ligase RNF157 inhibits lens epithelial cell apoptosis by negatively regulating p53 in age-related cataracts. Investigative Ophthalmology & Visual Science 63: 11. https://doi.org/10.1167/iovs.63.4.11 [Google Scholar] [PubMed] [CrossRef]
Rajaram M, Li J, Egeblad M, Powers RS (2013). System-wide analysis reveals a complex network of tumor-fibroblast interactions involved in tumorigenicity. PLoS Genetics 9: e1003789. https://doi.org/10.1371/journal.pgen.1003789 [Google Scholar] [PubMed] [CrossRef]
Ramachandran CD, Gholami K, Lam SK, Hoe SZ (2020). A preliminary study of the effect of a high-salt diet on transcriptome dynamics in rat hypothalamic forebrain and brainstem cardiovascular control centers. PeerJ 8: e8528. https://doi.org/10.7717/peerj.8528 [Google Scholar] [PubMed] [CrossRef]
Robitaille K, Daviau A, Lachance G, Couture JP, Blouin R (2008). Calphostin C-induced apoptosis is mediated by a tissue transglutaminase-dependent mechanism involving the DLK/JNK signaling pathway. Cell Death and Differentiation 15: 1522–1531. https://doi.org/10.1038/cdd.2008.77 [Google Scholar] [PubMed] [CrossRef]
Salazar Y, Zheng X, Brunn D, Raifer H, Picard F et al. (2020). Microenvironmental Th9 and Th17 lymphocytes induce metastatic spreading in lung cancer. The Journal of Clinical Investigation 130: 3560–3575. https://doi.org/10.1172/JCI124037 [Google Scholar] [PubMed] [CrossRef]
Seager RJ, Hajal C, Spill F, Kamm RD, Zaman MH (2017). Dynamic interplay between tumour, stroma and immune system can drive or prevent tumour progression. Convergent Science Physical Oncology 3: 034002. https://doi.org/10.1088/2057-1739/aa7e86 [Google Scholar] [PubMed] [CrossRef]
Solinas G, Germano G, Mantovani A, Allavena P (2009). Tumor-associated macrophages (TAM) as major players of the cancer-related inflammation. Journal of Leukocyte Biology 86: 1065–1073. https://doi.org/10.1189/jlb.0609385 [Google Scholar] [PubMed] [CrossRef]
Sung H, Ferlay J, Siegel RL, Laversanne M, Soerjomataram I, Jemal A, Bray F (2021). Global cancer statistics 2020: GLOBOCAN estimates of incidence and mortality worldwide for 36 cancers in 185 countries. CA: A Cancer Journal for Clinicians 71: 209–249. https://doi.org/10.3322/caac.21660 [Google Scholar] [PubMed] [CrossRef]
Thelen M (2001). Dancing to the tune of chemokines. Nature Immunology 2: 129–134. https://doi.org/10.1038/84224 [Google Scholar] [PubMed] [CrossRef]
Tizzano EF (2018). Correlation between SMA type and SMN2 copy number revisited: An analysis of 625 unrelated Spanish patients and a compilation of 2834 reported cases. Neuromuscular Disorders 28: 208–215. https://doi.org/10.1016/j.nmd.2018.01.003 [Google Scholar] [PubMed] [CrossRef]
Wu K, Fukuda K, Xing F, Zhang Y, Sharma S et al. (2015). Roles of the cyclooxygenase 2 matrix metalloproteinase 1 pathway in brain metastasis of breast cancer. The Journal of Biological Chemistry 290: 9842–9854. https://doi.org/10.1074/jbc.M114.602185 [Google Scholar] [PubMed] [CrossRef]
Xu P, Xu S, Pan H, Dai C, Xu Y et al. (2023). Differential effects of the LncRNA RNF157-AS1 on epithelial ovarian cancer cells through suppression of DIRAS3- and ULK1-mediated autophagy. Cell Death & Disease 14: 140. https://doi.org/10.1038/s41419-023-05668-5 [Google Scholar] [PubMed] [CrossRef]
Ye F, Gao H, Xiao L, Zuo Z, Liu Y et al. (2019). Whole exome and target sequencing identifies MAP2K5 as novel susceptibility gene for familial non-medullary thyroid carcinoma. International Journal of Cancer 144: 1321–1330. https://doi.org/10.1002/ijc.31825 [Google Scholar] [PubMed] [CrossRef]
Yu G, Wang LG, Han Y, He QY (2012). clusterProfiler: An R package for comparing biological themes among gene clusters. Omics 16: 284–287. https://doi.org/10.1089/omi.2011.0118 [Google Scholar] [PubMed] [CrossRef]
Zhang Z, Chen W, Luo C, Zhang W (2022). Exploring a four-gene risk model based on doxorubicin resistance-associated lncRNAs in hepatocellular carcinoma. Frontiers in Pharmacology 13: 1015842. https://doi.org/10.3389/fphar.2022.1015842 [Google Scholar] [PubMed] [CrossRef]
Zhang Y, Zhang Z (2020). The history and advances in cancer immunotherapy: Understanding the characteristics of tumor-infiltrating immune cells and their therapeutic implications. Cellular & Molecular Immunology 17: 807–821. https://doi.org/10.1038/s41423-020-0488-6 [Google Scholar] [PubMed] [CrossRef]
Zhao H, Wu L, Yan G, Chen Y, Zhou M, Wu Y, Li YJ (2021). Inflammation and tumor progression: Signaling pathways and targeted intervention. Signal Transduction and Targeted Therapy 6: 263. https://doi.org/10.1038/s41392-021-00658-5 [Google Scholar] [PubMed] [CrossRef]
Zhou G, Bao ZQ, Dixon JE (1995). Components of a new human protein kinase signal transduction pathway. The Journal of Biological Chemistry 270: 12665–12669. https://doi.org/10.1074/jbc.270.21.12665 [Google Scholar] [PubMed] [CrossRef]
Zhou C, Nitschke AM, Xiong W, Zhang Q, Tang Y et al. (2008). Proteomic analysis of tumor necrosis factor-alpha resistant human breast cancer cells reveals a MEK5/Erk5-mediated epithelial-mesenchymal transition phenotype. Breast Cancer Research 10: R105. https://doi.org/10.1186/bcr2210 [Google Scholar] [PubMed] [CrossRef]
Supplementary Materials
Figure S1: DNA methylation and mutational features of RNF157 in breast cancer from the the Cancer Genome Atlas (TCGA) database. The DNA methylation level of RNF157 in breast cancer. The data were obtained from the UALCAN database. ***p < 0.001.
Cite This Article
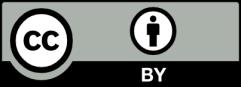