Open Access
ARTICLE
Bioinformatic analysis and in vivo validation of angiogenesis related genes in inflammatory bowel disease
Department of General Surgery, The First Affiliated Hospital of Xi’an Jiaotong University, Xi’an, 710061, China
* Corresponding Authors: JUNHUI YU. Email: ; JIANBAO ZHENG. Email:
(This article belongs to the Special Issue: Bioinformatics Study of Diseases)
BIOCELL 2023, 47(12), 2735-2745. https://doi.org/10.32604/biocell.2023.043422
Received 02 July 2023; Accepted 27 September 2023; Issue published 27 December 2023
Abstract
Objectives: Angiogenesis plays a significant role in the occurrence and development of inflammatory bowel disease (IBD). The aim of this study is to explore potential angiogenesis related genes (ARGs) in IBD through bioinformatics analysis and in vivo experiments. Methods: GSE57945, GSE87466, and GSE36807 were obtained from the Gene Expression Omnibus database. GSE57945 was used as the training set, while GSE87466 and GSE36807 were used as the validation set. The key ARGs associated with IBD were identified using the least absolute shrinkage and selection operator (LASSO) and random forest methods. These identified ARGs were then utilized to construct a diagnostic model for IBD. The Single-Sample Genome Enrichment Analysis, Cibersort, and Xcell methods were used to evaluate the immune infiltration. Expression of amyloid beta precursor protein (APP) was verified in the IBD mouse model induced by dextran sulfate sodium using immunohistochemistry (IHC). Results: The receiver operating curve area of GSE57945 was 0.948. Two distinct clusters were identified using consensus clustering and non-negative matrix factorization clustering. Subsequent analyses revealed significant differences in immune levels and functional enrichment between the two clusters. The successful construction of the animal model for the IBD was evident by hematoxylin and eosin staining, while IHC results showed a high expression of APP in IBD and a low expression in normal tissues. Conclusion: Our findings provide new insights into the diagnosis of IBD by ARGs, and APP could be a potential novel biomarker for IBD and promising therapeutic targets.Keywords
Supplementary Material
Supplementary Material FileInflammatory bowel disease (IBD) is characterized by chronic and recurrent inflammation of the digestive system, leading to immune-mediated tissue damage and impaired intestinal barrier function. Crohn's disease can affect any segment of the digestive tract, with the ability to penetrate the intestinal wall, whereas Ulcerative Colitis (UC) is limited to the colonic mucosa. Research suggests that genetic factors and gastrointestinal immune disorders are the primary contributors to the development of IBD (Rana et al., 2022).
Angiogenesis is the process of formation of new capillaries and generation of new blood vessels from the existing ones. The process is primarily mediated through two mechanisms: budding formation and sprouting. Growth factors, interleukins (ILs), and other cytokines play crucial roles in regulating angiogenesis, along with their involvement in signal transduction pathways (Alkim et al., 2015). Angiogenesis plays a role not only in cancer diseases, but also in non-neoplastic diseases, especially autoimmune diseases, such as gastric ulcer and Alzheimer’s disease (AD) (Costa et al., 2007).
IBD, being an autoimmune inflammatory disorder, often presents varying degrees of ulcerative lesions and regenerative processes. Angiogenesis plays a crucial role in repairing the sites of inflammation in IBD, highlighting its close association with the disease (Alkim et al., 2015). Associated studies have demonstrated that the lesion site of IBD, has more abundant neovascularization (Alkim et al., 2009), which is also confirmed by more intuitive narrowband endoscopic imaging (Danese, 2010).
In this study, the association of ARGs with IBD was examined at the transcriptomic level, and provides a deeper understanding of the pathogenesis, diagnosis, and immune microenvironment of IBD. Then, combined with the diagnostic model and predictive ability, amyloid beta precursor protein (APP) was screened for experimental verification.
The increased deposition of amyloid-like proteins is a major pathological feature of AD, and the APP gene plays a crucial role in this process. Transcriptomic analysis provides evidence of a strong association between AD and IBD (Maden and Acuner, 2021). While the APP gene is a well-known hallmark gene in AD, its presence in IBD does not imply a sole correlation between IBD and AD. Research suggests intricate connections between IBD and degenerative diseases represented by AD. For instance, triggering receptors expressed on myeloid cells (TREM-1/2) expressed on bone marrow cells has been confirmed as a common participant in both IBD and neurodegenerative diseases (Natale et al., 2019). Furthermore, as part of the amyloid precursor protein family, the APP gene plays a role in mutually influencing the expression of other amyloid-like proteins. APP has been established to have connections with α-synuclein, a characteristic protein associated with Parkinson’s disease (PD) (Cheng et al., 2023). This highlights the intricate interplay between different molecular pathways implicated in various neurodegenerative conditions. Indeed, while connections exist between IBD and degenerative diseases such as AD and PD, it is clear that the APP gene primarily holds a more substantial role in AD. As a result, this study specifically concentrates on the APP gene within the context of ARGs. IHC analysis of APP expression is carried out to provide insights into the potential relationship between IBD and AD from an angiogenesis perspective.
Our findings have significant implications for the diagnosis of patients with IBD and can potentially guide the development of novel targeted treatment strategies for IBD. This study holds the potential to uncover the causal relationship between IBD and AD.
Datasets GSE57945, GSE87466, and GSE36807 were obtained from the Gene Expression Omnibus (GEO) database. We chose the larger cohort of cases from GSE57945 as our training set, to ensure robust and reliable outcomes. Following this, we utilized the independent datasets GSE87466 and GSE36807 for validation purposes. For detailed information regarding these datasets, please refer to Table 1. MSigDB database was used to obtain 36 genes related to angiogenesis.
Construction of diagnostic model
Random forest algorithm and Meandegreasegini index were used to select the genes with significant influence on IBD from 36 genes related to angiogenesis. The least absolute shrinkage and selection operator (LASSO) technique was then used to train the model, and the candidate genes with significant effects on IBD were included in the model to obtain key genes associated with IBD. The risk scoring formula derived from the LASSO analysis is as follows:
Identification of molecular subtypes
The R package “ConsensusClusterPlus” was used to classify and verify samples. In order to verify the accuracy of consistent clustering, the non-negative matrix factorization (NMF) method is used. We chose the standard “brunet” option and went through 100 iterations. We set k clusters between 2 to 5, and the minimum member of the subclass was set to be 10.
Identification and association of immune infiltrating cells in disease
Exploring the composition of the immune microenvironment will help us better understand the occurrence and treatment of diseases. The Single-Sample Genome Enrichment Analysis (ssGSEA) algorithm is used to quantify the composition of 28 immune cells in the disease microenvironment. We used the “CIBERSORT” package to calculate the 22 immune cell types in the dataset for patients with different immune patterns and used boxplots to show the immune cell composition of patients with different immune patterns. As an approach based on gene expression characteristics, xCell is able to describe the enrichment of 64 immune and stromal cell types which assists in better understanding the cellular heterogeneous landscape of human tissue expression profiles.
Functional enrichment analysis of differentially expressed genes in different immune models
We used R package “limma” to screen for differentially expressed genes in subgroups. Differentially expressed genes with log2FC > 1 and adj. p < 0.05 were up-regulated, while differentially expressed genes with log2FC < −1 and adj. p < 0.05 were down-regulated. Based on patient gene expression profiles, we used GSEA to explore differences in biological processes between subgroups. Genome “c2. Cp. KEGG. Symbols. GMT” and “c5. Go. Version. Symbols. GMT” were obtained from the MSigDB database.
A total of 10 C57BL/6 male mice (8–9 weeks of age) (GemPharmatech, Nanjing, China) were placed together in one cage at 22/21°C for 12 h in a light/dark cycle. Each group of five mice was randomly assigned to: (1) the control group, receiving routine drinking water; (2) the treatment group, receiving 3% dextran sulfate sodium (DSS; MP Biomedicals, Shanghai, China) dissolved in high-pressure water and injected into the beverage bottle of the feeding cage. Each mouse was given 5 mL of DSS solution daily. On day 14, the mice were humanely sacrificed by cervical dislocation, and their colon tissue was obtained. All experiments were conducted in accordance with the animal experiment protocol described by Xi’an Jiaotong University.
Hematoxylin and eosin (H&E) staining and immunohistochemistry
The mice were sacrificed by cervical vertebrae dislocation method, and the cecum, colon, and anus of the mice were collected. After dissection, and H&E staining was performed on the obtained samples after fixation, dehydration, paraffin embedding. Paraffin-embedded tissue sections were dewaxed and heated in a pressure cooker. The endogenous peroxidase activity was inhibited by 3% hydrogen peroxide solution. Sections were sealed in 3% bovine serum albumin at room temperature for 30 min and incubated overnight with monoclonal mouse anti-APP IgG (Servicebio, Wuhan, China, diluted 1:200) at 4°C. After incubation with secondary antibody, chromogenic reaction was performed with DABkit (Servicebio, Wuhan, China). Subsequently, quantitative analysis of immunohistochemistry for the treatment and control groups was performed using the IHC-Toolbox plugin in ImageJ (National Institutes of Health, Maryland, USA) (Shu et al., 2016).
All data were processed and calculated in R Studio (Posit Software, Boston, USA). Independent sample t-test was used to compare normally distributed continuous variables, and Wilcoxon test was used to compare non-normally distributed continuous variables. Spearman rank correlation was used to analyze the correlation of two continuous variables. All statistical p-values were two-sided, and those <0.05 were considered to have significant differences.
Expression of angiogenesis related genes in inflammatory bowel disease
The research process is shown in Fig. 1. First, the distribution of 36 ARGs between healthy subjects and patients with IBD were explored. In the GSE57945 data set, the distribution of 30 ARGs was statistically different between healthy people and IBD patients (Suppl. Fig. S1A), which initially indicated that ARGs played a role in the development of IBD. To verify the authenticity of the distribution, we performed the same exploration in GSE87466, the results of which demonstrated that most genes still had statistically significant distributions (Suppl. Fig. S1B), supporting this conclusion.
Figure 1: Flow chart.
Construct angiogenesis related genes diagnostic model
The random forest algorithm was used to identify 15 genes with significant influence on IBD from 36 ARGs (Meandegreasegini > 0, Suppl. Fig. S2). Subsequently, the LASSO algorithm was used for regression analysis to construct the model (Figs. 2A and 2B). A total of 13 genes were selected to construct the model (Fig. 2A), and the corresponding coefficients are shown below: CXCL6 × (−1.588) + SERPINA5 × 0.831 + SLCO2A1 × (−0.580) + FSTL1 × 3.538 + COL3A1 × (−0.268) + TNFRSF21 × 1.409 + PDGFA × 1.464 + TIMP1 × (−0.865) + NRP1 × 0.014 + JAG1 × (−1.663) + POSTN × (−0.490) + VTN × (−0.024) + APP × (−1.221). According to the receiver operating curve (ROC) curve the area under the curve (AUC) was 0.944 (Fig. 2C). Similarly, we also tested the predictive power of 13 genes individually (Suppl. Fig. S3). In order to prove the predictability of the model, we verified the accuracy of our model in GSE87466 (Fig. 2D) and GSE36807 (Fig. 2E) with AUC values of 0.857 and 0.898, which indicated that the accuracy and specificity of our model were relatively high.
Figure 2: Construct angiogenesis related genes (ARGs) diagnostic model (A and B) Gene signatures were screened from ARGs with Meandegreasegini > 0 using the least absolute shrinkage and selection operator algorithm; (C) Receiver operating curve (ROC) of inflammatory bowel disease (IBD) diagnostic and predictive risk score in GSE57945; (D) ROC of IBD diagnostic and predictive risk score in GSE87466; (E) ROC of IBD diagnostic predictive risk score in GSE36807.
Correlation of angiogenesis related genes signature
We examined the functional correlation between 13 marker genes at the gene expression level. In the normal group (Suppl. Fig. S4A), FSTL1 was positively correlated with other genes except PDGFA. TIMP1 was negatively correlated with other genes except SERPINA5 and VTN, while VTN was negatively correlated with other genes except SERPINA5 and TIMP1. In the IBD group (Suppl. Fig. S4B), the correlation between COL3A1 and FSTL1 reached above 0.9. PDGFA was negatively correlated with other genes except JAG1. There were obvious differences between the normal group and the IBD group: TIMP1 was negatively correlated with most genes in the normal group, while positively correlated with most genes in the IBD group; on the contrary, SERPIN5 was negatively correlated with most genes in the IBD group from positively correlated with most genes in the normal group and negatively correlated with most genes in the IBD group. The negative correlation between APP and TIMP1 was weakened and a weak positive correlation was found in the IBD group.
Immune characteristics of angiogenesis related genes subtypes
The progression of IBD is closely related to changes in the immune microenvironment. In order to explore the immune characteristics under the ARGS model, we identified two types (Cluster 1 and Cluster 2) using Consensus Cluster analysis (Figs. 3A–3C). Concurrently, we used the NMF clustering method (Suppl. Fig. S5) to further confirm whether it is reliable to divide the samples into two types. Cluster 1 has 109 samples and Cluster 2 has 145 samples. A boxplot (Fig. 4A) was created to show the expression differences of the 13 ARGS in Cluster 1 and Cluster 2: except JAG1 and SERPINA5, the expression of the other genes showed statistically significant differences. Expressions of CXCL6, SLCO2A1, FSTL1, COL3A1 TNFRSF21, TIMP1, POSTN, and APP in Cluster 1 were higher, while PDGFA and VTN were higher in Cluster 2.
Figure 3: Construction of ARGs-subtypes (A–C) Consistent clustering of samples based on 13 gene signatures.
Figure 4: Immune characteristics of angiogenesis related genes (ARGs)-subtypes. (A) Expression of 13 gene signatures in cluster 1 and cluster 2: Horizontal axis represents genes, vertical axis represents gene expression levels (ns p ≥ 0.05, **p < 0.01, ***p < 0.001); (B) Histogram of immune cell content calculated by ssGSEA: horizontal axis represents 28 immune cells, vertical axis represents cell content; Blue represents Cluster 1 and Red represents Cluster 2.
In this study, the ssGSEA algorithm was used to quantify the immune microenvironment: there was no significant difference in activated CD8 T cell and memory B cell between Cluster 1 and Cluster 2 except activated B cell, and the rest of other immune cells was statistically different (Fig. 4B). There are a large number of significantly enriched immune cells in Cluster 1, especially dendritic cell, macrophage, mast cells, myeloid-derived suppressor cells (MDSC), neutrophils, regulatory T cells, macrophage T helper cells, and other inflammatory cells. We then proceeded to verify the immune differences between Cluster 1 and Cluster 2 using Xcell (Suppl. Fig. S6A) and cibersort (Suppl. Figs. S6B and S6C) algorithms. Consistent with the results of ssGSEA, both Cibersort and Xcell showed that Cluster 1 had more obvious immune cell enrichment. Notably, Cibersort showed that M1 and M0 macrophages were significantly increased in Cluster 1, while M2 macrophages were significantly increased in Cluster 2. Similarly, activated mast cells were significantly increased in Cluster 1, while resting mast cells were significantly increased in Cluster 2. In Xcell, the immune score, stroma score, and microenvironment score of Cluster 1 were higher than those of Cluster 2.
Correlation angiogenesis related genes signature with immune cells
Spearman correlation calculation was used to explore the role of 13 maker genes in immune microenvironment in ssGSEA (Fig. 5A). PDGFA was negatively correlated with all immune cells, especially with activated dendritic cell, eosinophils and type 1 helper T cell (Figs. 5B–5D). Except for the weak positive correlation between jagged 1 (JAG1) and central memory CD8 T cell and eosinophils, JAG1 was negatively correlated with the remaining immune cells. FSTL1 was positively correlated with all immune cells, especially with natural killer (NK) cells and plasmacytoid dendritic cells (Figs. 5E and 5F). Similarly, TIMP1 is positively correlated with all immune cells, and macrophage, MDSC, and regulatory T cell are particularly well correlated (Figs. 5G–5I). Except for the weak negative correlation between CXCL6 and memory B cells, CXCL6 was positively correlated with all other immune cells, for instance, the correlation between CXCL6 and immature dendritic cells reached a value of 0.89 (Fig. 5J). The special relationship between PDGFA and TIMP1 with immune cells is also reflected in the above discussion: PDGFA, FSTL1, and TIMP1 showed significant negative correlation in both normal patients and IBD groups. Both JAG1 and TIMP1 show an obvious negative correlation. The above results have also been verified in Cibersort, Xcell. It Cibersort, PDGFA, and macrophages_M2 have a positive correlation, which is significantly different from other algorithms. In the above mentioned study, cibersort showed that macrophages_M2 is obviously enriched in Cluster 2, indicating a possible important role of platelet-derived growth factor subunit A (PDGFA) in Cluster 2.
Figure 5: Correlation of angiogenesis related genes (ARGs) signature with immune cells (A) Correlation between 13 gene signatures and immune cells, red indicates negative correlation; blue indicates positive correlation; (B–D) The correlation between PDGFA and immune cells ((B) Activated dendritic cell; (C) Eosinophil; (D) Type 1 T helper cell). (E and F) The correlation between FSTL1 and immune cells ((E) Natural killer cell, (F) Plasmacytoid dendritic cells); (G and I) The correlation between TIMP1 and immune cells ((G) Macrophage; (H) MDSC; (I) Regulatory T cells); (J) Correlation between CXCL6 and immature dendritic cells.
Differential analysis of the two immune models
In this study, “limma” package was used to obtain 653 DEGs in Cluster 1 and Cluster 2. In the Cluster 1 group, 329 DEGs were up-regulated and 324 DEGs were down-regulated. In GSEA-GO (Fig. 6A), regulation of IL−6 production, cell activation involved in immune response, regulation of programmed cell death, and chemokine activity is suppressed; however, many molecular metabolic processes such as carboxylic acids and organic acids are activated. In GSEA-Kyoto Encyclopedia of Genes and Genomes (KEGG) (Fig. 6B), many intracellular cascade signaling pathways, including toll-like receptors, nod-like receptors, and IL-17 signals were inhibited; instead, the activated pathways are concentrated in compound metabolisms, such as carcinogenic-DNA adducts, drug metabolism, and steroid hormones.
Figure 6: Difference analysis of the two immune models. (A and B) Gene Set Enrichment Analysis (GSEA) analysis of differentially expressed genes (DEGs) ((A) GSEA-Gene Ontology (GO) analysis: horizontal coordinate is gene ratio; The ordinate represents the GO term; Color indicator -log10 (p-value); Node size represents the number of genes enriched in GO terms. (B) GSEA-Kyoto Encyclopedia of Genes and Genomes (KEGG): abscissa is the gene ratio; The ordinate represents the KEGG term; Node size represents the number of genes enriched in the path. Node color indicates -log10 (p-value).
Validation of amyloid beta precursor protein expression in the mouse model of colitis
To better understand whether the expression of signature genes is actually increased in IBD, the mouse model of colitis was established. Microscopic observation in the mice control group showed that the intestinal gland epithelial cells, and the intestinal villi were closely arranged, with no inflammation observed overall (Fig. 7A). The whole tissue of mice treated with DSS underwent severe destruction. Visible to the naked eye, the crypt structure was partially lost, intestinal villi were partially shed, and inflammation was obvious (Fig. 7B). These findings were evident in confirming the success of model construction. According to previous bioinformatical analysis, among the genes with predictive ability (AUC) > 0.65, CXCL6, COLA3A1, and TIMP1 genes have considerable experimental support for their function in IBD. APP has a relatively high predictive ability (AUC = 0.685), but few experiments support the value of APP in IBD; therefore, in this study, APP gene was selected for experimental verification of the diagnostic model. We used the above tissue sections to detect the expression of APP by immunohistochemistry. The results showed that the expression of APP in the colitis tissue was significantly higher than that in the normal tissue (p < 0.01), which was consistent with our results (Figs. 7C–7E).
Figure 7: Validation of amyloid beta precursor protein (APP) expression in the mouse model of colitis (scale bars: 100 and 50 µm) (A) Hematoxylin and Eosin (HE) staining of the colon of mice in the control group; (B) HE staining of the colon in the treatment group; (C) APP immunohistochemistry of the colon in the treatment group; (D) APP immunohistochemistry of the colon in the control group; (E) Histogram of APP immunohistochemistry in treatment group and control group, with the vertical axis representing APP positive cells/field (**p < 0.01) (3% dextran sulfate sodium (DSS) group: N = 5; Control group: N = 5).
The prevalence of IBD, including CD and ulcerative colitis, is expected to increase in 1% by 2030 due to changes in living conditions and environment (Kaplan and Windsor, 2021). Whether it is neovascularization or the formation of new blood vessels based on the original blood vessels, angiogenesis is very important for the occurrence, metastasis, and prognosis of tumors (Carmeliet, 2005). Vascular endothelial growth factors (VEGFs) have an impact on angiogenesis by affecting the corresponding pathways, thus affecting the disease (Wan et al., 2021). However, the clinical correlation between ARGs and IBD has not been fully elucidated.
In this study, we established a diagnostic model that predicts the prevalence of IBD patients based on characteristics of ARGs and validated its accuracy on an independent validation dataset. ARGs score and associated diagnostic model can preliminarily predict the prevalence of patients and immune characteristics of IBD patients. In addition, we also obtained differential genes from established diagnostic models to further explore the unique biological characteristics of IBD.
We identified 13 ARGs related to IBD diagnosis, including CXCL6, FSTL1, PDGFA, and VTN. PDGFA is a protein-coding gene, and an important paralog of this gene is PDGFB. In one previous study (Rana et al., 2022), the interaction of gasdermin B with PDGFA affected regeneration and repair of IBD epithelium through programmed cell death. Follistatin-like 1 (FSTL1), a secreted glycoprotein, is involved in various physiological processes, such as angiogenesis, immune response regulation, cell proliferation and differentiation, stimulates innate immune responses via CD14 and toll-like receptors (Murakami et al., 2012). In a Genome-Wide Association Study, sequencing study (Garcia-Etxebarria et al., 2022), FSTL1 was shown to have a clear correlation with IBD. Interestingly, in human aortic smooth muscle cells cultured in vitro (Miyabe et al., 2014), FSTL1 can inhibit the cell migration and proliferation process of PDFG-BB and reduce endocardial thickening after intimal injury. The correlation between FSTL1 and PDGFA is reflected in the correlation of gene expression in normal tissues of patients with IBD. In patients with growth hormone deficiency (Galimov et al., 2015) and acute myeloid leukemia with FTL3 mutation (Chen et al., 2020), both FSTL1 and COL3A1 have been identified as key genes affecting the development of the disease. Similarly, this study also observed a strong synergistic effect of FSTL1 and COL3A1 in IBD patients, suggesting that FSTL1 and COL3A1 may be related in patients with IBD. TIMP1 belongs to the TIMP gene family, which encodes proteins that can promote cell proliferation in various cell types and may have anti-apoptotic functions. Studies have shown (Albini et al., 2021) that TIMP1 is expressed more frequently in CD patients with colorectal cancer (CRC) development than in CD patients without CRC. In this study, the expression of TIMP1 was significantly different from the 12 genes identified in normal tissues and tissues of patients with IBD, which further proved that the genes we screened played a non-negligible role in the occurrence and development of IBD.
In this study, Consistent clustering and NMF clustering methods were used to divide the samples into two groups: Cluster 1 and Cluster 2. Then we used ssGSEA, cibersort and Xcell algorithm to analyze the difference of immune infiltration between the two groups. Macrophages and neutrophils are the most important gatekeepers of intestinal immune homeostasis and innate immunity against foreign pathogens (Pan et al., 2022). Macrophages and neutrophils in Cluster 1 were significantly higher than those in Cluster2. In addition, we found a large number of immune cells in Cluster 1, especially those expressing dendritic cells, mast cells, MDSC, NK T cells, regulatory T cells, T helper cells, and other inflammatory cells. We suggest Cluster 1 as “immune hot” type, which was also proved by Cibersort and Xcell. Interestingly, in Cibersort, macrophage M1 and M0 types were significantly increased in Cluster 1, while macrophage M2 type was significantly increased in Cluster 2. According to different functions, macrophages can be categorized into M1 and M2 macrophages (Shapouri Moghaddam et al., 2018). M1 macrophage is considered to be a proinflammatory subtype, leading to stimulating inflammation, tissue injury, and aggravating epithelial injury (Pan et al., 2022). M2 macrophages repair damaged tissue by promoting angiogenesis and phagocytic debris (Braga et al., 2015). This difference in the distribution of macrophages further supports the ability of the 13 marker genes we identified to distinguish patients with IBD from healthy subjects.
To better understand the influence of gene signatures on the immune environment, we continued to investigate the association between genes and immune cells. Immune cell types in head and neck squamous cell carcinoma are regulated by PDGFA (Duhen et al., 2021), and in this study, PDGFA is also closely associated with immune cells in IBD. As a cell fate decision factor during hematopoiesis (Li et al., 1998), JAG1 has been shown to promote inflammatory leukocyte recruitment through the Notch pathway in atherosclerotic lesions (Nus et al., 2016). In cell culture, tissue inhibitor of metalloproteinases 1 (TIMP1) has a potential role in controlling the polarization of NK cells induced by tumor-related cytokine TGFβ (Altadill et al., 2021). On the other hand, TIMP1 was found to promote the immune response in the lungs after influenza infection and promote the injurious phenotype caused by influenza infection (Allen et al., 2018). CD8 T cells, neutrophils, and other immune cells are affected by FSTL1 (Li et al., 2020a), resulting in the disorder of systemic immune status and promoting tumor metastasis (Kudo-Saito, 2013). The role of chemokines in angiogenesis has not been fully elucidated. SR-PSOX/CXCL16, as a scavenger receptor, is involved in bacterial antigen phagocytosis and Th1 immune response by producing IL-12 and interferon-γ in patients with IBD (Uza et al., 2011). However, studies have shown that neutrophils can be activated by CXC chemokine ligand 6 (CXCL6) to change the state of the tumor cell environment, further affecting the development of the disease (Gijsbers et al., 2005).
In the GSEA analyses, the DEGs were enriched in the IL−17 signaling pathway, cytokine activity, neutrophil-mediated immunity, neutrophil activation, leukocyte chemotaxis and Cytokine−cytokine receptor interaction, and tumor necrosis factor (TNF) signaling pathway. In fact, the hallmark of active IBD is neutrophil infiltration in IBD intestinal tissue (Li et al., 2020b). Neutrophils have a strong chemotaxis, so they are the first inflammatory cells to reach the focal area (Kolaczkowska and Kubes, 2013). CD177 neutrophils, as an important subgroup of neutrophils, play an important role in protecting the gastrointestinal immune environment, and their deficiency leads to impaired intestinal barrier function (Zhou et al., 2018). More studies have pointed out that the neutrophil-lymphocyte ratio may be a promising biomarker of IBD (Langley et al., 2021). IL-17 affects T cell differentiation by affecting the NF-κB signaling pathway and induces the generation of more Th17 cells, thus influencing the immune environment (Blauvelt and Chiricozzi, 2018). The mucous membranes of the gastrointestinal tract play an important role in immunity. Th17 cells can affect the permeability of the mucous membranes, resulting in dysregulation of the immune response (McGeachy et al., 2019). In addition to neutrophils and IL17, the role of chemokines and TNF in IBD cannot be overlooked. In the intestine, different intestinal sites show different chemokine expression patterns, which contribute to the division of leukocyte recruitment and thereby regulate regional immunity (Trivedi and Adams, 2018). The CCR6-CCL20 axis and CCR9-CCL25 axis are important for intestinal homeostasis in IBD, and their functional status affects the inflammatory load and thus increases the risk of colon cancer in colitis (Meitei et al., 2021).
While the AUC values of CXCL6, COL3A1, and TIMP1 may be higher than that of APP, it is worth noting that the involvement of these three genes in the development of IBD has already been established. Therefore, to further validate the scientific validity of our diagnostic model, we specifically selected the APP gene for verification as a key gene. This selection is based on the potential of APP as a novel biomarker for IBD and its potential relevance in the pathogenesis and treatment of the disease. In this study, IHC was used to detect the expression levels of key gene-APP in IBD and normal bowel tissues. The results showed that the trend of APP expression between patients with IBD and normal tissues was consistent with our results: APP expression increased in tissues of patients with IBD, thus indicating the reliability of our diagnostic model.
APP plays an important role in AD (Maden and Acuner, 2021), which can activate microglia cells in the brain and cause inflammation (Akiyama, 1994). Existing studies have demonstrated the association between AD and IBD (Maden and Acuner, 2021). Notably, KEGG and immune infiltration analysis revealed a substantial presence of neutrophils in the lesions of both IBD and AD. The role of neutrophils as a bridge between the two diseases has drawn attention. Studies using mouse models have confirmed that increased neutrophils caused by acute intestinal inflammation can also lead to the aggregation of neutrophils in the brain, thus promoting the occurrence of AD (Kaneko et al., 2023). However, this phenomenon has not been observed in chronic intestinal inflammation. Matrix metalloproteinase 9 (MMP-9) has been found to be highly expressed in glial cells of AD mouse models (Yan et al., 2006), and researchers have also discovered increased neutrophils in the brain enhance MMP-9 activity, resulting in elevated levels of amyloid-like proteins and an increased risk of AD (Kaneko et al., 2023). Similar findings have been observed in clinical practice. As a therapeutic medication for IBD, thiopurine is an inhibitor of Ras-related C3 botulinum toxin substrate 1 (Rac1) (Wang et al., 2009), which can lead to an increase in APP (Borin et al., 2018). Clinical cohort studies have revealed a lower incidence of AD in IBD patients treated with thiopurine, indicating the continued importance of APP in this context (Sutton et al., 2019).
This study represents our initial endeavor to employ a bioinformatics approach for identifying ARGs in IBD. Bioinformatics and artificial intelligence have become pivotal in the realm of IBD research. These technologies facilitate personalized treatment strategies, novel drug development, and numerous other facets of IBD research (Agrawal et al., 2022). Moreover, they open new vistas in areas like pathological image analysis, prediction modeling, and preventive measures, propelling groundbreaking progress in IBD diagnosis, treatment, and management (Stidham and Takenaka, 2022). Utilizing various bioinformatics methods, we successfully identified 13 potential diagnostic biomarkers. Furthermore, the IHC results validated the significance of APP as not only a potential novel biomarker for IBD but also a promising therapeutic target. However, further experiments, including clinical data validation, are necessary to confirm the value of these 13 genes in the context of IBD. Building upon previous studies, our findings contribute to a deeper understanding of the relationship between angiogenesis and IBD. This knowledge holds potential implications for the diagnosis and treatment of IBD.
In this study, bioinformatics methods were employed to investigate the relationship between ARGs and IBD, as well as its associated immune microenvironment. IHC was conducted in a mouse model of colitis, confirming the elevated expression of APP. Although the primary function of APP is to contribute to the formation of amyloid-like proteins, its role in angiogenesis may also be significant in AD and IBD. These findings suggest that APP has the potential to serve as a biomarker for the treatment of IBD.
Acknowledgement: The authors declare no acknowledgment.
Funding Statement: This work was funded by the Nanjing Tianqing Research Fund Project (Grant Serial Number: HX202334), and the Institute Fund from First Affliated Hospital of Xi’an Jiaotong University (Grant Serial Number: 2022MS-17).
Author Contributions: Conceptualization: Jian-Bao Zheng, Jun-Hui Yu, and Xue-Jun Sun; Methodology: Chen-Ye Zhao; Writing—original draft preparation: Ze-Peng Dong, and Shi-Bo Hu; Writing—review and editing: Kui Yang. All authors have read and agreed to the published version of the manuscript.
Availability of Data and Materials: GSE57945, GSE87466, and GSE36807 were obtained from the Gene Expression Omnibus (GEO) database (https://www.ncbi.nlm.nih.gov/geo/), further inquiries can be directed to the corresponding authors.
Ethics Approval: The experimental protocols were evaluated and approved by the Animal Care and Use Committee of Xi’an Jiaotong University, China (XJTUAE2023-1577).
Conflicts of Interest: The authors declare that they have no conflicts of interest to report regarding the present study.
Supplementary Materials: The supplementary material is available online at https://doi.org/10.32604/biocell.2023.043422.
References
Agrawal M, Allin KH, Petralia F, Colombel JF, Jess T (2022). Multiomics to elucidate inflammatory bowel disease risk factors and pathways. Nature Reviews. Gastroenterology & Hepatology 19: 399–409. https://doi.org/10.1038/s41575-022-00593-y [Google Scholar] [PubMed] [CrossRef]
Akiyama H (1994). Inflammatory response in alzheimer's disease. The Tohoku Journal of Experimental Medicine 174: 295–303. https://doi.org/10.1620/tjem.174.295 [Google Scholar] [PubMed] [CrossRef]
Albini A, Gallazzi M, Palano MT, Carlini V, Ricotta R, Bruno A, Stetler-Stevenson WG, Noonan DM (2021). TIMP1 and TIMP2 downregulate TGFβ induced decidual-like phenotype in natural killer cells. Cancers 13: 4955. https://doi.org/10.3390/cancers13194955 [Google Scholar] [PubMed] [CrossRef]
Alkim C, Alkim H, Koksal AR, Boga S, Sen I (2015). Angiogenesis in inflammatory bowel disease. International Journal of Inflammation 2015: 1–10. https://doi.org/10.1155/2015/970890 [Google Scholar] [PubMed] [CrossRef]
Alkim C, Savas B, Ensari A, Alkim H, Dagli U, Parlak E, Ulker A, Sahin B (2009). Expression of p53, VEGF, microvessel density, and cyclin-d1 in noncancerous tissue of inflammatory bowel disease. Digestive Diseases and Sciences 54: 1979–1984. https://doi.org/10.1007/s10620-008-0554-x [Google Scholar] [PubMed] [CrossRef]
Allen JR, Ge L, Huang Y, Brauer R, Parimon T, Cassel SL, Sutterwala FS, Chen P (2018). TIMP-1 promotes the immune response in influenza-induced acute lung injury. Lung 196: 737–743. https://doi.org/10.1007/s00408-018-0154-2 [Google Scholar] [PubMed] [CrossRef]
Altadill A, Eiro N, González LO, Andicoechea A, Fernández-Francos S, Rodrigo L, García-Muñiz JL, Vizoso FJ (2021). Relationship between metalloprotease-7 and -14 and tissue inhibitor of metalloprotease 1 expression by mucosal stromal cells and colorectal cancer development in inflammatory bowel disease. Biomedicines 9: 495. https://doi.org/10.3390/biomedicines9050495 [Google Scholar] [PubMed] [CrossRef]
Blauvelt A, Chiricozzi A (2018). The immunologic role of IL-17 in psoriasis and psoriatic arthritis pathogenesis. Clinical Reviews in Allergy & Immunology 55: 379–390. https://doi.org/10.1007/s12016-018-8702-3 [Google Scholar] [PubMed] [CrossRef]
Borin M, Saraceno C, Catania M, Lorenzetto E, Pontelli V et al. (2018). Rac1 activation links tau hyperphosphorylation and abeta dysmetabolism in Alzheimer’s disease. Acta Neuropathologica Communications 6: 602. https://doi.org/10.1186/s40478-018-0567-4 [Google Scholar] [PubMed] [CrossRef]
Braga TT, Agudelo JSH, Camara NOS (2015). Macrophages during the fibrotic process: M2 as friend and foe. Frontiers in Immunology 6: 953. https://doi.org/10.3389/fimmu.2015.00602 [Google Scholar] [PubMed] [CrossRef]
Carmeliet P (2005). Angiogenesis in life, disease and medicine. Nature 438: 932–936. https://doi.org/10.1038/nature04478 [Google Scholar] [PubMed] [CrossRef]
Chen S, Chen Y, Zhu Z, Tan H, Lu J, Qin P, Xu L (2020). Identification of the key genes and microRNAs in adult acute myeloid leukemia with FLT3 mutation by bioinformatics analysis. International Journal of Medical Sciences 17: 1269–1280. https://doi.org/10.7150/ijms.46441 [Google Scholar] [PubMed] [CrossRef]
Cheng F, Fransson LA, Mani K (2023). Interplay between APP and glypican-1 processing and α-synuclein aggregation in undifferentiated and differentiated human neural progenitor cells. Glycobiology 33: 325–341. https://doi.org/10.1093/glycob/cwad013 [Google Scholar] [PubMed] [CrossRef]
Costa C, Incio J, Soares R (2007). Angiogenesis and chronic inflammation: Cause or consequence? Angiogenesis 10: 149–166. https://doi.org/10.1007/s10456-007-9074-0 [Google Scholar] [PubMed] [CrossRef]
Danese S (2010). Narrow-band imaging endoscopy to assess mucosal angiogenesis in inflammatory bowel disease: A pilot study. World Journal of Gastroenterology 16: 2396. https://doi.org/10.3748/wjg.v16.i19.2396 [Google Scholar] [PubMed] [CrossRef]
Duhen R, Ballesteros-Merino C, Frye AK, Tran E, Rajamanickam V et al. (2021). Neoadjuvant anti-OX40 (MEDI6469) therapy in patients with head and neck squamous cell carcinoma activates and expands antigen-specific tumor-infiltrating T cells. Nature Communications 12: 1047. https://doi.org/10.1038/s41467-021-21383-1 [Google Scholar] [PubMed] [CrossRef]
Galimov A, Hartung A, Trepp R, Mader A, Flück M, Linke A, Blüher M, Christ E, Krützfeldt J (2015). Growth hormone replacement therapy regulates microRNA-29a and targets involved in insulin resistance. Journal of Molecular Medicine 93: 1369–1379. https://doi.org/10.1007/s00109-015-1322-y [Google Scholar] [PubMed] [CrossRef]
Garcia-Etxebarria K, Merino O, Gaite-Reguero A, Rodrigues PM, Herrarte A et al. (2022). Local genetic variation of inflammatory bowel disease in basque population and its effect in risk prediction. Scientific Reports 12: 3386. https://doi.org/10.1038/s41598-022-07401-2 [Google Scholar] [PubMed] [CrossRef]
Gijsbers K, Gouwy M, Struyf S, Wuyts A, Proost P, Opdenakker G, Penninckx F, Ectors N, Geboes K, van Damme J (2005). GCP-2/CXCL6 synergizes with other endothelial cell-derived chemokines in neutrophil mobilization and is associated with angiogenesis in gastrointestinal tumors. Experimental Cell Research 303: 331–342. https://doi.org/10.1016/j.yexcr.2004.09.027 [Google Scholar] [PubMed] [CrossRef]
Kaneko R, Matsui A, Watanabe M, Harada Y, Kanamori M et al. (2023). Increased neutrophils in inflammatory bowel disease accelerate the accumulation of amyloid plaques in the mouse model of Alzheimer’s disease. Inflammation and Regeneration 43: 20. https://doi.org/10.1186/s41232-023-00257-7 [Google Scholar] [PubMed] [CrossRef]
Kaplan GG, Windsor JW (2021). The four epidemiological stages in the global evolution of inflammatory bowel disease. Nature Reviews Gastroenterology & Hepatology 18: 56–66. https://doi.org/10.1038/s41575-020-00360-x [Google Scholar] [PubMed] [CrossRef]
Kolaczkowska E, Kubes P (2013). Neutrophil recruitment and function in health and inflammation. Nature Reviews. Immunology 13: 159–175. https://doi.org/10.1038/nri3399 [Google Scholar] [PubMed] [CrossRef]
Kudo-Saito C (2013). Fstl1 promotes bone metastasis by causing immune dysfunction. Oncoimmunology 2: e26528. https://doi.org/10.4161/onci.26528 [Google Scholar] [PubMed] [CrossRef]
Langley BO, Guedry SE, Goldenberg JZ, Hanes DA, Beardsley JA, Ryan JJ (2021). Inflammatory bowel disease and neutrophil-lymphocyte ratio: A systematic scoping review. Journal of Clinical Medicine 10: 4219. https://doi.org/10.3390/jcm10184219 [Google Scholar] [PubMed] [CrossRef]
Li L, Huang S, Yao Y, Chen J, Li J, Xiang X, Deng J, Xiong J (2020a). Follistatin-like 1 (FSTL1) is a prognostic biomarker and correlated with immune cell infiltration in gastric cancer. World Journal of Surgical Oncology 18: 324. https://doi.org/10.1186/s12957-020-02070-9 [Google Scholar] [PubMed] [CrossRef]
Li L, Milner LA, Deng Y, Iwata M, Banta A et al. (1998). The human homolog of rat jagged1 expressed by marrow stroma inhibits differentiation of 32D cells through interaction with notch1. Immunity 8: 43–55. https://doi.org/10.1016/S1074-7613(00)80457-4 [Google Scholar] [PubMed] [CrossRef]
Li T, Wang C, Liu Y, Li B, Zhang W et al. (2020b). Neutrophil extracellular traps induce intestinal damage and thrombotic tendency in inflammatory bowel disease. Journal of Crohn’s & Colitis 14: 240–253. https://doi.org/10.1093/ecco-jcc/jjz132 [Google Scholar] [PubMed] [CrossRef]
Maden SF, Acuner SE (2021). Mapping transcriptome data to protein-protein interaction networks of inflammatory bowel diseases reveals disease-specific subnetworks. Frontiers in Genetics 12: 688447. https://doi.org/10.3389/fgene.2021.688447 [Google Scholar] [PubMed] [CrossRef]
McGeachy MJ, Cua DJ, Gaffen SL (2019). The IL-17 family of cytokines in health and disease. Immunity 50: 892–906. https://doi.org/10.1016/j.immuni.2019.03.021 [Google Scholar] [PubMed] [CrossRef]
Meitei HT, Jadhav N, Lal G (2021). CCR6-CCL20 axis as a therapeutic target for autoimmune diseases. Autoimmunity Reviews 20: 102846. https://doi.org/10.1016/j.autrev.2021.102846 [Google Scholar] [PubMed] [CrossRef]
Miyabe M, Ohashi K, Shibata R, Uemura Y, Ogura Y et al. (2014). Muscle-derived follistatin-like 1 functions to reduce neointimal formation after vascular injury. Cardiovascular Research 103: 111–120. https://doi.org/10.1093/cvr/cvu105 [Google Scholar] [PubMed] [CrossRef]
Murakami K, Tanaka M, Usui T, Kawabata D, Shiomi A et al. (2012). Follistatin-related protein/follistatin-like 1 evokes an innate immune response via cd14 and toll-like receptor 4. FEBS Letters 586: 319–324. https://doi.org/10.1016/j.febslet.2012.01.010 [Google Scholar] [PubMed] [CrossRef]
Natale G, Biagioni F, Busceti CL, Gambardella S, Limanaqi F, Fornai F (2019). TREM receptors connecting bowel inflammation to neurodegenerative disorders. Cells 8: 1124. https://doi.org/10.3390/cells8101124 [Google Scholar] [PubMed] [CrossRef]
Nus M, Martínez-Poveda B, MacGrogan D, Chevre R, D’Amato G et al. (2016). Endothelial Jag1-RBPJ signalling promotes inflammatory leucocyte recruitment and atherosclerosis. Cardiovascular Research 112: 568–580. https://doi.org/10.1093/cvr/cvw193 [Google Scholar] [PubMed] [CrossRef]
Pan X, Zhu Q, Pan L, Sun J (2022). Macrophage immunometabolism in inflammatory bowel diseases: From pathogenesis to therapy. Pharmacology & Therapeutics 238: 108176. https://doi.org/10.1016/j.pharmthera.2022.108176 [Google Scholar] [PubMed] [CrossRef]
Rana N, Privitera G, Kondolf HC, Bulek K, Lechuga S et al. (2022). GSDMB is increased in IBD and regulates epithelial restitution/repair independent of pyroptosis. Cell 185: 283–298. https://doi.org/10.1016/j.cell.2021.12.024 [Google Scholar] [PubMed] [CrossRef]
Shapouri Moghaddam A, Mohammadian S, Vazini H, Taghadosi M, Esmaeili SA, Mardani F, Seifi B, Mohammadi A, Afshari JT, Sahebkar A (2018). Macrophage plasticity, polarization, and function in health and disease. Journal of Cellular Physiology 233: 6425–6440. https://doi.org/10.1002/jcp.26429 [Google Scholar] [PubMed] [CrossRef]
Shu J, Dolman GE, Duan J, Qiu G, Ilyas M (2016). Statistical colour models: An automated digital image analysis method for quantification of histological biomarkers. BioMedical Engineering Online 15: 46. https://doi.org/10.1186/s12938-016-0161-6 [Google Scholar] [PubMed] [CrossRef]
Stidham RW, Takenaka K (2022). Artificial intelligence for disease assessment in inflammatory bowel disease: How will it change our practice? Gastroenterology 162: 1493–1506. https://doi.org/10.1053/j.gastro.2021.12.238 [Google Scholar] [PubMed] [CrossRef]
Sutton SS, Magagnoli J, Cummings T, Hardin JW (2019). Association between thiopurine medication exposure and Alzheimer’s disease among a cohort of patients with inflammatory bowel disease. Alzheimer’s & Dementia 5: 809–813. https://doi.org/10.1016/j.trci.2019.10.002 [Google Scholar] [PubMed] [CrossRef]
Trivedi PJ, Adams DH (2018). Chemokines and chemokine receptors as therapeutic targets in inflammatory bowel disease; pitfalls and promise. Journal of Crohn’s & Colitis 12: S641–S652. https://doi.org/10.1093/ecco-jcc/jjx145 [Google Scholar] [PubMed] [CrossRef]
Uza N, Nakase H, Yamamoto S, Yoshino T, Takeda Y et al. (2011). SR-PSOX/CXCL16 plays a critical role in the progression of colonic inflammation. Gut 60: 1494–1505. https://doi.org/10.1136/gut.2010.221879 [Google Scholar] [PubMed] [CrossRef]
Wan X, Guan S, Hou Y, Qin Y, Zeng H et al. (2021). FOSL2 promotes VEGF-independent angiogenesis by transcriptionnally activating Wnt5a in breast cancer-associated fibroblasts. Theranostics 11: 4975–4991. https://doi.org/10.7150/thno.55074 [Google Scholar] [PubMed] [CrossRef]
Wang PL, Niidome T, Akaike A, Kihara T, Sugimoto H (2009). Rac1 inhibition negatively regulates transcriptional activity of the amyloid precursor protein gene. Journal of Neuroscience Research 87: 2105–2114. https://doi.org/10.1002/jnr.22039 [Google Scholar] [PubMed] [CrossRef]
Yan P, Hu X, Song H, Yin K, Bateman RJ et al. (2006). Matrix metalloproteinase-9 degrades amyloid-β fibrils in vitro and compact plaques in situ. The Journal of Biological Chemistry 281: 24566–24574. https://doi.org/10.1074/jbc.M602440200 [Google Scholar] [PubMed] [CrossRef]
Zhou G, Yu L, Fang L, Yang W, Yu T et al. (2018). CD177(+) neutrophils as functionally activated neutrophils negatively regulate IBD. Gut 67: 1052–1063. https://doi.org/10.1136/gutjnl-2016-313535 [Google Scholar] [PubMed] [CrossRef]
Cite This Article
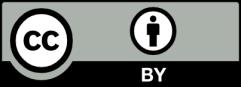