Open Access
ARTICLE
Prognostic model for prostate cancer based on glycolysis-related genes and non-negative matrix factorization analysis
1 Department of Urology, The Eighth Affiliated Hospital, Sun Yat-Sen University, Shenzhen, 518033, China
2 The First Clinical College of Guangzhou Medical University, Guangzhou, 511436, China
3 The Second Clinical College of Guangzhou Medical University, Guangzhou, 511436, China
4 Department of Social and Behavioural Sciences, City University of Hong Kong, Hong Kong, 999077, China
5 The Sixth Clinical College of Guangzhou Medical University, Guangzhou, 511436, China
6 The Third Clinical College of Guangzhou Medical University, Guangzhou, 511436, China
* Corresponding Authors: YONGCHANG LAI. Email: ; ZHAOHUI HE. Email:
(This article belongs to the Special Issue: Bioinformatics Study of Diseases)
BIOCELL 2023, 47(2), 339-350. https://doi.org/10.32604/biocell.2023.023750
Received 13 May 2022; Accepted 22 August 2022; Issue published 18 November 2022
Abstract
Background: Establishing an appropriate prognostic model for PCa is essential for its effective treatment. Glycolysis is a vital energy-harvesting mechanism for tumors. Developing a prognostic model for PCa based on glycolysis-related genes is novel and has great potential. Methods: First, gene expression and clinical data of PCa patients were downloaded from The Cancer Genome Atlas (TCGA) and Gene Expression Omnibus (GEO), and glycolysis-related genes were obtained from the Molecular Signatures Database (MSigDB). Gene enrichment analysis was performed to verify that glycolysis functions were enriched in the genes we obtained, which were used in non-negative matrix factorization (NMF) to identify clusters. The correlation between clusters and clinical features was discussed, and the differentially expressed genes (DEGs) between the two clusters were investigated. Based on the DEGs, we investigated the biological differences between clusters, including immune cell infiltration, mutation, tumor immune dysfunction and exclusion, immune function, and checkpoint genes. To establish the prognostic model, the genes were filtered based on univariable Cox regression, LASSO, and multivariable Cox regression. Kaplan–Meier analysis and receiver operating characteristic analysis validated the prognostic value of the model. A nomogram of the risk score calculated by the prognostic model and clinical characteristics was constructed to quantitatively estimate the survival probability for PCa patients in the clinical setting. Result: The genes obtained from MSigDB were enriched in glycolysis functions. Two clusters were identified by NMF analysis based on 272 glycolysis-related genes, and a prognostic model based on DEGs between the two clusters was finally established. The prognostic model consisted of LAMPS, SPRN, ATOH1, TANC1, ETV1, TDRD1, KLK14, MESP2, POSTN, CRIP2, NAT1, AKR7A3, PODXL, CARTPT, and PCDHGB2. All sample, training, and test cohorts from The Cancer Genome Atlas (TCGA) and the external validation cohort from GEO showed significant differences between the high-risk and low-risk groups. The area under the ROC curve showed great performance of this prognostic model. Conclusion: A prognostic model based on glycolysis-related genes was established, with great performance and potential significance to the clinical application.Keywords
Prostate cancer (PCa) is the second most common cancer and the main disease affecting men’s health worldwide (Nguyen-Nielsen and Borre, 2016). The treatment methods for PCa include active surveillance, radiation therapy, local ablative therapies, radical prostatectomy (RP), androgen deprivation therapy (ADT), and others (Sebesta and Anderson, 2017). For metastatic PCa, ADT has been the major treatment schedule (Ritch and Cookson, 2018). However, the majority of patients undergoing ADT develop metastatic castration-resistant PCa (mCRPC) (Karantanos et al., 2013). It is important to establish an accurate prognostic model for PCa to avoid unnecessary side effects that burden patients’ quality of life.
Non-negative matrix factorization (NMF) is an unsupervised learning technique with the advantages of reducing noise and interpretability (Devarajan, 2008; Gaujoux and Seoighe, 2010). It is currently widely used in computational biology. Unlike other clustering methods, NMF places emphasis on parts-based clustering, which is different from many unsupervised clustering methods (Devarajan, 2008). Recently, some studies have used NMF to establish risk models with good performance (Wang et al., 2020; Song et al., 2021).
The tumor microenvironment includes tumor cells and surrounding nontumor components, including cancer-associated fibroblasts, endothelial cells, immune cells, the extracellular matrix, and a hypoxic glycolysis condition (Lai et al., 2021). Glycolysis is the process in which two molecules of pyruvate are produced by the cleavage of one molecule of glucose. Tumor cells tend to obtain large quantities of energy by glycolysis instead of oxidative phosphorylation, and the energy demands of tumor cells make glycolysis more efficient (Gill et al., 2016) which is called the Warburg effect, but PCa cells do not follow the Warburg effect entirely (Eidelman et al., 2017). In general, only advanced PCa begins to exhibit high glucose uptake rather than the early PCa cells (Eidelman et al., 2017). This phenomenon may be because PCa cells require higher levels of citric acid cycling activity to consume zinc to avoid cell death (Feng et al., 2002; Eidelman et al., 2017). This metabolic feature of PCa may be a prognostic factor. A recent study showed that the abnormal overexpression of glycolytic pathway-related proteins in PCa is associated with a poor prognosis of PCa (Pertega-Gomes et al., 2015).
However, classification and construction of a high-risk and low-risk groups for the glycolysis-related genes based on the NMF method has been rarely adopted. According to the above reasons, we classified two clusters of PCa patients by NMF based on glycolysis-related genes. Through further analysis of the two clusters, we found interesting differences in survival probability and biological process between the two clusters. Then we established a prognostic model and a nomogram based on the DEGs between the clusters.
Acquisition and identification of genes associated with glycolysis
We downloaded RNA sequences (fragments per kilobase million, FPKM), clinical information, follow-up data, Gleason score, mutation data, etc., of PCa patients from the TCGA database. The samples lacking of critical clinical information were excluded. Glycolysis-related genes were obtained from MSigDB (https://www.gsea-msigdb.org/gsea/msigdb/index.jsp). To verify that the functions of the genes obtained from MSigDB were enriched in glycolysis, the Kyoto Encyclopedia of Genes and Genomes (KEGG) pathway and Gene Ontology (GO) enrichment analyses were performed by the R package “clusterProfiler”.
Acquisition of glycolysis-related gene expression profiles
The mRNA data and clinical information of the PCa patients were downloaded from TCGA (https://cancergenome.nih.gov). In addition, external validation cohorts of PCa patients were acquired from the GSE116918 series in Gene Expression Omnibus (GEO) (https://www.ncbi.nlm.nih.gov/geo/). The samples with incomplete follow-up information were discarded. The expression matrices of glycolysis-related genes were extracted from the expression profiles of TCGA and GSE116918 by the R package “limma.” Batch effects need to be considered in the use of high-throughput data for biological analysis. Different potential variables are derived from biological and abiotic factors among different batches, which may seriously affect the results (Leek et al., 2010). Therefore, the R package “sva” (Leek et al., 2012) was used to remove the batch effects. After data processing, 272 glycolysis genes and their expression profiles were finally obtained.
Identification of PCa clusters by NMF
To differentiate subclasses based on glycolysis-related genes, NMF was performed for the glycolysis-related gene expression profiles by the R package “NMF.” A suitable number of clusters was necessary for steady and available clusters (Brunet et al., 2004). Therefore, we calculated the cophenetic correlation in the different numbers of clusters and selected the value of its initial decline as the number of clusters according to Brunet’s method (Brunet et al., 2004). Finally, we selected two clusters, named cluster 1 and cluster 2. Patients with similar glycolysis states were assigned to one cluster. In other words, patients in cluster 1 had a significantly different glycolysis state from those in cluster 2.
Kaplan–Meier analysis was performed, and a curve was created; in our analysis, the event of interest was set to death. After Kaplan–Meier curves were created, log-rank tests were performed. The P-value in log-rank tests was calculated to identify any statistically significant difference in the probability of survival between the two clusters.
Analysis of the relationship between clusters and clinical features
After classification and Kaplan–Meier analysis, we focused on exploring the correlation between clusters and clinical features. The clinical features included in the analysis were T and N stages (from the TNM stage), Gleason score, race, and age. The Gleason stage is a widely used grading method for PCa (Srigley et al., 2019). It is commonly used to prognosticate patients and provide advice on appropriate treatment for PCa patients with different conditions (Srigley et al., 2019). In addition, TNM is a comprehensively accepted tumor staging method (Cserni et al., 2018). The R package “pheatmap” was used to create a heatmap that visualized the differences between the clusters and clinical features. Meanwhile, the DEGs between the two clusters were also rendered on the heatmap.
Analysis of the differences in the biological mechanism
To examine the cause of significant differences in survival probability between the two clusters, we investigated the difference in immune cell infiltration between the clusters. To quantify the infiltration of immune cells, the Microenvironment Cell Populations-counter method (Becht et al., 2016) was used based on the differential genes between the clusters. Then, the difference in immune cell infiltration scores between the two clusters was analyzed, and the results were visualized by the R package “ggpubr.” In addition, the Sankey diagram was used to visualize the congruent relationship between clusters and immune subtypes by the R package “ggalluvial.”
To analyze the mutation of the two clusters, we downloaded the mutation data of the PCa in varscan format in TCGA. The R package “maftools” is a powerful tool to analyze somatic mutations developed by Mayakonda (Mayakonda et al., 2018). To analyze the differences in immune function between clusters, we performed a single-sample gene set enrichment analysis (ssGSEA). To obtain the gene set score matrix, the R packages “GSVA” and “reshape2” were used and to label immune function information, “GSEABass” was used. Then, “limma” was used to identify differential immune function genes between the two clusters. Finally, “ggpubr” was used for visualization. Then, we assessed the difference in immune escape between the clusters. Tumor immune dysfunction and exclusion (TIDE) analysis is a scoring method for immune escape and a predictive method for immune checkpoint blockade (ICB) developed by Jiang et al. (2018). The scoring documents were downloaded from http://tide.dfci.harvard.edu/. Data processing was completed by “limma,” and for visualization, “ggpubr” was used. Finally, to analyze the difference in immune checkpoints between the clusters, “limma” and “reshape2” were used to process the data and filter DEGs. Then, the expression of the DEGs between the two clusters was compared, and the P-values of all DEGs were calculated to verify if the differences in expression were significant. “ggplot2” and “ggpubr” were used for visualization.
Establishment of the prognostic model
Differential genes between two clusters were filtered by “limma” with |log2FC| > 0.585, fold change = 1.5, and false discovery rate (FDR) < 0.05. To filter prognostic genes from differential genes, we first performed univariable Cox regression. The criterion was set to a P-value of 0.05. Then, the genes filtered by univariable Cox regression were processed by least absolute shrinkage and selection operator (LASSO) analysis to further reduce the number of genes. To avoid overfitting, the optimal penalty parameter was determined by cross-validation to filter genes further. Finally, multivariable Cox regression was performed to select the most meaningful genes from the genes filtered by univariable Cox regression and LASSO analysis to establish a prognostic model. The above analyses were based on the R packages “survival,” “caret,” “glmnet,” “survminer,” and “timeROC.” The specific formula for the risk score calculation is as follows:
The independent parameters in PCa patients were analyzed, including risk scores and clinical characteristics by univariable and multivariable Cox regression. The parameters in Cox regression with P < 0.05 were included in the construction of the nomogram. The nomogram was constructed via stepwise Cox regression to predict the probability of survival of PCa patients in TCGA for 1, 3, and 5 years. The above analyses were based on R packages “survival,” “regplot,” and “rms.”
Kaplan–Meier analysis and receiver operating characteristic curve (ROC) analysis were used to assess each cohort and verify the feasibility and prognostic value of the prognostic model. To perform the Kaplan–Meier analysis, the TCGA cohort was randomly divided into training cohorts and test cohorts. GSE116918 was the external validation cohort. To distinguish the high-risk group from the low-risk group, the criteria were set to the median risk score. Then, log-rank tests were performed to verify the significant difference between the high-risk and low-risk cohorts. After Kaplan–Meier analysis and creation of curves, ROC analysis was performed for each cohort. One of the vital indicators for ROC analysis was the area under the ROC curve (AUC). We calculated the AUC at 1, 3, and 5 years and also investigated the performance of the prognostic model in patients with pathological scores <8 and ≥8. The above analyses were based on the R packages “survival” and “time ROC.”
The continuous variables were compared by Student’s t-test in the normal distribution, while the Wilcoxon test was performed for other cases. The Kaplan-Meier curves were used, and the log-rank test was performed to analyze the survival rates. Univariable and multivariable Cox regression models were used for the independent analysis of parameters related to overall survival. All statistical tests were bilateral. P < 0.05 was considered statistically significant. The correlation between the two variables was measured by calculating the Pearson coefficient.
Identification of PCa clusters
The gene sets downloaded from MSigDB were summarized and deduplicated to filter the glycolysis-related genes. GO and KEGG enrichment analyses were performed for the genes. In GO analysis, one of the ontologies, namely, biological process (BP), showed the function of the genes enriched in glycolysis-related processes, such as pyruvate metabolism and carbohydrate catabolism. Moreover, KEGG analysis showed that the genes were enriched in glycolysis/gluconeogenesis (Fig. 1). The above results proved that the genes from MsigDB had a strong correlation with glycolysis.
Figure 1: (A) The gene ontology enrichment analysis for glycolysis-related genes. (B) The Kyoto Encyclopedia of genes and genomes pathways enrichment analysis for glycolysis-related genes. The volume of the bubbles is the number of genes. The color of the bubble represents the size of the P-value, and all the functions shown had P < 0.001. The abscissa is the gene ratio.
After the correlation test, we obtained the control samples and cancer samples in TCGA and GEO to extract the expression matrices of glycolysis-related genes. A total of 485 cancer samples were obtained from TCGA, and the GSE116918 dataset was downloaded from GEO. The expression matrices of glycolysis genes were extracted from the expression profiles from TCGA and GSE116918. We finally obtained 272 glycolysis-related genes and their expression profiles. These genes were used in the NMF analysis (Fig. 2A). The cophenetic correlation in the different numbers of clusters resulted in two suitable clusters. Based on NMF analysis, finally, two clusters, namely cluster 1 and cluster 2, were obtained.
Figure 2: (A) The consensus matrix map. The horizontal axis and the vertical axis represent the samples. Clusters that never cluster together to always cluster together are shown in blue to red. (B) Kaplan–Meier curves for the survival probability between two clusters with different states of glycolysis. The P-value was calculated to be less than 0.001 based on the log-rank test, which means the difference was considered statistically significant.
Kaplan-Meier analysis was performed and visualized (Fig. 2B). There were 207 patients classified into cluster 1 and 278 patients classified into cluster 2. The Kaplan–Meier curves indicated that the patients in cluster 2 had a greater probability of survival. To scrupulously judge the matter, log-rank tests were performed, and a P-value less than 0.001 was calculated. This means that the survival time of patients from different clusters was considered to be statistically significant, and classification was succussed and significant.
The relationship between clusters and clinical features
A heatmap was created to exhibit the correlation between clusters and clinical features, including T and N stage (from TNM stage), Gleason score, race, and age (Fig. 3). Among them, T, N, and Gleason scores reached statistical significance with a P-value of less than 0.001. The patients with higher TNM stage, namely, T3 and N1, and higher Gleason scores were mainly congregated at cluster 1, while the patients with lower TNM stage, namely, T2 and N0, and lower Gleason scores were mainly congregated at cluster 2; thus, these findings indicate a greater probability of survival for the patients in cluster 2.
Figure 3: A heatmap for the correlation between the clusters and clinical features. Each column in the heatmap represents a sample and records the information in different colors. The relationship between clinical features and clusters is shown above the row “cluster,” and gene expression levels are shown below the row “cluster.” The meaning of symbols: *** P < 0.001.
Analysis of the differences in biomechanisms between clusters
To explore the difference in immune cell infiltration between the two clusters, we compared the degrees of infiltration of different immune cells, including B lineage, CD8 T cells, cytotoxic lymphocytes, endothelial cells, fibroblasts, monocytic lineage, myeloid dendritic cells, neutrophils, natural killer (NK) cells, and T cells (Figs. 4A–4J). All the abovementioned immune cells, except for neutrophils, had significant differences between the two clusters, among which only the P-value of neutrophils was equal to 0.053, and the remaining had a P-value less than 0.001. In summary, there were interesting differences in specific immune cell infiltration between the two clusters. The relationship between the glycolysis-related NMF clusters and subtypes was also analyzed (Fig. 4K); both clusters had genes involved in four immune subtypes (namely, immune C1, C2, C3, and C4).
Figure 4: (A–J) Violin plot of differential immune cell infiltration between two clusters with different states of glycolysis. The number between the two violin plots represents the P-value (B lineage: P = 0.0000018, CD8 T cells: P = 0.0000015, cytotoxic lymphocytes: P < 0.0000001, endothelial cells: P < 0.0000001, fibroblasts: P = 0.00033, monocytic lineage: P < 0.0000001, myeloid dendritic cells: P < 0.0000001, neutrophils = 0.053, natural killer cells: P < 0.0000001, T cells: P < 0.0000001) (K) Sankey plot showing the relationship between glycolysis-related NMF clusters and immune subtypes.
In the mutation analysis, 118 of 200 samples were found to have a mutation in cluster 1 (59%), and 128 of 264 samples were found in cluster 2 (48.48%). The samples in cluster 1 had the most mutations in the TP53 gene (13%), and in cluster 2, the most mutations in the SPOP gene (13%). The main alteration was a missense mutation in both clusters (Figs. 5Aand 5B). Then, we analyzed the difference in TIDE scores between clusters (Fig. 5C). There was a significant difference in TIDE scores between the two clusters (P < 0.001). Cluster 2 had a higher TIDE score than cluster 1. In immune function analysis, the difference was significant in the scores of antigen-presenting cells (APC) co inhibition, APC co-stimulation, complete cytogenetic response, checkpoint, cytolytic activity, human leukocyte antigen, inflammation-promoting, major histocompatibility complex class 1, parainflammation, T-cell co-inhibition, T-cell co-stimulation, type I interferon (IFN) response, and type II IFN response between two clusters, with P < 0.001 (Fig. 5D). All the differences in immune functions we investigated showed amazing consistency and that the immune function scores of cluster 1 were higher than those of cluster 2. In the checkpoint differential analysis, all of the immune checkpoints we investigated showed significant differences between the high-risk group and the low-risk group, such as CD80, CD86, and CD27 (Fig. 5E).
Figure 5: (A and B) The difference in mutation between clusters with different states of glycolysis. (C) The violin figure: comparison of tumor immune dysfunction and exclusion (TIDE) scores between the two clusters. (D) Box plots: the difference in immune functions between the two clusters. (E) Box plots: the expression of checkpoint-related genes between the two clusters (*P < 0.05; **P < 0.01; ***P < 0.001).
Prognostic model establishment
Univariable Cox regression, LASSO analysis, and multivariable Cox regression were used to filter the prognostic genes (Figs. 6A and 6B). Fifteen glycolysis-related prognostic genes, namely, LAMPS, SPRN, ATOH1, TANC1, ETV1, TDRD1, KLK14, MESP2, POSTN, CRIP2, NAT1, AKR7A3, PODXL, CARTPT, and PCDHGB2, were finally selected. When the coefficient was positive, the gene was associated with high risk in PCa patients, while negative coefficients indicated low risk in PCa patients. The correlation among glycolysis-related prognostic genes was analyzed and is shown in Fig. 6C.
Figure 6: (A) Cross-validation was performed. The dotted line on the left represents the optimum penalty parameter. (B) Diagram for the locus of the coefficients of each independent variable. (C) A plot showing the correlation among 15 genes that were used to establish prognostic models. The sign “*” means the correlation is statistically significant.
Based on 15 glycolysis-related prognostic genes, we established a glycolysis-related prognostic model for PCa. The risk score of the prognostic models was calculated, and the calculation method of the risk score was as follows: risk score = (0.480*LAMPS exp.) + (1.591*SPRN exp.) + (0.505*ATOH1 exp.) + (1.197*TANC1 exp.) + (0.250*ETV1 exp.) + (-0.390*TDRD1 exp.) + (0.298*KLK14 exp.) + (0.673*MESP2 exp.) + (0.841*POSTN exp.) + (1.047*CRIP2 exp.) + (-0.419*NAT1 exp.) + (1.635*AKR7A3 exp.) + (0.953*PODXL exp.) + (0.544*CARTPT exp.) + (0.801*PCDHGB2 exp.).
Good performance of the prognostic model in a series of validation tests
The TCGA samples were categorized into training and test groups. To verify the performance of predicting survival, Kaplan–Meier analysis and ROC analysis were performed. There were four different groups, including the TCGA all group (total samples from TCGA), the TCGA training group, the TCGA test group, and an external data validation group of samples from GEO (GEO group) (Figs. 7A–7D).
Figure 7: Patients above the median risk score were assigned to the high-risk group; otherwise, patients were assigned to the low-risk group. (A–D) Kaplan–Meier curves for the survival probability between two risk groups. TCGA all group: P < 0.001. TCGA training group: P < 0.001. TCGA test: P = 0.022. GEO group: P < 0.001. (E–H) The ROC curves for different cohorts. Different colors are used to show the receiver operating characteristic (ROC) curves with different prediction times. (I–J) The Kaplan–Meier curves between high-risk and low-risk groups in histological score of < 8 (P < 0.001) or ≥ 8 (P < 0.001).
The result was that the P-values were less than 0.001 in the TCGA all group, the TCGA training group, and the GEO group, and the P-value was 0.022 in the TCGA test group. In the TCGA all-group, the AUCs at 1, 3, and 5 years were 0.892, 0.875, and 0.816, respectively. In the ROC analysis of the TCGA training group, the AUCs at 1, 3, and 5 years were 0.940, 0.935, and 0.929, respectively. In the ROC analysis of the TCGA test group, the AUCs at 1, 3, and 5 years were 0.724, 0.671, and 0.669, respectively. In the ROC analysis of the GEO group, the AUCs at 1, 3, and 5 years were 0.927, 0.656, and 0.605, respectively (Figs. 7E–7H). These findings suggest that our model had great performance for prognosis. We also performed a survival probability analysis for the patients with histological scores < 8 and ≥ 8 (Figs. 7I–7J). The results showed a significant difference between high-risk and low-risk patients with histological scores < 8 (P < 0.001) and ≥ 8 (P = 0.003). Thus, the prognostic model we established could precisely divide patients into high-risk and low-risk groups regardless of whether the patients had a histological score <8 or ≥8. Therefore, the prognostic model could effectively distinguish the risk of PCa in patients.
We constructed a nomogram in the TCGA training group to quantitatively estimate the survival probability for PCa patients in the clinical setting. Univariable and multivariable Cox regression for risk scores and clinical characteristics were performed to screen independent prognostic factors. In the univariable Cox regression, Gleason score (P < 0.001, Hazard ratio = 2.039 and 95% CI: 1.435–2.896), T stage in TNM (P < 0.001, Hazard ratio = 3.064 and 95% CI: 1.595–5.882), N stage in TNM (P = 0.049, Hazard ratio = 2.101 and 95% CI: 1.003–4.400), and risk score (P < 0.001, Hazard ratio = 1.004 and 95% CI: 1.003–1.005) were considered to be correlated with the prognosis of PCa patients (Fig. 8A). In multivariable Cox regression, Gleason score (P = 0.025, Hazard ratio = 1.612 and 95% CI: 1.063–2.445), T stage in TNM (P = 0.032, Hazard ratio = 2.350 and 95% CI: 1.076–5.135), and risk score (P < 0.001, Hazard ratio = 1.005 and 95% CI: 1.003–1.007) were considered the parameters for the construction of nomogram (Fig. 8B). Age, race, and N stage were excluded because they were not considered to be significantly related to prognosis in the Cox regression (P > 0.05 and/or confidence interval (CI) spanning 1). Then, the nomogram was constructed consisting of the above significant parameters to predict the probability of 1, 3, and 5 survivals of the PCa patients by stepwise Cox regression, which consisted of Gleason score, T stage, and risk scores (Fig. 8C).
Figure 8: Construction of nomogram. (A) Forest plot for univariable Cox regression (B) Forest plot for multivariable Cox regression (C) A prognostic nomogram consisting of Gleason scores, T stage, and risk scores for predicting the 1-, 3-, and 5-year overall survival of PCa patients.
Despite the low mortality of patients with PCa, one-third of men, after treatment, experience relapse, and advanced PCa may finally progress to castration-resistant disease (Bansal et al., 2021). Despite treatment, many patients with moderate and above-risk localized or castration-resistant PCa die of the disease (Teo et al., 2019). It is difficult to manage PCa because it is heterogeneous and complex (Sebesta and Anderson, 2017). Positive treatment for PCa often brings side effects, such as erectile dysfunction and urinary toxicity (Sebesta and Anderson, 2017). ADT has been the major treatment schedule for metastatic PCa (Ritch and Cookson, 2018); however, the side effects of ADT have been debated. ADT may lead to cognitive decline and reduced quality of life (Nelson et al., 2008; Reiss et al., 2022). Therefore, an accurate and reliable prognostic model is urgently needed to predict subsequent survival for PCa patients and avoid the risk of side effects after treatment.
There has been some literature supporting the influence of glycolysis-related genes on the prognosis of patients with PCa (Pertega-Gomes et al., 2015; Shangguan et al., 2021). A study showed that the expression of glycolysis-related proteins, including glucose transporter 1 (GLUT1), lactate dehydrogenase 5 (LDH5), and some other classic glycolysis-associated proteins, were associated with the progression of PCa (Pertega-Gomes et al., 2015). The abnormal expression of a molecule regulated by glycolysis-related gene hexokinase 2 (HK2, a gene that encodes one of the known hexokinase isoforms) has also been shown to be associated with increased glycolysis in PCa cells and lead to poor outcomes and bad chemotherapy response in PCa patients (Shangguan et al., 2021). With the purpose of developing prognostic models, we realized that glycolysis is a vital energy-harvesting mechanism for tumors. We obtained 272 proven glycolysis-related genes for NMF analysis, and two clusters with different glycolysis states were divided with clear and sharp boundaries in the NMF analysis. The DEGs between the two clusters were subjected to univariable Cox regression, lasso analysis, and multivariable Cox regression. We finally obtained 15 genes, including LAMPS, SPRN, ATOH1, TANC1, ETV1, TDRD1, KLK14, MESP2, POSTN, CRIP2, NAT1, AKR7A3, PODXL, CARTPT, and PCDHGB2 to establish the risk model.
In our model, LAMPS, SPRN, ATOH1, TANC1, ETV1, KLK14, MESP2, POSTN, CRIP2, AKR7A3, PODXL, CARTPT, and PCDHGB2 are considered to promote PCa, while TDRD1 and NAT1 are considered to inhibit tumor progression. The ETS factors and PCa have been extensively studied. Some ETS factors, including the transcription factor ETS-related gene and ETS variant 1, are abnormally expressed in PCa (Qian et al., 2022). The overexpression of ETV1 increases the ability of PCa cells to migrate, invade, and increase androgen metabolism (Oh et al., 2019). This is consistent with our findings that ETV1 is a risk factor in the prognostic model. In published studies, Kallikrein-related peptidase 14 (KLK14) was found to be associated with PCa aggressiveness (Kryza et al., 2020), and podocalyxin (PODXL) was also found to be associated with PCa aggressiveness (Casey et al., 2006). Our study also confirmed that KLK14 and PODXL promote cancer. Cysteine-rich intestinal protein 2 (CRIP2) is a protein with rich cysteine and has been reported to act as a suppressor in other cancers (Cheung et al., 2011; Lo et al., 2012). However, high expression of CRIP2 was strongly associated with a high-risk factor in our study, with a coefficient of even more than 1. There are few reports about the relationship between CRIP2 and PCa; the identification of high expression of CRIP2 as a risk factor remains to be determined.
During the process of establishing the prognostic model, we found a significant difference in the probability between the clusters. Therefore, we investigated biological differences. The TP53 protein is commonly regarded as a tumor suppressor protein (Aubrey et al., 2016), but TP53 mutation is considered a complex biomarker (Olivier et al., 2010). In our study, cluster 1 had 19% TP53 alterations, while cluster 2 had only 2% alterations. In the survival analysis for the two clusters, the mortality of cluster 1 was higher than that of cluster 2. This might be explained by the high mutations in TP53 in cluster 1.
Immune cell infiltration plays a complex biological role in the development and progression of PCa (Andersen et al., 2021), and more studies have shown that immune cell infiltration is associated with PCa prognosis (Kiniwa et al., 2007). Some of the studies have shown that some kinds of T cells might promote PCa (Ellem et al., 2009; Valdman et al., 2010; Flammiger et al., 2012; Yuan et al., 2013; Strasner and Karin, 2015). PCa cells benefit from regulatory T (Treg) cells because both CD4 + CD25 + FoxP3+ and CD8+ FoxP3+ T reg cells can be found in PCa and play a powerful immunosuppressive role through contact-dependent and cytokine-dependent suppression (Kiniwa et al., 2007; Stultz and Fong, 2021) which affect the prognosis (Davidsson et al., 2012; Andersen et al., 2021). Some of the studies have also shown that B cells promote PCa cancer (Ammirante et al., 2010; Shalapour et al., 2015; Strasner and Karin, 2015), which may be associated with IkappaB kinase α, interleukin10, and other cytokines (Ammirante et al., 2013; Shalapour et al., 2015). The presence of B cells is associated with a high-grade and high risk of recurrence of PCa (Woo et al., 2014), which may have prognostic significance. Neutrophils, a type of innate immune cell, are associated with the outcome of the patients (Shiao et al., 2016), and neutrophils are also reported to promote PCa (Nuhn et al., 2014; Sonpavde et al., 2014; Templeton et al., 2014; Strasner and Karin, 2015). In the immune cell infiltration analysis, we found that the infiltration of immune cells, such as B lineage, and T cells, was higher in cluster 1 than that in cluster 2. In addition, the immune function analysis revealed a higher score for cluster 1 than that for cluster 2. Through Kaplan–Meier curves, we found that cluster 2 had a higher survival probability. The result was more inclined to suggest that high immune infiltration is a risk factor for PCa patients. Meanwhile, the infiltration levels of T cells and B cells were higher in cluster 1, confirming the conclusion in correlational studies that these cells promote PCa.
Our study was the first research for classifying PCa by NMF based on glycolysis-related genes and then established a prognostic model with good performance. The results of a series of validations, including internal TCGA and external GEO validation, confirmed that the 15 genes based on glycolysis and NMF analysis have a good prognosis potential (the 1-year AUC of the TCGA all group was 0.892). It can provide a solution to the prognosis for PCa patients and help patients choose appropriate treatment options. Besides, the glycolysis-related genes were proved to be associated with the prognosis of the PCa patients in this study and also provide new understandings of the pathogenesis of PCa. However, our study had a few shortcomings and limitations. All of the datasets used for training and testing were from TCGA and GEO; the prognostic performance of the model should be further verified.
Availability of Data and Materials: The mRNA data and clinical data associated with the PCa patient samples were downloaded from the TCGA database (https://cancergenome.nih.gov/) and GEO database (https://www.ncbi.nlm.nih.gov/geo/). Information of glycolysis-related genes were downloaded from MSigDB (https://www.gsea-msigdb.org/gsea/msigdb/index.jsp).
Author Contribution: The authors confirm contribution to the paper as follows: study conception and design: Zechao Lu, Fucai Tang, Haobin Zhou, Zeguang Lu; data collection: Zechao Lu, Fucai Tang, Haobin Zhou, Zeguang Lu; analysis and interpretation of results: Zechao Lu, Fucai Tang, Haobin Zhou, Zeguang Lu; draft manuscript preparation: Zechao Lu, Fucai Tang, Haobin Zhou, Zeguang Lu, Wanyan Cai, Jiahao Zhang, Zhicheng Tang, Yongchang Lai, Zhaohui He; investigation: Zechao Lu, Fucai Tang, Haobin Zhou, Zeguang Lu, Wanyan Cai, Jiahao Zhang; project administration: Zechao Lu, Fucai Tang, Haobin Zhou, Zeguang Lu, Yongchang Lai, Zhaohui He. Zechao Lu, Fucai Tang, Haobin Zhou, Zeguang Lu make an equally important and indispensable contribution to research. All authors reviewed the results and approved the final version of the manuscript.
Ethics Approval: Not applicable.
Funding Statement: The present study was supported by the Public Health Research Project in Futian District, Shenzhen (Grant Nos. FTWS2020026, FTWS2021073).
Conflicts of Interest: The authors declare that they have no conflicts of interest to report regarding the present study.
References
- Ammirante, M., Kuraishy, AI., Shalapour, S., Strasner, A., Ramirez-Sanchez, C., Zhang, WZ., Shabaik, A., & Karin, M. (2013). An IKK alpha-E2F1-BMI1 cascade activated by infiltrating B cells controls prostate regeneration and tumor recurrence. Genes & Development, 27, 1435-1440. [Google Scholar] [CrossRef]
- Ammirante, M., Luo, JL., Grivennikov, S., Nedospasov, S., & Karin, M. (2010). B-cell-derived lymphotoxin promotes castration-resistant prostate cancer. Nature, 464, 302-305. [Google Scholar] [CrossRef]
- Andersen, LB., Nørgaard, M., Rasmussen, M., Fredsøe, J., Borre, M., Ulhøi, BP., & Sørensen, KD. (2021). Immune cell analyses of the tumor microenvironment in prostate cancer highlight infiltrating regulatory T cells and macrophages as adverse prognostic factors. Journal of Pathology, 255, 155-165. [Google Scholar] [CrossRef]
- Aubrey, BJ., Strasser, A., & Kelly, GL. (2016). Tumor-suppressor functions of the TP53 pathway. Cold Spring Harbor Perspectives in Medicine, 6, a026062. [Google Scholar] [CrossRef]
- Bansal, D., Reimers, MA., Knoche, EM., & Pachynski, RK. (2021). Immunotherapy and immunotherapy combinations in metastatic castration-resistant prostate cancer. Cancers, 13, 334. [Google Scholar] [CrossRef]
- Becht, E., Giraldo, NA., Lacroix, L., Buttard, B., & Elarouci, N. (2016). Estimating the population abundance of tissue-infiltrating immune and stromal cell populations using gene expression. Genome Biology, 17, 218. [Google Scholar] [CrossRef]
- Brunet, JP., Tamayo, P., Golub, TR., & Mesirov, JP. (2004). Metagenes and molecular pattern discovery using matrix factorization. PNAS, 101, 4164-4169. [Google Scholar] [CrossRef]
- Casey, G., Neville, PJ., Liu, X., Plummer, SJ., & Cicek, MS. (2006). Podocalyxin variants and risk of prostate cancer and tumor aggressiveness. Human Molecular Genetics, 15, 735-741. [Google Scholar] [CrossRef]
- Cheung, AKL., Ko, JMY., Lung, HL., Chan, KW., & Stanbridge, EJ. (2011). Cysteine-rich intestinal protein 2 (CRIP2) acts as a repressor of NF-kappa B-mediated proangiogenic cytokine transcription to suppress tumorigenesis and angiogenesis. PNAS, 108, 8390-8395. [Google Scholar] [CrossRef]
- Cserni, G., Chmielik, E., Cserni, B., & Tot, T. (2018). The new TNM-based staging of breast cancer. Virchows Archiv, 472, 697-703. [Google Scholar] [CrossRef]
- Davidsson, S., Ohlson, AL., Andersson, SO., Fall, K., Meisner, A., Fiorentino, M., Andrén, O., & Rider, JR. (2012). CD4 helper T cells, CD8 cytotoxic T cells, and FOXP3+ regulatory T cells with respect to lethal prostate cancer. Modern Pathology, 26, 448-455. [Google Scholar] [CrossRef]
- Devarajan, K. (2008). Nonnegative matrix factorization: An analytical and interpretive tool in computational biology. PLoS Computational Biology, 4, e1000029. [Google Scholar] [CrossRef]
- Eidelman, E., Twum-Ampofo, J., Ansari, J., & Siddiqui, MM. (2017). The metabolic phenotype of prostate cancer. Frontiers in Oncology, 7, 131. [Google Scholar] [CrossRef]
- Ellem, SJ., Wang, H., Poutanen, M., & Risbridger, GP. (2009). Increased endogenous estrogen synthesis leads to the sequential induction of prostatic inflammation (Prostatitis) and prostatic pre-malignancy. American Journal of Pathology, 175, 1187-1199. [Google Scholar] [CrossRef]
- Feng, P., Li, TL., Guan, ZX., Franklin, RB., & Costello, LC. (2002). Direct effect of zinc on mitochondrial apoptogenesis in prostate cells. The Prostate, 52, 311-318. [Google Scholar] [CrossRef]
- Flammiger, A., Bayer, F., Cirugeda-Kuehnert, A., Huland, H., & Tennstedt, P. (2012). Intratumoral T but not B lymphocytes are related to clinical outcome in prostate cancer. APMIS, 120, 901-908. [Google Scholar] [CrossRef]
- Gaujoux, R., & Seoighe, C. (2010). A flexible R package for nonnegative matrix factorization. BMC Bioinformatics, 11, 367. [Google Scholar] [CrossRef]
- Gill, KS., Fernandes, P., O’Donovan, TR., McKenna, SL., Doddakula, KK., Power, DG., Soden, DM., & Forde, PF. (2016). Glycolysis inhibition as a cancer treatment and its role in an anti-tumour immune response. Biochimica et Biophysica Acta-Reviews on Cancer, 1866, 87-105. [Google Scholar] [CrossRef]
- Jiang, P., Gu, S., Pan, D., Fu, J., & Sahu, A. (2018). Signatures of T cell dysfunction and exclusion predict cancer immunotherapy response. Nature Medicine, 24, 1550-1558. [Google Scholar] [CrossRef]
- Karantanos, T., Corn, PG., & Thompson, TC. (2013). Prostate cancer progression after androgen deprivation therapy: Mechanisms of castrate resistance and novel therapeutic approaches. Oncogene, 32, 5501-5511. [Google Scholar] [CrossRef]
- Kiniwa, Y., Miyahara, Y., Wang, HY., Peng, W., Peng, G., Wheeler, TM., Thompson, TC., Old, LJ., & Wang, RF. (2007). CD Foxp regulatory T cells mediate immunosuppression in prostate cancer. Clinical Cancer Research, 13, 6947-6958. [Google Scholar] [CrossRef]
- Kryza, T., Bock, N., Lovell, S., Rockstroh, A., & Lehman, ML. (2020). The molecular function of kallikrein-related peptidase 14 demonstrates a key modulatory role in advanced prostate cancer. Molecular Oncology, 14, 105-128. [Google Scholar] [CrossRef]
- Lai, YC., Tang, FC., Huang, YP., He, CW., Chen, CH., Zhao, JQ., Wu, WQ., & He, ZH. (2021). The tumour microenvironment and metabolism in renal cell carcinoma targeted or immune therapy. Journal of Cellular Physiology, 236, 1616-1627. [Google Scholar] [CrossRef]
- Leek, JT., Johnson, WE., Parker, HS., Jaffe, AE., & Storey, JD. (2012). The SVA package for removing batch effects and other unwanted variation in high-throughput experiments. Bioinformatics, 28, 882-883. [Google Scholar] [CrossRef]
- Leek, JT., Scharpf, RB., Bravo, HC., Simcha, D., Langmead, B., Johnson, WE., Geman, D., Baggerly, K., & Irizarry, RA. (2010). Tackling the widespread and critical impact of batch effects in high-throughput data. Nature Reviews Genetics, 11, 733-739. [Google Scholar] [CrossRef]
- Lo, PHY., Ko, JMY., Yu, ZY., Law, S., Wang, LD., Li, JL., Srivastava, G., Tsao, SW., Stanbridge, EJ., & Lung, ML. (2012). The LIM domain protein, CRIP2, promotes apoptosis in esophageal squamous cell carcinoma. Cancer Letters, 316, 39-45. [Google Scholar] [CrossRef]
- Mayakonda, A., Lin, D-C., Assenov, Y., Plass, C., & Koeffler, HP. (2018). Maftools: Efficient and comprehensive analysis of somatic variants in cancer. Genome Research, 28, 1747-1756. [Google Scholar] [CrossRef]
- Nelson, CJ., Lee, JS., Garnboa, MC., & Roth, AJ. (2008). Cognitive effects of hormone therapy in men with prostate cancer. Cancer, 113, 1097-1106. [Google Scholar] [CrossRef]
- Nguyen-Nielsen, M., & Borre, M. (2016). Diagnostic and therapeutic strategies for prostate cancer. Seminars in Nuclear Medicine, 46, 484-490. [Google Scholar] [CrossRef]
- Nuhn, P., Vaghasia, AM., Goyal, J., Zhou, XC., Carducci, MA., Eisenberger, MA., & Antonarakis, ES. (2014). Association of pretreatment neutrophil-to-lymphocyte ratio (NLR) and overall survival (OS) in patients with metastatic castration-resistant prostate cancer (mCRPC) treated with first-line docetaxel. BJU International, 114, E11-E17. [Google Scholar] [CrossRef]
- Oh, S., Shin, S., Song, H., Grande, JP., & Janknecht, R. (2019). Relationship between ETS transcription factor ETV1 and TGF-beta-regulated SMAD proteins in prostate cancer. Scientific Reports, 9, 8186. [Google Scholar] [CrossRef]
- Olivier, M., Hollstein, M., & Hainaut, P. (2010). TP53 mutations in human cancers: Origins, consequences, and clinical use. Cold Spring Harbor Perspectives in Biology, 2, 17. [Google Scholar] [CrossRef]
- Pertega-Gomes, N., Felisbino, S., Massie, CE., Vizcaino, JR., & Coelho, R. (2015). A glycolytic phenotype is associated with prostate cancer progression and aggressiveness: A role for monocarboxylate transporters as metabolic targets for therapy. Journal of Pathology, 236, 517-530. [Google Scholar] [CrossRef]
- Qian, C., Li, D., & Chen, Y. (2022). ETS factors in prostate cancer. Cancer Letters, 530, 181-189. [Google Scholar] [CrossRef]
- Reiss, AB., Saeedullah, U., Grossfeld, DJ., Glass, AD., Pinkhasov, A., & Katz, AE. (2022). Prostate cancer treatment and the relationship of androgen deprivation therapy to cognitive function. Clinical & Translational Oncology, 24, 733-741. [Google Scholar] [CrossRef]
- Ritch, C., & Cookson, M. (2018). Recent trends in the management of advanced prostate cancer. F1000Research, 7, 1513. [Google Scholar] [CrossRef]
- Sebesta, EM., & Anderson, CB. (2017). The surgical management of prostate cancer. Seminars in Oncology, 44, 347-357. [Google Scholar] [CrossRef]
- Shalapour, S., Font-Burgada, J., di Caro, G., Zhong, Z., & Sanchez-Lopez, E. (2015). Immunosuppressive plasma cells impede T-cell-dependent immunogenic chemotherapy. Nature, 521, 94-98. [Google Scholar] [CrossRef]
- Shangguan, X., He, J., Ma, Z., zhang, W., & Ji, Y. (2021). SUMOylation controls the binding of hexokinase 2 to mitochondria and protects against prostate cancer tumorigenesis. Nature Communications, 12, 1812. [Google Scholar] [CrossRef]
- Shiao, SL., Chu, GCY., & Chung, LWK. (2016). Regulation of prostate cancer progression by the tumor microenvironment. Cancer Letters, 380, 340-348. [Google Scholar] [CrossRef]
- Song, W., He, X., Gong, P., Yang, Y., Huang, S., Zeng, Y., Wei, L., & Zhang, J. (2021). Glycolysis-related gene expression profiling screen for prognostic risk signature of pancreatic ductal adenocarcinoma. Frontiers in Genetics, 12, 639246. [Google Scholar] [CrossRef]
- Sonpavde, G., Pond, GR., Armstrong, AJ., Clarke, SJ., & Vardy, JL. (2014). Prognostic impact of the neutrophil-to-lymphocyte ratio in men with metastatic castration-resistant prostate cancer. Clinical Genitourinary Cancer, 12, 317-324. [Google Scholar] [CrossRef]
- Srigley, JR., Delahunt, B., Samaratunga, H., Billis, A., & Cheng, L. (2019). Controversial issues in gleason and international society of urological pathology (ISUP) prostate cancer grading: Proposed recommendations for international implementation. Pathology, 51, 463-473. [Google Scholar] [CrossRef]
- Strasner, A., & Karin, M. (2015). Immune infiltration and prostate cancer. Frontiers in Oncology, 5, 128. [Google Scholar] [CrossRef]
- Stultz, J., & Fong, L. (2021). How to turn up the heat on the cold immune microenvironment of metastatic prostate cancer. Prostate Cancer and Prostatic Diseases, 24, 697-717. [Google Scholar] [CrossRef]
- Templeton, AJ., Pezaro, C., Omlin, A., McNamara, MG., Leibowitz-Amit, R., Vera-Badillo, FE., Attard, G., de Bono, JS., Tannock, IF., & Amir, E. (2014). Simple prognostic score for metastatic castration-resistant prostate cancer with incorporation of neutrophil-to-lymphocyte ratio. Cancer, 120, 3346-3352. [Google Scholar] [CrossRef]
- Teo MY, Rathkopf DE, Kantoff P (2019). Treatment of advanced prostate cancer. In: Klotman ME (ed.), Annual Review of Medicine, vol. 70, pp. 479–499. DOI 10.1146/annurev-med-051517-011947. [CrossRef]
- Valdman, A., Jaraj, SJ., Comperat, E., Charlotte, F., Roupret, M., Pisa, P., & Egevad, L. (2010). Distribution of Foxp3-, CD4- and CD8-positive lymphocytic cells in benign and malignant prostate tissue. APMIS, 118, 360-365. [Google Scholar] [CrossRef]
- Wang, Q., Li, M., Yang, M., Yang, Y., Song, F., Zhang, W., Li, X., & Chen, K. (2020). Analysis of immune-related signatures of lung adenocarcinoma identified two distinct subtypes: Implications for immune checkpoint blockade therapy. Aging, 12, 3312-3339. [Google Scholar] [CrossRef]
- Woo, JR., Liss, MA., Muldong, MT., Palazzi, K., & Strasner, A. (2014). Tumor infiltrating B-cells are increased in prostate cancer tissue. Journal of Translational Medicine, 12, 30. [Google Scholar] [CrossRef]
- Yuan, H., Hsiao, YH., Zhang, Y., Wang, J., Yin, C., Shen, R., & Su, Y. (2013). Destructive impact of t-lymphocytes, NK and mast cells on basal cell layers: Implications for tumor invasion. BMC Cancer, 13, 258. [Google Scholar] [CrossRef]
Cite This Article
LU, Z., TANG, F., ZHOU, H., LU, Z., CAI, W. et al. (2023). Prognostic model for prostate cancer based on glycolysis-related genes and non-negative matrix factorization analysis. BIOCELL, 47(2), 339–350. https://doi.org/10.32604/biocell.2023.023750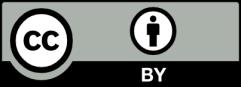