Open Access
ABSTRACT
Mechanics Based Tomography Using Camera Images
1 Texas A&M University, College Station, TX 77843, USA.
2 State Key Laboratory of Structural Analysis for Industrial Equipment, Department of Engineering Mechanics, Dalian University of Technology, Dalian 116023, China.
3 International Research Center for Computational Mechanics, Dalian University of Technology, Dalian 116023, China.
* Corresponding Author: Sevan Goenezen. Email: sgoenezen@tamu.edu.
Molecular & Cellular Biomechanics 2019, 16(Suppl.2), 46-48. https://doi.org/10.32604/mcb.2019.07348
Abstract
It is well known that the mechanical properties of tissues may vary spatially due to changing tissue types or due to inherent tissue disease. For example, the biomechanical properties are known to vary throughout blood vessels [1]. Diseases such as cancers may also lead to locally altered mechanical properties, thus allow a preliminary diagnosis via finger palpation. Quantifying the mechanical property distribution of tissues for a given constitutive equation will allow to characterize the biomechanical response of tissues. This may help to 1) predict disease progression, 2) diagnose diseases that alter the biomechanics of the tissue, e.g., skin cancers, breast cancers, prostate cancers, etc., and 3) engineer tissues with representative biomechanical behavior of the actual tissues.Strain imaging was the first attempt to infer the mechanical property composition of tissues [2] based on the assumption that the stress distribution is uniform in the problem domain. This concept evolved to solving actual mathematical problems for the mechanical property distribution using measured displacement fields and is referred to as inverse problems in solid mechanics. Displacements are often measured using ultrasound based techniques that utilize sequentially recorded images during tissue deformation. This technique relies on random speckles in the tissue’s domain of interest to track displacements on grid points via “window” similarities. The discrete displacement points are then interpolated throughout the entire problem domain to continuously determine the displacement field.
The solution of the mechanical property distribution discussed above utilizes full field displacement fields, i.e., displacement fields measured in the entire domain. An alternative approach we propose here is to utilize displacements measured solely on the domain’s boundary. This idea has been successfully developed in other disciplines, namely in computed tomography, ultrasound tomography [3], and geological sciences. Consequently, we term this methodology as “MECHANICS BASED TOMOGRAPHY” [4, 5]. We have tested this approach with synthetic data, created by solving boundary value problems for the problem domain defined in Fig. 1, where the left image defines the problem domain with the shear modulus distribution µ. The right image reveals the stiff cylindrical inclusions and defines boundary conditions, with the bottom fixed and pairwise forces applied on the sides that are depicted by arrows. The pairwise forces are applied sequentially and the area they act upon is depicted with orange circular regions. The displacement response is computed using finite element methods and recorded on the 3 green shaded faces and utilized to recover the target distribution. We also add random Gaussian noise to the displacements to mimic noisy displacement measurements. In this talk, we will elaborate more on the mathematical problem formulation and present reconstructions using actual displacement measurements on a similar problem domain made of a composite silicone material. The displacement fields will be determined using a digital image correlation system. The associate reconstructed shear modulus distributions in the presence of different noise levels are shown in Fig. 2.
We note that past works by other research groups aiming to recover the mechanical property distribution from measured surface displacements made major simplifications. In those works, it was assumed that the shear modulus values in the problem domain (e.g., background value and inclusion value) were known, but they were unknown within each finite element. Other works assumed the locally homogenous regions (e.g., location and geometry of inclusions) to be known. In our studies, we do not make such assumptions and assume that the shear moduli are unknowns on the finite element mesh nodes. In the problem presented in this talk, we will solve for more than 50,000 unknowns in the problem domain. With that, the proposed method is not confined to simple inclusion geometries, but allows distributions of any type.
Keywords
Cite This Article
Citations
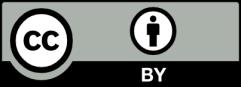