Open Access
ARTICLE
Artificial Intelligence Enabled Apple Leaf Disease Classification for Precision Agriculture
1 Department of Computer Science, King Khalid University, Muhayel Aseer, Saudi Arabia
2 Faculty of Computer and IT, Sana'a University, Yemen
3 Department of Information Systems, College of Computer and Information Sciences, Princess Nourah Bint Abdulrahman University, Saudi Arabia
4 Department of Computer and Self Development, Preparatory Year Deanship, Prince Sattam bin Abdulaziz University, Alkharj, Saudi Arabia
* Corresponding Author: Fahd N. Al-Wesabi. Email:
Computers, Materials & Continua 2022, 70(3), 6223-6238. https://doi.org/10.32604/cmc.2022.021299
Received 28 June 2021; Accepted 29 July 2021; Issue published 11 October 2021
Abstract
Precision agriculture enables the recent technological advancements in farming sector to observe, measure, and analyze the requirements of individual fields and crops. The recent developments of computer vision and artificial intelligence (AI) techniques find a way for effective detection of plants, diseases, weeds, pests, etc. On the other hand, the detection of plant diseases, particularly apple leaf diseases using AI techniques can improve productivity and reduce crop loss. Besides, earlier and precise apple leaf disease detection can minimize the spread of the disease. Earlier works make use of traditional image processing techniques which cannot assure high detection rate on apple leaf diseases. With this motivation, this paper introduces a novel AI enabled apple leaf disease classification (AIE-ALDC) technique for precision agriculture. The proposed AIE-ALDC technique involves orientation based data augmentation and Gaussian filtering based noise removal processes. In addition, the AIE-ALDC technique includes a Capsule Network (CapsNet) based feature extractor to generate a helpful set of feature vectors. Moreover, water wave optimization (WWO) technique is employed as a hyperparameter optimizer of the CapsNet model. Finally, bidirectional long short term memory (BiLSTM) model is used as a classifier to determine the appropriate class labels of the apple leaf images. The design of AIE-ALDC technique incorporating the WWO based CapsNet model with BiLSTM classifier shows the novelty of the work. A wide range of experiments was performed to showcase the supremacy of the AIE-ALDC technique. The experimental results demonstrate the promising performance of the AIE-ALDC technique over the recent state of art methods.Keywords
Cite This Article
F. N. Al-Wesabi, A. Abdulrahman Albraikan, A. Mustafa Hilal, M. M. Eltahir, M. Ahmed Hamza et al., "Artificial intelligence enabled apple leaf disease classification for precision agriculture," Computers, Materials & Continua, vol. 70, no.3, pp. 6223–6238, 2022. https://doi.org/10.32604/cmc.2022.021299Citations
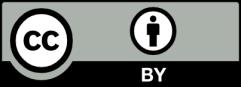