Open Access
ARTICLE
DNNBoT: Deep Neural Network-Based Botnet Detection and Classification
Department of Computer Science, College of Computer and Information Sciences, Majmaah University, Al-Majmaah 11952, Saudi Arabia
* Corresponding Author: Mohd Abdul Rahim Khan. Email:
(This article belongs to the Special Issue: Advanced IoT Industrial Solutions and Cyber Security Threats in Communication Networks)
Computers, Materials & Continua 2022, 71(1), 1729-1750. https://doi.org/10.32604/cmc.2022.020938
Received 15 June 2021; Accepted 30 August 2021; Issue published 03 November 2021
Abstract
The evolution and expansion of IoT devices reduced human efforts, increased resource utilization, and saved time; however, IoT devices create significant challenges such as lack of security and privacy, making them more vulnerable to IoT-based botnet attacks. There is a need to develop efficient and faster models which can work in real-time with efficiency and stability. The present investigation developed two novels, Deep Neural Network (DNN) models, DNNBoT1 and DNNBoT2, to detect and classify well-known IoT botnet attacks such as Mirai and BASHLITE from nine compromised industrial-grade IoT devices. The utilization of PCA was made to feature extraction and improve effectual and accurate Botnet classification in IoT environments. The models were designed based on rigorous hyperparameters tuning with GridsearchCV. Early stopping was utilized to avoid the effects of overfitting and underfitting for both DNN models. The in-depth assessment and evaluation of the developed models demonstrated that accuracy and efficiency are some of the best-performed models. The novelty of the present investigation, with developed models, bridge the gaps by using a real dataset with high accuracy and a significantly lower false alarm rate. The results were evaluated based on earlier studies and deemed efficient at detecting botnet attacks using the real dataset.Keywords
Cite This Article
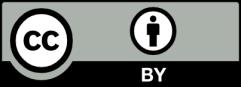