Open Access
ARTICLE
Forecasting Mental Stress Using Machine Learning Algorithms
1 Department of Software Engineering, Daffodil University, Dhaka, Bangladesh
2 Collage of Computer Science and Information System, Najran University, Najran, Saudi Arabia
3 Department of Computer Science and Engineering, Khwaja Yunus Ali University, Sirajganj 6751, Bangladesh
* Corresponding Author: Abdulwahab Alazeb. Email:
Computers, Materials & Continua 2022, 72(3), 4945-4966. https://doi.org/10.32604/cmc.2022.027058
Received 10 January 2022; Accepted 08 March 2022; Issue published 21 April 2022
Abstract
Depression is a crippling affliction and affects millions of individuals around the world. In general, the physicians screen patients for mental health disorders on a regular basis and treat patients in collaboration with psychologists and other mental health experts, which results in lower costs and improved patient outcomes. However, this strategy can necessitate a lot of buy-in from a large number of people, as well as additional training and logistical considerations. Thus, utilizing the machine learning algorithms, patients with depression based on information generally present in a medical file were analyzed and predicted. The methodology of this proposed study is divided into six parts: Proposed Research Architecture (PRA), Data Pre-processing Approach (DPA), Research Hypothesis Testing (RHT), Concentrated Algorithm Pipeline (CAP), Loss Optimization Stratagem (LOS), and Model Deployment Architecture (MDA). The Null Hypothesis and Alternative Hypothesis are applied to test the RHT. In addition, Ensemble Learning Approach (ELA) and Frequent Model Retraining (FMR) have been utilized for optimizing the loss function. Besides, the Features Importance Interpretation is also delineated in this research. These forecasts could help individuals connect with expert mental health specialists more quickly and easily. According to the findings, 71% of people with depression and 80% of those who do not have depression can be appropriately diagnosed. This study obtained 91% and 92% accuracy through the Random Forest (RF) and Extra Tree Classifier. But after applying the Receiver operating characteristic (ROC) curve, 79% accuracy was found on top of RF, 81% found on Extra Tree, and 82% recorded for the eXtreme Gradient Boosting (XGBoost) algorithm. Besides, several factors are identified in terms of predicting depression through statistical data analysis. Though the additional effort is needed to develop a more accurate model, this model can be adjustable in the healthcare sector for diagnosing depression.Keywords
Cite This Article
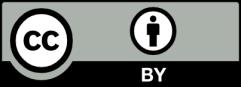