Open Access
ARTICLE
Rice Disease Diagnosis System (RDDS)
1 Maturi Venkata Subba Rao (MVSR) Engineering College, Hyderabad, Telangana, India
2 College of Computer and Information Sciences, Majmaah University, KSA
3 Indian Institute of Technology, Indian School of Mines (ISM), Dhanbad, Jharkhand, India
* Corresponding Author: Shirina Samreen. Email:
Computers, Materials & Continua 2022, 73(1), 1895-1914. https://doi.org/10.32604/cmc.2022.028504
Received 11 February 2022; Accepted 07 April 2022; Issue published 18 May 2022
Abstract
Hitherto, Rice (Oryza Sativa) has been one of the most demanding food crops in the world, cultivated in larger quantities, but loss in both quality and quantity of yield due to abiotic and biotic stresses has become a major concern. During cultivation, the crops are most prone to biotic stresses such as bacterial, viral, fungal diseases and pests. These stresses can drastically damage the crop. Lately and erroneously recognized crop diseases can increase fertilizers costs and major yield loss which results in high financial loss and adverse impact on nation’s economy. The proven methods of molecular biology can provide accurate detection of pathogenic factors, but these methods are not accessible to the majority of the farmers, needs high costs or resources, and require domain knowledge to implement. Expert’s field inspection report provides precise crop diagnosis but continuous field inspection over the remotely placed agriculture fields is not feasible. Therefore, cost effective approach for early detection of diseases can help farmers to take necessary steps in time to boost up the crop production. Precision agriculture makes use of decision support systems built using Machine Learning (ML) or Deep Learning (DL) approaches to cut down heavy costs. Timely crop diagnosis process can be automated with the involvement of Computer Vision, Image Processing and Deep Learning (DL) based methods for more precise prediction in less cost and time. Latest research shows that more accurate image classification can be implemented using Deep Learning based Convolutional Neural Network (CNN) model. In this paper, we have proposed an automated Rice Disease Diagnosis System (RDDS) for timely, more accurate and detailed crop disease diagnosis, which consisting of two modules, they are Leaf Disease Identification (LDI) module for disease detection and Infection Intensity Estimation (IIE) module for disease severity analysis. The LDI module is based on the proposed novel RDD_CNN model that classified the eight most harmful and commonly occurring diseases, it has obtained the best test accuracy of 98.47% when compared to its first three versions. And the IIE module is designed for estimating identified disease’s intensity in terms of extent and stage of infection providing detailed and overall diagnosis report specially designed for Brown Spot disease.Keywords
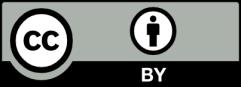