Open Access
ARTICLE
Optimal Deep Learning Driven Intrusion Detection in SDN-Enabled IoT Environment
1 Department of Information Systems, College of Computer Science, King Khalid University, Abha, Saudi Arabia
2 Department of Information Systems, College of Computer and Information Sciences, Princess Nourah bint Abdulrahman University, P.O. Box 84428, Riyadh, 11671, Saudi Arabia
3 SAUDI ARAMCO Cybersecurity Chair, Networks and Communications Department, College of Computer Science and Information Technology, Imam Abdulrahman Bin Faisal University, P.O. Box 1982, Dammam, 31441, Saudi Arabia
4 Prince Saud AlFaisal Institute for Diplomatic Studies, Riyadh, Saudi Arabia
5 Department of Computer Sciences, College of Computing and Information System, Umm Al-Qura University, Saudi Arabia
6 Department of Computer Science, Warwick University, Covetry, UK
7 Department of Computer Science, Faculty of Computers and Information Technology, Future University in Egypt New Cairo, 11835, Egypt
8 Department of Computer and Self Development, Preparatory Year Deanship, Prince Sattam bin Abdulaziz University, AlKharj, Saudi Arabia
* Corresponding Author: Manar Ahmed Hamza. Email:
Computers, Materials & Continua 2023, 74(3), 6587-6604. https://doi.org/10.32604/cmc.2023.034176
Received 07 July 2022; Accepted 03 October 2022; Issue published 28 December 2022
Abstract
In recent years, wireless networks are widely used in different domains. This phenomenon has increased the number of Internet of Things (IoT) devices and their applications. Though IoT has numerous advantages, the commonly-used IoT devices are exposed to cyber-attacks periodically. This scenario necessitates real-time automated detection and the mitigation of different types of attacks in high-traffic networks. The Software-Defined Networking (SDN) technique and the Machine Learning (ML)-based intrusion detection technique are effective tools that can quickly respond to different types of attacks in the IoT networks. The Intrusion Detection System (IDS) models can be employed to secure the SDN-enabled IoT environment in this scenario. The current study devises a Harmony Search algorithm-based Feature Selection with Optimal Convolutional Autoencoder (HSAFS-OCAE) for intrusion detection in the SDN-enabled IoT environment. The presented HSAFS-OCAE method follows a three-stage process in which the Harmony Search Algorithm-based FS (HSAFS) technique is exploited at first for feature selection. Next, the CAE method is leveraged to recognize and classify intrusions in the SDN-enabled IoT environment. Finally, the Artificial Fish Swarm Algorithm (AFSA) is used to fine-tune the hyperparameters. This process improves the outcomes of the intrusion detection process executed by the CAE algorithm and shows the work’s novelty. The proposed HSAFS-OCAE technique was experimentally validated under different aspects, and the comparative analysis results established the supremacy of the proposed model.Keywords
Cite This Article
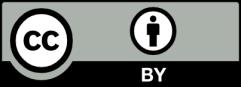