Open Access
ARTICLE
Machine Learning-based Electric Load Forecasting for Peak Demand Control in Smart Grid
1 Department of Electrical Engineering, Indian Institute of Technology (ISM), Dhanbad, 826004, India
2 Department of Nuclear Science & Technology, Pandit Deendayal Energy University, Gandhinagar, India
* Corresponding Author: Manish Kumar. Email:
Computers, Materials & Continua 2023, 74(3), 4785-4799. https://doi.org/10.32604/cmc.2022.032971
Received 03 June 2022; Accepted 27 August 2022; Issue published 28 December 2022
Abstract
Increasing energy demands due to factors such as population, globalization, and industrialization has led to increased challenges for existing energy infrastructure. Efficient ways of energy generation and energy consumption like smart grids and smart homes are implemented to face these challenges with reliable, cheap, and easily available sources of energy. Grid integration of renewable energy and other clean distributed generation is increasing continuously to reduce carbon and other air pollutants emissions. But the integration of distributed energy sources and increase in electric demand enhance instability in the grid. Short-term electrical load forecasting reduces the grid fluctuation and enhances the robustness and power quality of the grid. Electrical load forecasting in advance on the basic historical data modelling plays a crucial role in peak electrical demand control, reinforcement of the grid demand, and generation balancing with cost reduction. But accurate forecasting of electrical data is a very challenging task due to the nonstationary and nonlinearly nature of the data. Machine learning and artificial intelligence have recognized more accurate and reliable load forecasting methods based on historical load data. The purpose of this study is to model the electrical load of Jajpur, Orissa Grid for forecasting of load using regression type machine learning algorithms Gaussian process regression (GPR). The historical electrical data and whether data of Jajpur is taken for modelling and simulation and the data is decided in such a way that the model will be considered to learn the connection among past, current, and future dependent variables, factors, and the relationship among data. Based on this modelling of data the network will be able to forecast the peak load of the electric grid one day ahead. The study is very helpful in grid stability and peak load control management.Keywords
Cite This Article
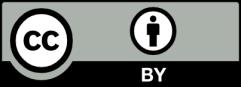