Open Access
ARTICLE
Digital Twin-Based Automated Fault Diagnosis in Industrial IoT Applications
1 Department of Information Technology, College of Computer and Information Sciences, Princess Nourah bint Abdulrahman University, P.O. Box 84428, Riyadh, 11671, Saudi Arabia
2 Department of Computer Science and Engineering, Faculty of Electronic Engineering, Menoufia University, Menouf, 32952, Egypt
3 Computer Science Department, Security Engineering Lab, Prince Sultan University, Riyadh, 11586, Saudi Arabia
4 Department of Electronics and Electrical Communications Engineering, Faculty of Electronic Engineering, Menoufia University, Menouf, 32952, Egypt
5 Department of Industrial Electronics and Control Engineering, Faculty of Electronic Engineering, Menoufia University, Menouf, 32952, Egypt
6 Department of Electrical Energy Engineering, College of Engineering & Technology, Arab Academy for Science Technology & Maritime Transport, Smart Village Campus, Egypt
* Corresponding Author: Walid El-Shafai. Email:
Computers, Materials & Continua 2023, 75(1), 183-196. https://doi.org/10.32604/cmc.2023.034048
Received 05 July 2022; Accepted 31 October 2022; Issue published 06 February 2023
Abstract
In recent years, Digital Twin (DT) has gained significant interest from academia and industry due to the advanced in information technology, communication systems, Artificial Intelligence (AI), Cloud Computing (CC), and Industrial Internet of Things (IIoT). The main concept of the DT is to provide a comprehensive tangible, and operational explanation of any element, asset, or system. However, it is an extremely dynamic taxonomy developing in complexity during the life cycle that produces a massive amount of engendered data and information. Likewise, with the development of AI, digital twins can be redefined and could be a crucial approach to aid the Internet of Things (IoT)-based DT applications for transferring the data and value onto the Internet with better decision-making. Therefore, this paper introduces an efficient DT-based fault diagnosis model based on machine learning (ML) tools. In this framework, the DT model of the machine is constructed by creating the simulation model. In the proposed framework, the Genetic algorithm (GA) is used for the optimization task to improve the classification accuracy. Furthermore, we evaluate the proposed fault diagnosis framework using performance metrics such as precision, accuracy, F-measure, and recall. The proposed framework is comprehensively examined using the triplex pump fault diagnosis. The experimental results demonstrated that the hybrid GA-ML method gives outstanding results compared to ML methods like Logistic Regression (LR), Naïve Bayes (NB), and Support Vector Machine (SVM). The suggested framework achieves the highest accuracy of 95% for the employed hybrid GA-SVM. The proposed framework will effectively help industrial operators make an appropriate decision concerning the fault analysis for IIoT applications in the context of Industry 4.0.Keywords
Cite This Article
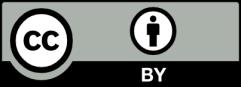