Open Access
ARTICLE
Federated Learning Based on Data Divergence and Differential Privacy in Financial Risk Control Research
School of Artificial Intelligence/School of Future Technology, Nanjing University of Information Science and Technology, Nanjing, 210044, China
* Corresponding Author: Wang Honglin. Email:
Computers, Materials & Continua 2023, 75(1), 863-878. https://doi.org/10.32604/cmc.2023.034879
Received 30 July 2022; Accepted 15 November 2022; Issue published 06 February 2023
Abstract
In the financial sector, data are highly confidential and sensitive, and ensuring data privacy is critical. Sample fusion is the basis of horizontal federation learning, but it is suitable only for scenarios where customers have the same format but different targets, namely for scenarios with strong feature overlapping and weak user overlapping. To solve this limitation, this paper proposes a federated learning-based model with local data sharing and differential privacy. The indexing mechanism of differential privacy is used to obtain different degrees of privacy budgets, which are applied to the gradient according to the contribution degree to ensure privacy without affecting accuracy. In addition, data sharing is performed to improve the utility of the global model. Further, the distributed prediction model is used to predict customers’ loan propensity on the premise of protecting user privacy. Using an aggregation mechanism based on federated learning can help to train the model on distributed data without exposing local data. The proposed method is verified by experiments, and experimental results show that for non-iid data, the proposed method can effectively improve data accuracy and reduce the impact of sample tilt. The proposed method can be extended to edge computing, blockchain, and the Industrial Internet of Things (IIoT) fields. The theoretical analysis and experimental results show that the proposed method can ensure the privacy and accuracy of the federated learning process and can also improve the model utility for non-iid data by 7% compared to the federated averaging method (FedAvg).Keywords
Cite This Article
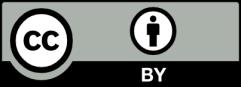