Open Access
ARTICLE
A COVID-19 Detection Model Based on Convolutional Neural Network and Residual Learning
1 Luoyang Normal University, Luoyang, 471934, China
2 Southwest Jiaotong University, Chengdu, 611756, China
* Corresponding Author: Bo Wang. Email:
Computers, Materials & Continua 2023, 75(2), 3625-3642. https://doi.org/10.32604/cmc.2023.036754
Received 11 October 2022; Accepted 15 January 2023; Issue published 31 March 2023
Abstract
A model that can obtain rapid and accurate detection of coronavirus disease 2019 (COVID-19) plays a significant role in treating and preventing the spread of disease transmission. However, designing such a model that can balance the detection accuracy and weight parameters of memory well to deploy a mobile device is challenging. Taking this point into account, this paper fuses the convolutional neural network and residual learning operations to build a multi-class classification model, which improves COVID-19 pneumonia detection performance and keeps a trade-off between the weight parameters and accuracy. The convolutional neural network can extract the COVID-19 feature information by repeated convolutional operations. The residual learning operations alleviate the gradient problems caused by stacking convolutional layers and enhance the ability of feature extraction. The ability further enables the proposed model to acquire effective feature information at a low cost, which can make our model keep small weight parameters. Extensive validation and comparison with other models of COVID-19 pneumonia detection on the well-known COVIDx dataset show that (1) the sensitivity of COVID-19 pneumonia detection is improved from 88.2% (non-COVID-19) and 77.5% (COVID-19) to 95.3% (non-COVID-19) and 96.5% (COVID-19), respectively. The positive predictive value is also respectively increased from 72.8% (non-COVID-19) and 89.0% (COVID-19) to 88.8% (non-COVID-19) and 95.1% (COVID-19). (2) Compared with the weight parameters of the COVIDNet-small network, the value of the proposed model is 13 M, which is slightly higher than that (11.37 M) of the COVIDNet-small network. But, the corresponding accuracy is improved from 85.2% to 93.0%. The above results illustrate the proposed model can gain an efficient balance between accuracy and weight parameters.Keywords
Cite This Article
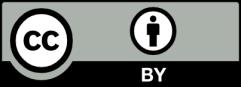