Open Access
ARTICLE
Research on Human Activity Recognition Algorithm Based on LSTM-1DCNN
1 School of Automation, Guangxi University of Science and Technology, Liuzhou, 545006, China
2 Student Mental Health Education Center, Guangxi University of Science and Technology, Liuzhou, 545006, China
3 Artificial Intelligence Research Institute, China University of Mining and Technology, Xuzhou, 221116, China
* Corresponding Authors: Xiaoling Wang. Email: ; Yutong Luo. Email:
Computers, Materials & Continua 2023, 77(3), 3325-3347. https://doi.org/10.32604/cmc.2023.040528
Received 21 March 2023; Accepted 20 October 2023; Issue published 26 December 2023
Abstract
With the rapid advancement of wearable devices, Human Activities Recognition (HAR) based on these devices has emerged as a prominent research field. The objective of this study is to enhance the recognition performance of HAR by proposing an LSTM-1DCNN recognition algorithm that utilizes a single triaxial accelerometer. This algorithm comprises two branches: one branch consists of a Long and Short-Term Memory Network (LSTM), while the other parallel branch incorporates a one-dimensional Convolutional Neural Network (1DCNN). The parallel architecture of LSTM-1DCNN initially extracts spatial and temporal features from the accelerometer data separately, which are then concatenated and fed into a fully connected neural network for information fusion. In the LSTM-1DCNN architecture, the 1DCNN branch primarily focuses on extracting spatial features during convolution operations, whereas the LSTM branch mainly captures temporal features. Nine sets of accelerometer data from five publicly available HAR datasets are employed for training and evaluation purposes. The performance of the proposed LSTM-1DCNN model is compared with five other HAR algorithms including Decision Tree, Random Forest, Support Vector Machine, 1DCNN, and LSTM on these five public datasets. Experimental results demonstrate that the F1-score achieved by the proposed LSTM-1DCNN ranges from 90.36% to 99.68%, with a mean value of 96.22% and standard deviation of 0.03 across all evaluated metrics on these five public datasets-outperforming other existing HAR algorithms significantly in terms of evaluation metrics used in this study. Finally the proposed LSTM-1DCNN is validated in real-world applications by collecting acceleration data of seven human activities for training and testing purposes. Subsequently, the trained HAR algorithm is deployed on Android phones to evaluate its performance. Experimental results demonstrate that the proposed LSTM-1DCNN algorithm achieves an impressive F1-score of 97.67% on our self-built dataset. In conclusion, the fusion of temporal and spatial information in the measured data contributes to the excellent HAR performance and robustness exhibited by the proposed 1DCNN-LSTM architecture.Keywords
Cite This Article
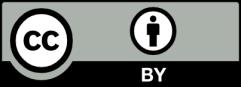