Open Access
ARTICLE
Machine Learning Techniques Using Deep Instinctive Encoder-Based Feature Extraction for Optimized Breast Cancer Detection
1 Department of Computer Science, Gautam Buddha University, Greater Noida, Uttar Pradesh, India
2 College of Computing and Informatics, Saudi Electronic University, Riyadh, 11673, Saudi Arabia
3 School of Computer Science, Shri Vaishno Devi University, Katra, India
* Corresponding Authors: Mohammad Khalid Imam Rahmani. Email: ; Rania Almajalid. Email:
Computers, Materials & Continua 2024, 78(2), 2441-2468. https://doi.org/10.32604/cmc.2024.044963
Received 12 August 2023; Accepted 22 December 2023; Issue published 27 February 2024
Abstract
Breast cancer (BC) is one of the leading causes of death among women worldwide, as it has emerged as the most commonly diagnosed malignancy in women. Early detection and effective treatment of BC can help save women’s lives. Developing an efficient technology-based detection system can lead to non-destructive and preliminary cancer detection techniques. This paper proposes a comprehensive framework that can effectively diagnose cancerous cells from benign cells using the Curated Breast Imaging Subset of the Digital Database for Screening Mammography (CBIS-DDSM) data set. The novelty of the proposed framework lies in the integration of various techniques, where the fusion of deep learning (DL), traditional machine learning (ML) techniques, and enhanced classification models have been deployed using the curated dataset. The analysis outcome proves that the proposed enhanced RF (ERF), enhanced DT (EDT) and enhanced LR (ELR) models for BC detection outperformed most of the existing models with impressive results.Keywords
Cite This Article
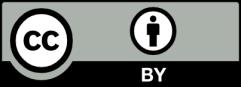