Open Access
ARTICLE
Workout Action Recognition in Video Streams Using an Attention Driven Residual DC-GRU Network
1 Department of Computer Science and Technology, Indian Institute of Engineering Science and Technology, Shibpur, Howrah, 711103, India
2 Faculty of Information Technology, Haiphong University, Haiphong, 180000, Vietnam
* Corresponding Author: Arnab Dey. Email:
(This article belongs to the Special Issue: Advances and Applications in Signal, Image and Video Processing)
Computers, Materials & Continua 2024, 79(2), 3067-3087. https://doi.org/10.32604/cmc.2024.049512
Received 09 January 2024; Accepted 09 April 2024; Issue published 15 May 2024
Abstract
Regular exercise is a crucial aspect of daily life, as it enables individuals to stay physically active, lowers the likelihood of developing illnesses, and enhances life expectancy. The recognition of workout actions in video streams holds significant importance in computer vision research, as it aims to enhance exercise adherence, enable instant recognition, advance fitness tracking technologies, and optimize fitness routines. However, existing action datasets often lack diversity and specificity for workout actions, hindering the development of accurate recognition models. To address this gap, the Workout Action Video dataset (WAVd) has been introduced as a significant contribution. WAVd comprises a diverse collection of labeled workout action videos, meticulously curated to encompass various exercises performed by numerous individuals in different settings. This research proposes an innovative framework based on the Attention driven Residual Deep Convolutional-Gated Recurrent Unit (ResDC-GRU) network for workout action recognition in video streams. Unlike image-based action recognition, videos contain spatio-temporal information, making the task more complex and challenging. While substantial progress has been made in this area, challenges persist in detecting subtle and complex actions, handling occlusions, and managing the computational demands of deep learning approaches. The proposed ResDC-GRU Attention model demonstrated exceptional classification performance with 95.81% accuracy in classifying workout action videos and also outperformed various state-of-the-art models. The method also yielded 81.6%, 97.2%, 95.6%, and 93.2% accuracy on established benchmark datasets, namely HMDB51, Youtube Actions, UCF50, and UCF101, respectively, showcasing its superiority and robustness in action recognition. The findings suggest practical implications in real-world scenarios where precise video action recognition is paramount, addressing the persisting challenges in the field. The WAVd dataset serves as a catalyst for the development of more robust and effective fitness tracking systems and ultimately promotes healthier lifestyles through improved exercise monitoring and analysis.Keywords
Cite This Article
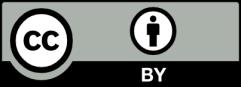