Open Access
ARTICLE
Arrhythmia Detection by Using Chaos Theory with Machine Learning Algorithms
1 Math and Computer Science Department, Faculty of Science, Menoufia University, Shebin El-kom, Egypt
2 College of Engineering, Computer Science Department, Effat University, Jeddah, Kingdom of Saudi Arabia
3 Department of Computer Engineering, College of Computer Science, King Khalid University, Abha, 61421, Saudi Arabia
4 Computer Science and Engineering Department, Faculty of Electronic Engineering, Menoufia University, Shebin El-kom, Egypt
5 Climate Change Information Center and Expert Systems, Agriculture Research Center, Giza, Egypt
* Corresponding Author: Maie Aboghazalah. Email:
Computers, Materials & Continua 2024, 79(3), 3855-3875. https://doi.org/10.32604/cmc.2023.039936
Received 25 February 2023; Accepted 25 July 2023; Issue published 20 June 2024
Abstract
Heart monitoring improves life quality. Electrocardiograms (ECGs or EKGs) detect heart irregularities. Machine learning algorithms can create a few ECG diagnosis processing methods. The first method uses raw ECG and time-series data. The second method classifies the ECG by patient experience. The third technique translates ECG impulses into Q waves, R waves and S waves (QRS) features using richer information. Because ECG signals vary naturally between humans and activities, we will combine the three feature selection methods to improve classification accuracy and diagnosis. Classifications using all three approaches have not been examined till now. Several researchers found that Machine Learning (ML) techniques can improve ECG classification. This study will compare popular machine learning techniques to evaluate ECG features. Four algorithms—Support Vector Machine (SVM), Decision Tree, Naive Bayes, and Neural Network—compare categorization results. SVM plus prior knowledge has the highest accuracy (99%) of the four ML methods. QRS characteristics failed to identify signals without chaos theory. With 99.8% classification accuracy, the Decision Tree technique outperformed all previous experiments.Keywords
Cite This Article
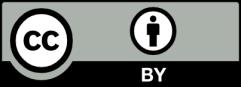