Open Access
ARTICLE
Regularised Layerwise Weight Norm Based Skin Lesion Features Extraction and Classification
School of Computer Science of Engineering, Bharathidasan University, Tiruchirapalli, 620023, India
* Corresponding Author: S. Gopikha. Email:
Computer Systems Science and Engineering 2023, 44(3), 2727-2742. https://doi.org/10.32604/csse.2023.028609
Received 13 February 2022; Accepted 07 April 2022; Issue published 01 August 2022
Abstract
Melanoma is the most lethal malignant tumour, and its prevalence is increasing. Early detection and diagnosis of skin cancer can alert patients to manage precautions and dramatically improve the lives of people. Recently, deep learning has grown increasingly popular in the extraction and categorization of skin cancer features for effective prediction. A deep learning model learns and co-adapts representations and features from training data to the point where it fails to perform well on test data. As a result, overfitting and poor performance occur. To deal with this issue, we proposed a novel Consecutive Layerwise weight Constraint MaxNorm model (CLCM-net) for constraining the norm of the weight vector that is scaled each time and bounding to a limit. This method uses deep convolutional neural networks and also custom layer-wise weight constraints that are set to the whole weight matrix directly to learn features efficiently. In this research, a detailed analysis of these weight norms is performed on two distinct datasets, International Skin Imaging Collaboration (ISIC) of 2018 and 2019, which are challenging for convolutional networks to handle. According to the findings of this work, CLCM-net did a better job of raising the model’s performance by learning the features efficiently within the size limit of weights with appropriate weight constraint settings. The results proved that the proposed techniques achieved 94.42% accuracy on ISIC 2018, 91.73% accuracy on ISIC 2019 datasets and 93% of accuracy on combined dataset.Keywords
Cite This Article
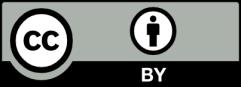