Open Access
ARTICLE
A Framework of Deep Learning and Selection-Based Breast Cancer Detection from Histopathology Images
1 Department of Computer Science, COMSATS University Islamabad, Wah Campus, Wah Cantt, Pakistan
2 Computer Sciences Department, College of Computer and Information Sciences, Princess Nourah Bint Abdulrahman University (PNU), P.O. BOX 84428, Riyadh, 11671, Saudi Arabia
3 College of Computer Engineering and Science, Prince Sattam Bin Abdulaziz University, Al-Kharaj, 11942, Saudi Arabia
4 Department of Computer Science, Hanyang University, Seoul, 04763, Korea
5 Center for Computational Social Science, Hanyang University, Seoul, 04763, Korea
* Corresponding Author: Byoungchol Chang. Email:
Computer Systems Science and Engineering 2023, 45(2), 1001-1016. https://doi.org/10.32604/csse.2023.030463
Received 26 March 2022; Accepted 17 May 2022; Issue published 03 November 2022
Abstract
Breast cancer (BC) is a most spreading and deadly cancerous malady which is mostly diagnosed in middle-aged women worldwide and effecting beyond a half-million people every year. The BC positive newly diagnosed cases in 2018 reached 2.1 million around the world with a death rate of 11.6% of total cases. Early diagnosis and detection of breast cancer disease with proper treatment may reduce the number of deaths. The gold standard for BC detection is biopsy analysis which needs an expert for correct diagnosis. Manual diagnosis of BC is a complex and challenging task. This work proposed a deep learning-based (DL) solution for the early detection of this deadly disease from histopathology images. To evaluate the robustness of the proposed method a large publically available breast histopathology image database containing a total of 277524 histopathology images is utilized. The proposed automatic diagnosis of BC detection and classification mainly involves three steps. Initially, a DL model is proposed for feature extraction. Secondly, the extracted feature vector (FV) is passed to the proposed novel feature selection (FS) framework for the best FS. Finally, for the classification of BC into invasive ductal carcinoma (IDC) and normal class different machine learning (ML) algorithms are used. Experimental outcomes of the proposed methodology achieved the highest accuracy of 92.7% which shows that the proposed technique can successfully be implemented for BC detection to aid the pathologists in the early and accurate diagnosis of BC.Keywords
Cite This Article
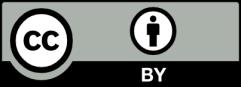