Open Access
ARTICLE
FST-EfficientNetV2: Exceptional Image Classification for Remote Sensing
School of Geography Science and Tourism, Hunan University of Arts and Science, Changde, 415000, China
* Corresponding Author: Huaxiang Song. Email:
Computer Systems Science and Engineering 2023, 46(3), 3959-3978. https://doi.org/10.32604/csse.2023.038429
Received 12 December 2022; Accepted 02 February 2023; Issue published 03 April 2023
Abstract
Recently, the semantic classification (SC) algorithm for remote sensing images (RSI) has been greatly improved by deep learning (DL) techniques, e.g., deep convolutional neural networks (CNNs). However, too many methods employ complex procedures (e.g., multi-stages), excessive hardware budgets (e.g., multi-models), and an extreme reliance on domain knowledge (e.g., handcrafted features) for the pure purpose of improving accuracy. It obviously goes against the superiority of DL, i.e., simplicity and automation. Meanwhile, these algorithms come with unnecessarily expensive overhead on parameters and hardware costs. As a solution, the author proposed a fast and simple training algorithm based on the smallest architecture of EfficientNet version 2, which is called FST-EfficientNet. The approach employs a routine transfer learning strategy and has fast training characteristics. It outperforms all the former methods by a 0.8%–2.7% increase in accuracy. It does, however, use a higher testing resolution of 5122 and 6002, which results in high consumption of graphics processing units (GPUs). As an upgrade option, the author proposes a novel and more efficient method named FST-EfficientNetV2 as the successor. The new algorithm still employs a routine transfer learning strategy and maintains fast training characteristics. But a set of crucial algorithmic tweaks and hyperparameter re-optimizations have been updated. As a result, it achieves a noticeable increase in accuracy of 0.3%–1.1% over its predecessor. More importantly, the algorithm’s GPU costs are reduced by 75%–81%, with a significant reduction in training time costs of 60%–80%. The results demonstrate that an efficient training optimization strategy can significantly boost the CNN algorithm's performance for RSI-SC. More crucially, the results prove that the distribution shift introduced by data augmentation (DA) techniques is vital to the method’s performance for RSI-SC, which has been ignored to date. These findings may help us gain a correct understanding of the CNN algorithm for RSI-SC.Keywords
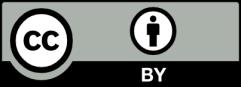