Open Access
ARTICLE
Adaptive Kernel Firefly Algorithm Based Feature Selection and Q-Learner Machine Learning Models in Cloud
1
Department of Information Technology, Sri Ramakrishna Engineering College, Coimbatore, Tamilnadu, India
2
Department of Computer Science & Engineering, RVS College of Engineering and Technology, Coimbatore, Tamilnadu, India
* Corresponding Author: I. Mettildha Mary. Email:
Computer Systems Science and Engineering 2023, 46(3), 2667-2685. https://doi.org/10.32604/csse.2023.031114
Received 11 April 2022; Accepted 21 July 2022; Issue published 03 April 2023
Abstract
CC’s (Cloud Computing) networks are distributed and dynamic as signals appear/disappear or lose significance. MLTs (Machine learning Techniques) train datasets which sometime are inadequate in terms of sample for inferring information. A dynamic strategy, DevMLOps (Development Machine Learning Operations) used in automatic selections and tunings of MLTs result in significant performance differences. But, the scheme has many disadvantages including continuity in training, more samples and training time in feature selections and increased classification execution times. RFEs (Recursive Feature Eliminations) are computationally very expensive in its operations as it traverses through each feature without considering correlations between them. This problem can be overcome by the use of Wrappers as they select better features by accounting for test and train datasets. The aim of this paper is to use DevQLMLOps for automated tuning and selections based on orchestrations and messaging between containers. The proposed AKFA (Adaptive Kernel Firefly Algorithm) is for selecting features for CNM (Cloud Network Monitoring) operations. AKFA methodology is demonstrated using CNSD (Cloud Network Security Dataset) with satisfactory results in the performance metrics like precision, recall, F-measure and accuracy used.Keywords
Cite This Article
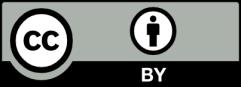