Open Access
ARTICLE
Deep Belief Network for Lung Nodule Segmentation and Cancer Detection
Rajalakshmi Engineering College, Thandalam, Chennai, 602105, Tamil Nadu, India
* Corresponding Author: Sindhuja Manickavasagam. Email:
Computer Systems Science and Engineering 2023, 47(1), 135-151. https://doi.org/10.32604/csse.2023.030344
Received 24 March 2022; Accepted 30 June 2022; Issue published 26 May 2023
Abstract
Cancer disease is a deadliest disease cause more dangerous one. By identifying the disease through Artificial intelligence to getting the mage features directly from patients. This paper presents the lung knob division and disease characterization by proposing an enhancement calculation. Most of the machine learning techniques failed to observe the feature dimensions leads inaccuracy in feature selection and classification. This cause inaccuracy in sensitivity and specificity rate to reduce the identification accuracy. To resolve this problem, to propose a Chicken Sine Cosine Algorithm based Deep Belief Network to identify the disease factor. The general technique of the created approach includes four stages, such as pre-processing, segmentation, highlight extraction, and the order. From the outset, the Computerized Tomography (CT) image of the lung is taken care of to the division. When the division is done, the highlights are extricated through morphological factors for feature observation. By getting the features are analysed and the characterization is done dependent on the Deep Belief Network (DBN) which is prepared by utilizing the proposed Chicken-Sine Cosine Algorithm (CSCA) which distinguish the lung tumour, giving two classes in particular, knob or non-knob. The proposed system produce high performance as well compared to the other system. The presentation assessment of lung knob division and malignant growth grouping dependent on CSCA is figured utilizing three measurements to be specificity, precision, affectability, and the explicitness.Keywords
Cancer is one of the risky disorders brought about by the vast majority of the living creatures is disease. Most of the existing methods failed to detect the disease level prone factor, because of identifying objective factors are non-related feature during image processing The problem arise from improper pre-processing to attain noise, error rate, segmentation failures, region specification, threshold variation factors are degrade the identification accuracy Most developed Existing machine learning techniques like K-Nearest Neighbour (KNN) is used for finding the relation between the diseases and its treatments. By identifying the feature observation, the Lung malignancy is the sort of disease that starts in lungs. Lungs are considered as the most critical organs in our respiratory framework. It is accounted for by the World Health Organization (WHO) that in 2012, this ailment had caused 1.59 million people’s in 2015, around 158080 passing’s happened. The endurance rate relies upon the way that the treatment ought to be begun at beginning phases. It implies on the off chance that it isn’t treated at beginning phases, at that point the odds of endurance are less. These days, the death rate is expanded in light of the fact that it is unpredictable for recognizing threatening lung knobs at the previous stage. The estimation of size might be reliable and precise for empowering the evaluation of progress in knob at constrained time span. The time stretch may differ depending on certain clinical conditions [1]. A portion of the lung malignancies, particularly adenocarcinomas are more likely than other kinds of lung disease, since it might spread the malignant growth cells outside the locale of the chest and become dispersed fundamentally, regardless of whether the tumour is extremely little. The size of the knob is multiplied when it estimates 6.3 mm in breadth [2]. On the off chance that the size of the knob is unpredictable, at that point it is intricate for perceiving outwardly. Moreover, the momentary information on the tumour reaction is vital for settling on tolerant explicit treatment choices for better clinical results [3].
The Computed Tomography (CT) is most regularly utilized imaging strategies in thoracic radiology [4]. The lung knob estimations are made with CT for checking the tumour for treatment reasons. More up to date age focused on treatments have started to show clinical guarantee in lung disease. Be that as it may, huge numbers of these operators are cytostatic and neglected to influence tumour shrinkage, or may surrender the injury shrinkage than the past ages of cytotoxic chemotherapy [5]. The fundamental point of lung tumour location is to identify the lung malignant growth in a previous stage and to lessen the lung disease passing. Consequently, the danger decision by the pathologist doesn’t imply that the patient is advantaged from the treatment of the knob. These days, both the picture investigation, and picture obtaining approaches permit the semi robotized tumour division and extraction of a few highlights from images [6]. The information from the image is used for developing prescient and unmistakable models compared to the picture related highlights to quality protein marks or phenotypes that incorporate clinical information or organic for significant prognostic, analytic, or the prescient data [7]. An advancing study showed that early tumor volume change is more fragile than early EGFR (epidermal growth factor receptor) change estimate change expected in non-small cell lung threatening growth. The potential employment volumetric CT is capable of playing in dynamically helpful and precise treatment response evaluation is by and by under genuine assessment.
The lung knob division is noteworthy for two unique frameworks, similar to PC helped determination using Computer Aided Design (CAD), and Content Based Medical Image Recovery (CBMIR) so as to forestall or find the sores. The underlying CAD framework is used for distinguishing and classifying the knobs as amiable or the harmful [8]. The auxiliary CBMIR distinguishes the arrangement of pictures from the database, which have the same qualities to the lung knob. The fundamental point of this framework is to separate the favourable and harmful sores for better analysis. Various classifiers are utilized for characterizing the harmful and benevolent lung knobs, such as Artificial Neural Network (ANN), Linear Discriminant Analysis (LDA), and the Support Vector Machine (SVM). Many new Deep learning algorithms are proving good in the medical field to diagnose the diseases in early stages [9]. The vast majority of the classifiers require information marks for preparation; however it is over the top expensive for producing named information in radiology. All in all, a portion of the solo calculations are utilized to characterize unlabelled information [10]. Convolution Neural Networks (CNN) to take in the various levelled portrayals from the unlabelled pictures. The main contribution and motivation of the research is to improve the detection accuracy based on Chicken Sine Cosine Algorithm based Deep Belief Network for Lung Nodule Segmentation and Cancer Detection. By susytin the best feature observation through image processing and classy the result to increase the sensitivity and specificity.
The organisation of the paper in introduction covers the problem statements and its various approaches. The Section 2 contains the literature review, Section 3 descripes the proposed solution. Section 4 describes the result and discussion and Section 5 concludes the performance of the proposed system.
This segment portrays an audit of the writing on different existing lung knob division, and malignant growth discovery. These examination papers are taken and assessed by the ongoing distributed years dependent on lung knob division, and malignant growth location strategies.
This research proposes a novel Chicken sine-cosine algorithm and modified Spatial Kernelized fuzzy c-means (MSFCM) and ensemble learning to segment and classify the lung tumor Developing an exact approval system in the division of lung knob research is exceptionally testing a direct result of manual sore molding utilized by the spectators for planning Ground Truth (GT) divisions as work escalated, making it complex for making colossal GT datasets. In Spatial Multi-Kernel FCM, Hybrid clever procedure is created for lung tumor analysis from CT pictures [11]. Here, the presentation was discovered better, yet neglected to analyze 3D preparing rather than 2D for improving the truth of the created technique. Lung knob division on the chest CT filters is hard for successful CAD respiratory infections, similar to lung malignancy [12]. Here, the division precision was discovered better, yet the expense for division id high. The significant test for lung knob division is merge rules However, the shape imperative is thought of, unpredictable molded knobs stay critical for preparing in light of the fact that shape theory is disregarded. An ordered record of the inception of malignant growth registers and uncommon studies to determine disease occurrence, with subtleties of the data requested in each occasion, is introduced. Comparisons made between rates obtained for lung cancer in 12 regions where it is to be accepted that 90% or a higher amount of all malignancies happening has been recorded [13]. The standard interims between the beginning of first side effects and enlistment at death for diseases of various locales considered, and the extents of cases recorded upon death endorsements just, and it is recommended that exactness old enough explicit rates might be improved by utilizing the age at the beginning of first indications as a basis rather than age at registration or death, and that “ inception rates” so derived are more meaningful.
The essential objective of this exploration is to structure and present a methodology for lung knob division and malignant growth identification by proposing a streamlining calculation. The way of ensemble the KNN and Decision Tree was used in classification of medical data. The general method of the proposed approach includes four stages, as pre-preparing, lung knob division, highlight extraction and grouping. At first, the CT lung picture will be exposed to the pre-handling. After pre-handling, the lung knob division will be completely dependent on adjusted Spatial Multi-Kernel and Fuzzy C-Means (FCM) in which a few piece capacities, as Gaussian, exponential, and digressive will be used. After the division of lung knobs, the element extraction will be performed dependent on Morphological and factual highlights, similar to elasticity, entropy, roundness, differentiate, homogeneity, circularity, vitality, connection, standard deviation, mean, Euler number, significant pivot length, direction, robustness, and the surface highlights, similar to LOOP and Local Gradient Pattern (LGP) Fig. 1.
Figure 1: Framework design of lung nodule segmentation using deep belief network
At long last, the order will be done dependent on the extricated highlights utilizing Deep Belief Network (DBN) that are prepared utilizing the proposed Chicken-Sine Cosine Algorithm (CSCA). The proposed CSCSA is planned by joining Chicken Swarm Optimization and Sine Cosine Algorithm for lung tumour identification, giving two classes, which incorporates non-knob, and knob. The square chart of the lung tumour division and malignant growth identification approach utilizing the proposed CSCA is appeared.
Adenocarcinoma In Situ (AIS) is a pre-intrusive injury in the lung and a subtype of lung adenocarcinoma. The patients with AIS can be quieted by dismissing the injury. Inquisitively, patients with noticeable lung adenocarcinoma have a shocking 5-year duration rate. AIS can outline into noticeable lung adenocarcinoma. The evaluation and relationship of AIS and noticeable lung adenocarcinoma at the genomic level can extend our comprehension of the instrument’s crucial lung perilous advancement improvement.
As of now, I of 61 Lung Adenocarcinoma (LUAD) noticeable express differentially passed on attributes, including nine long non-coding RNAs (lncRNAs) given RNA (Ribonucleic acid)sequencing procedures (RNA-seq) information from standard, AIS, and interfering tissue tests. These qualities showed concordant Differential Enunciation (DE) structures in the free stage III LUAD tissues acquired from The Cancer Genome Atlas (TCGA). For individual conspicuous unequivocal attributes, we amassed sub frameworks utilizing the Genetic Algorithm (GA) given protein-protein composed endeavours, protein-DNA (deoxyribonucleic acid.) affiliations, and lncRNA rules. Our evaluation perceived an aggregate of 19 centres sub-sorts that included intrusive express attributes and, in any case, one putative lung risk driver quality. Convenient appraisal of the centres sub frameworks uncovered their improvement in known pathways and regular advances in danger for tumor headway and attack, including the VEGF hailing pathway and the negative principle of cell progression.
The Computed Tomography (CT) is the most ordinarily utilized imaging system in thoracic radiology. The lung knob estimations are made with CT for observing the tumour for treatment reasons. More current age focused treatments have started to show clinical guarantee in lung malignancy. Huge numbers of these specialists are cytostatic and neglected to influence tumor shrinkage, or may abandon the injury shrinkage than the past ages of cytotoxic chemotherapy. The fundamental point of lung tumour identification is to distinguish the lung malignancy in a prior stage and to decrease the lung disease passing.
Consequently, the threat decision by the pathologist does not imply that the patient is an advantage from the treatment of the knob. These days, both the picture investigation and picture obtaining approaches permit the semi-mechanized tumour division and extraction of a few highlights from pictures. The information from the picture is used for developing prescient and enlightening models compared to the picture related highlights to quality protein marks or phenotypes that incorporate clinical information or natural for important prognostic, symptomatic, or the prescient data. An advancing report indicated that new change in tumor volume is highly touchy than the early partition across change at Epidermal Growth Factor Receptor (EGFR). change, imagining in non-little cell lung hurtful turn of events. The latent occupation volumetric CT may play in progressively supportive and definite treatment reaction evaluation is beginning at now under concentrated appraisal.
The lung knob division is vast for two unique frameworks, similar to PC supported finding (CAD), and substance-based clinical picture recovery (CBMIR) to forestall or determination of the sores. The underlying CAD framework is used for recognizing and ordering the knobs as considerate or threatening. The optional CBMIR recognizes the arrangement of pictures from the database, which have the same attributes to the lung knob. The primary point of this framework is to separate the benevolent and dangerous sores for better analysis. Various classifiers are utilized for grouping the dangerous and considerate lung knobs, like ANN, LDA, and SVM. A large portion of the classifiers require information names for preparing reasons. However, it is pricey for creating named information in radiology. When all is said in done, a portion of the unaided calculations is utilized to arrange unlabelled information.
The dataset used for this research is Lung Image Database Consortium Image Collection (LIDC-IDRI) consists of thoracic Computed Tomography (CT) Scans for diagnostic and lung cancer screening with marked-up annotated lesions. It is an international web accessible resource for Computer Assisted Diagnostic (CAD) methods for the detection and diagnosis of lung cancer. In this dataset there are about 1018 cases with each subject includes a clinical thoracic CT scan and an associated XML file that records the results of a two-phase image annotation process.
From the earliest point, a soft II figuring is used in the proposed method to enhance harsh CT images. By that point, a novel division calculation subject to cushy c-infers bunching, called Modified Spatial Kernelized Fuzzy C-Means (MSFCM) gathering, is offered so as to accomplish different delineation of lung regions through a movement approach. Next, handle applicants are perceived amongst every single accessible thing in the lung zones by a morphological system. This is followed by evacuating fundamental genuine and morphological highlights from such handle all in all, an outfit of three classification containing Multi-Layer Perceptron (MLP), KNN, SVM is utilized for the real finding and picking if the handle candidate is handle (harm) or non-handle (prosperity).
Considerably more essentially, extraordinary obliging execution estimations in clinical applications including precision, affectability, unequivocality, turmoil arrange, likewise as the space under the Receiver Operating Characteristic (ROC) contort are figured. The got outcomes admit the promising demonstration of the proposed mixture procedure in got a handle on aspiratory handles finding.
From Fig. 1, the Artificial Intelligence application model is planned utilizing Machine Learning calculations for forecast of results. The accompanying stages are utilized to execute this calculation. First stage information is procured from Wisconsin lung malignant growth database and pre-prepared utilizing the managed calculations as demonstrated Fig. 2.
Figure 2: Lung nodule images pre-processing sample dataset
In Fig. 2, a sample dataset of CT lung images are processed and checking the presence of cancer nodes. In this paper we have utilised more than 1000 images for classification of lung nodules. The testing and training was carried out through dependencies. The images are taken from all the aged people of both male and female. We have noticed every image carefully to identify for which age it was occurring and also we noticed the cause for this happening. Here the lung nodule is taken as one of the feature extraction attributes to classify these images. vascular endothelial growth factor (VEGF) Using the above images dataset, we have prepared a table to process the dataset into a system for better segmentation to predict better results in feature. We have indicated pulmonary areas with colours in above images for better view.
From the above Tables 1 and 2, we have arranged our gathered data from all over the world (using Wisconsin dataset and kaggle dataset), using the patient characteristics. These characteristics are used to design pre-processing data and converted into .csv files to process into AI system models.
3.3 Lung Nodule Segmentation- Kernel Functions
In Second phase lung nodule segmentation, kernel functions are used to separate the labelled and unlabelled data using feature extraction and feature selection methods like Gaussian, exponential, tangential and Modified Spatial Kernelized Fuzzy C-Means (MSKFCM). This kernel reduces the dimensionality between the dataset attributes and produces the only defined variables. The defined variables are calculated using attribute bias and variance values. The purpose of finding these values is to know how the designed model is producing the accuracy. So all the processed dataset variables are arranged in a matrix and avoiding the unwanted elements to reduce the cost function and search space.
The Gaussian part (assortment of irregular factors ordered by time or space) is characterized in 1-D, 2D and N-D individually as, for discretionary genuine constants a, b and non-zero c. The boundary is the stature of the bend’s pinnacle, b is the situation of the focal point of the pinnacle and c controls the width of the “ringer”. From Fig. 3, Gaussian capacities are frequently used to speak to the likelihood thickness capacity of an ordinarily disseminated irregular variable with expected worth μ = b and fluctuation σ2 = c2. For this situation, the Gaussian is of the structure given in (1).
Figure 3: Normalized gaussian form with expected value μ and variance σ2, b = μ, c = σ
The Exponential kernel function covariance function is defined by (2).
In the investigation of ANNs, the Neural Tangential Kernel (NTK) is a portion which portrays the development of profound counterfeit neural systems during their preparation by inclination plunge. It permits ANNs to be contemplated utilizing hypothetical instruments from Kernel Methods. For most normal neural system structures, in the constraint of huge layer width the NTK gets consistent. This empowers straightforward shut structure articulations to be made about neural system forecasts, preparing elements, speculation, and misfortune surfaces. For instance, it ensures that enormous enough ANNs unite to a worldwide minimum when prepared to limit an observational misfortune. The NTK of enormous width systems is likewise identified with a few other huge widths cut off points of neural systems and the equation given as (3).
Let’s bring in some notation:
● Call the neural network function
● In this 1-D example, our dataset will just be points
For learning the network, we’ll take a simple approach again: just perform full-batch gradient descent on the least squares loss. Now, you might be familiar with writing this loss
But we can simplify this using some vector notation.
● First, stack all the output dataset values
● Similarly, stack all the model outputs for each input,
● We basically have
So, our loss simplifies to this:
Now, from Fig. 4, we won’t be changing the dataset size NN anywhere, and it’s an unnecessary constant in the loss expression. So, we can just drop it without affecting any of the further results, while making the algebra look less cluttered
Figure 4: Neural tangential vector notation graph
From the Fig. 5, if the weights double, this relative change will be 2 irrespective of the size of the hidden layers. Now if we plot this for the nets we trained above:
Figure 5: Neural tangential training loss and relative change in weights
Fuzzy Clustering (moreover insinuated as fragile gathering or sensitive k-suggests) each data point can have a spot with more than one gathering. Gathering or bundle assessment incorporates consigning data centres to packs with the ultimate objective that things in a comparative gathering are as equivalent as could sensibly be normal, while things having a spot with different gatherings are as dissimilar as could sensibly be normal. Gatherings are recognized by methods for closeness measures. These likeness estimates join division, accessibility, and force. Particular indicators of similarity can be chosen based on the data or the application.
In non-fuzzy clustering (in any case called hard gathering), data is detached into specific packs, where each data point can simply have a spot with correctly one gathering. In cushioned gathering, data centres can possibly have a spot with various bundles. For example, a grape can be purple or green (hard packing), yet a grape can moreover be purple AND green (cushy gathering). Here, the grape can be purple with a particular goal in mind similarly as green with a particular goal in mind. Instead of the grape having a spot with green [green = 1] and not purple [purple = 0], the grape can have a spot with green [green = 0.5] and purple [red = 0.5]. These values are normalized some place in the scope of 0 and 1; regardless, they don’t address probabilities, so the two characteristics don’t need to mean 1.
The mostly used Fuzzy Clustering Algorithm is the Fuzzy C-Means Clustering Algorithm. The fuzzy c-means algorithm is in a general sense equivalent to the k-means algorithm:
● Choose different bundles.
● Each data point is to be assigned with coefficients self-assertively for being in the packs.
● Until the estimation has joined together repeat the process. (that is, the coefficients’ change between two emphases is near ε, the given affectability limit).
● Compute the centroid for each bundle (exhibited as follows).
● Every data point, figure coefficients of presence in their gatherings.
The point x coefficients degree of presence in the kth pack is wk(x). The centroid gathering is the mean equivalent is weighted by the degree of having a spot with the pack, or, numerically, addressed in (4)
here m is termed as hyper-boundary. The calculation endeavours to segment a limited assortment of n components
The FCM minimized objective function from (5).
Feature Extracted
Contrast, Mean, Entropy, Elasticity, Correlation, Homogeneity, Circularity, Orientation, Energy, Solidity, Euler number, Standard deviation, Area, Roundness, Major axis length are taken as a Morphological features and Statistics in image processing. Loop and LGP are taken as Textual Features in this paper.
3.4 Chicken -Sine Cosine Algorithm
It is exceptionally basic from the scientific and algorithmic points of view. It gives in numerous cases exceptionally exact outcomes. This calculation probably won’t have the option to beat after calculations on explicit arrangement of issues. Presences of four arbitrary boundaries are accessible. The tent guide is applied to a nearby interest depending on the best person of the chicken enormous number and the subjectively picked chicken is replaced by the picked person. Scattered chicken huge number smoothing out is proposed finally. The key centers are delineated as follows.
Adaptation Search and Probability: This interest is progressively convincing in a little space; anyway it requires a long exertion to glance in an enormous space, which impacts the capability of the estimation. In this paper, the progression of the estimate changes the search space is adaptively adjusted by the progression of the estimation.
where
Chicken Algorithm:
Stage 1: Determine the boundaries size N, no. of cycles M, singular measurements d, refreshed recurrence G, the no of chickens, hens, chicks and mother hens P1, P2, P3 and P4 following coefficients FL, Cmax is most extreme disorderly inquiry α is a search factor.
Stage 2: Initialize the chick esteem that is arbitrarily produced between the upper and lower limits and do emphasis.
Stage 3: Update swarm esteems
Stage 4: Select best fit worth
Stage 5: Randomly select a chicken esteem and supplant with best fit worth.
Stage 6: If the most extreme no of emphases arrived at then stop, if not bring 3 back.
The Sine Cosine Algorithm (SCA) was proposed by S. Mirjalili as a populace based meta-heuristic calculation in which it utilized the sine and cosine capacities to look for the ideal arrangement. In this manner, the SCA calculations, like other MH calculations, begin by producing a lot of N arrangements called X utilizing the accompanying condition.
A nonlinear programming problem is stated as follows
Global minimum: for the function
Sine Cosine Algorithm:
Stage 1: Initialise the area for search specialists
Stage 2: Evaluate the inquiry specialist by target work
Stage 3: Update the area of the acquired best arrangement
Stage 4: Update the boundaries r1, r2, r3 and r4
Stage 5: Update the situation of search operators
Stage 6: Record the best arrangement
where r1 shows next bit locales, r2 characterizes how for the development ought to be towards or outwards, r3 gives irregular loads for goal so as to stochastically underscore (r3 > 1) or deemphasize (r3 < 1) the impact of desalination in characterizing the separation. At long last the boundary r4 similarly switches between the sine and cosine segments.
From the (6a) to (6f) defines the mathematical form of Chicken Sine Cosine.
3.5 Deep Belief Networks (DBN)
Deep Belief Networks are utilized to perceive, group and create pictures, video successions and movement catch information. A ceaseless profound conviction arranges is just an augmentation of a profound conviction organized that acknowledges a continuum of decimals, instead of paired information. DBN model joint distribution between observed vector x and l hidden layers
The above equation is a conditional distribution for the visible conditioned units on the hidden units of the RBM (Restricted Boltzmann Machines) at each level k and
The resulted outcome of the proposed Chicken-Sine Cosine Algorithm (CSCA) will be greater than the accuracy of 96.5%, sensitivity of 93.2%, and the specificity of 98.1%, which have been obtained by the method Modified Spatial Kernelized Fuzzy C-Means (MSFCM) and ensemble learning. The implementation of the proposed approach done in MATLAB and the dataset that is employed is LIDC-IDRI . The performance of the proposed technique for lung tumorsegmentation, and cancer detection evaluated using three metrics, namely accuracy, sensitivity and specificity, and the results attained compared with that of existing works.
Fig. 6 the result of Nodule Segmentation produced from trained dataset is divided into individual segmented images and classified as nodule and non-nodule images by keeping CT slice thickness M = 5(nodule) and M = 1 (non-nodule).
Figure 6: The result of nodule segmentation
In the Fig. 6, it is shown Nodule and Non-Nodule segmented images. Here the thickness of CT slice is M = 5 and M = 1. The below Tables 3 and 4 gives information on calculations of Sine Cosine algorithm and Deep Belief Network values irrespective of Sensitivity, Specificity and Figs. 7 and 8 represents their graph.
Figure 7: Sensitivity representation graph
Figure 8: Specificity representation graph
The above Table 5 gives the performance comparison of datasets used in this paper. Table 6 gives information on the performance of algorithms.
Nodule depends on the Deep Belief Network (DBN) highlight articulation just as the radiological quantitative picture includes articulation. For foreseeing harmful lung knobs utilizing CT filter pictures, we utilized totally isolated datasets for preparing and for approval. We led a precise report and examination of the models. The Figs. 6–8 are produced when the dataset is arranged according to Tables 2–4 characteristics. The algorithms are compared with the same dataset and results are given in Tables 5 and 6. The profound learning-based knob order results were additionally assessed with various elements, for example, tolerant family ancestry, age, smoking history, clinical biomarkers, size, and area of the distinguished knob. A ton of investigations were performed on the publically. The finding to be greater to achieve the result. The agreement danger rating was the normal of all harm appraisals appointed to all cuts remembered for the last accord division, adjusted to the closest whole number. “Non-knob” areas were sectioned utilizing a mechanized Python programming library. The sectioned areas were additionally prepared by a MATLAB library to create the quantitative picture highlights estimations. The exhibition assessment of lung knob division and malignant growth arrangement dependent on CSCA is registered utilizing three measurements correctly, exactness, affectability, and the particularity. Results show the predominance of the proposed framework with lesser computational expense. Finally we conclude that the combination of Chicken Swarm and Sine Cosine Algorithms produces high accurate results, accuracy 99%, sensitivity 92%, and specificity 91% when compared with other optimization techniques. The same proposed method can also be applicable in finding Breast Cancer, Heart Disease etc.
Funding Statement: The authors received no specific funding for this study.
Conflicts of Interest: The authors declare that they have no conflicts of interest to report regarding the present study.
References
1. A. Soliman, F. Khalifa, A. Elnakib, M. Abou El-Ghar, N. Dunlap et al., “Accurate lungs segmentation on ct chest images by adaptive appearance-guided shape modelling,” IEEE Transactions on Medical Imaging, vol. 36, no. 1, pp. 263–276, 2016 [Google Scholar] [PubMed]
2. F. V. Farahani, A. Ahmadi and M. H. F. Zarandi, “Hybrid intelligent approach for diagnosis of the lung nodule from CT images using spatial kernelized fuzzy c-means and ensemble learning,” Mathematics and Computers in Simulation, vol. 149, pp. 48–68, 2018. [Google Scholar]
3. G. Wei, H. Ma, W. Qian, F. Han, H. Jiang et al., “Lung nodule classification using local kernel regression models with out-of-sample extension,” Biomedical Signal Processing and Control, vol. 40, pp. 1–9, 2018. [Google Scholar]
4. S. Qiu, D. Wen, Y. Cui and J. Feng, “Lung nodules detection in ct images using gestalt-based algorithm,” Chinese Journal of Electronics, vol. 25, no. 4, pp. 711–718, 2016. [Google Scholar]
5. S. Wang, M. Zhou, Z. Liu, Z. Liu, D. Gu et al., “Central focused convolutional neural networks. developing a data-driven model for lung nodule segmentation,” Medical Image Analysis, vol. 40, pp. 172–183, 2017 [Google Scholar] [PubMed]
6. S. Mukhopadhyay, “A segmentation framework of pulmonary nodules in lung CT images,” Journal of Medical Imaging, vol. 29, no. 1, pp. 86–103, 2016. [Google Scholar]
7. Z. Wang, J. Xin, P. Sun, Z. Lin, Y. Yao et al., “Improved lung nodule diagnosis accuracy using lung CT images with uncertain class,” Computer Methods and Programs in Biomedicine, vol. 162, pp. 197–209, 2018 [Google Scholar] [PubMed]
8. A. Bhattacharjee and S. Majumder, “Automated computer-aided lung cancer detection system,” in Advances in Communication, Devices and Networking, Springer, Singapore, pp. 425–433, 2019. [Google Scholar]
9. S. Poonkuzhali and N. Deepika, “Design of hybrid classifier for prediction of diabetes through feature relevance analysis,” International Journal of Innovative Science, Engineering & Technology, vol. 2, pp. 788–793, 2015. [Google Scholar]
10. A. G. Binsaadoon and E. S. M. El-Alfy, “Gait-based recognition for human identification using fuzzy local binary patterns,” International Conference on Agents and Artificial Intelligence, vol. 2, pp. 314–321, 2016. [Google Scholar]
11. T. Chakraborti, B. McCane, S. Mills and U. Pal, “LOOP descriptor. encoding repeated local patterns for fine-grained visual identification of lepidoptera,” IEEE Signal Processing Letters, vol. 25, pp. 335–639, 2018. [Google Scholar]
12. B. Wang, W. Li, X. Chen and H. Chen, “Improved chicken swarm algorithms based on chaos theory and Its application in wind power interval prediction,” Mathematical Problems in Engineering, vol. 12, pp. 1476–1492, 2019. [Google Scholar]
13. S. Mirjalili, “SCA: A sine cosine algorithm for solving optimization problems,” Knowledge-Based Systems, vol. 96, no. 1, pp. 120–133, 2016. [Google Scholar]
Cite This Article
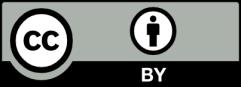