Open Access
ARTICLE
A Method of Multimodal Emotion Recognition in Video Learning Based on Knowledge Enhancement
1 School of Information Science and Engineering, Guilin University of Technology, Guilin, 541004, China
2 Guangxi Key Laboratory of Embedded Technology and Intelligent System, Guilin University of Technology, Guilin, 541004, China
3 Guangxi Key Lab of Multi-Source Information Mining and Security, Guangxi Normal University, Guilin, 541004, China
* Corresponding Author: Xiaomei Tao. Email:
Computer Systems Science and Engineering 2023, 47(2), 1709-1732. https://doi.org/10.32604/csse.2023.039186
Received 13 January 2023; Accepted 20 March 2023; Issue published 28 July 2023
Abstract
With the popularity of online learning and due to the significant influence of emotion on the learning effect, more and more researches focus on emotion recognition in online learning. Most of the current research uses the comments of the learning platform or the learner’s expression for emotion recognition. The research data on other modalities are scarce. Most of the studies also ignore the impact of instructional videos on learners and the guidance of knowledge on data. Because of the need for other modal research data, we construct a synchronous multimodal data set for analyzing learners’ emotional states in online learning scenarios. The data set recorded the eye movement data and photoplethysmography (PPG) signals of 68 subjects and the instructional video they watched. For the problem of ignoring the instructional videos on learners and ignoring the knowledge, a multimodal emotion recognition method in video learning based on knowledge enhancement is proposed. This method uses the knowledge-based features extracted from instructional videos, such as brightness, hue, saturation, the videos’ click-through rate, and emotion generation time, to guide the emotion recognition process of physiological signals. This method uses Convolutional Neural Networks (CNN) and Long Short-Term Memory (LSTM) networks to extract deeper emotional representation and spatiotemporal information from shallow features. The model uses multi-head attention (MHA) mechanism to obtain critical information in the extracted deep features. Then, Temporal Convolutional Network (TCN) is used to learn the information in the deep features and knowledge-based features. Knowledge-based features are used to supplement and enhance the deep features of physiological signals. Finally, the fully connected layer is used for emotion recognition, and the recognition accuracy reaches 97.51%. Compared with two recent researches, the accuracy improved by 8.57% and 2.11%, respectively. On the four public data sets, our proposed method also achieves better results compared with the two recent researches. The experiment results show that the proposed multimodal emotion recognition method based on knowledge enhancement has good performance and robustness.Keywords
Cite This Article
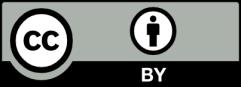