Open Access
ARTICLE
Heart Disease Prediction Using Convolutional Neural Network with Elephant Herding Optimization
School of Information Technology and Engineering, Vellore Institute of Technology, Vellore, 632014, India
* Corresponding Author: R. Subhashini. Email:
Computer Systems Science and Engineering 2024, 48(1), 57-75. https://doi.org/10.32604/csse.2023.042294
Received 25 May 2023; Accepted 24 July 2023; Issue published 26 January 2024
Abstract
Heart disease is a major cause of death for many people in the world. Each year the death rate of people affected with heart disease increased a lot. Machine learning models have been widely used for the prediction of heart disease from the different University of California Irvine (UCI) Machine Learning Repositories. But, due to certain data, it predicts less accurately, whereas, for large data, its sub-model deep learning is used. Our literature work has identified that only traditional methods are used for the prediction of heart disease. It will produce less accuracy. To produce more efficacy, Euclidean Distance was used in this work for data pre-processing that will clean the unwanted data and metaheuristics bio-inspired algorithm such as elephant herding optimization (EHO) is utilized for feature selection. Then, this article proposes deep learning models such as convolutional neural network (CNN) and Inception-ResNet-v2 model for the prediction of heart disease from the benchmark dataset such as the UCI Cleveland heart dataset. Finally, the proposed hybrid model utilizes a convolutional neural network with an Inception-ResNet-v2 in the third layer of the architecture that classifies heart disease with the promising result of 98.77%, accuracy for the Cleveland dataset which outperforms all the other state-of-the-art methods. In future work, this model can be used to predict other diseases such as cancer, brain tumor and COVID-19 in available datasets for the betterment of human lives.Keywords
Cite This Article
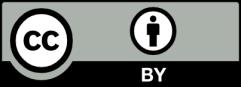