Open Access
ARTICLE
Micro-Locational Fine Dust Prediction Utilizing Machine Learning and Deep Learning Models
1 Department of Applied Artificial Intelligence, Sungkyunkwan University, Seoul, 03063, Korea
2 Department of Human-Artificial Intelligence Interaction, Sungkyunkwan University, Seoul, 03063, Korea
3 AI Team, Raon Data, Seoul, 03073, Korea
* Corresponding Author: Eunil Park. Email:
# These two authors contributed equally to this work
Computer Systems Science and Engineering 2024, 48(2), 413-429. https://doi.org/10.32604/csse.2023.041575
Received 28 April 2023; Accepted 11 July 2023; Issue published 19 March 2024
A correction of this article was approved in:
Correction: Micro-Locational Fine Dust Prediction Utilizing Machine Learning and Deep Learning Models
Read correction
Abstract
Given the increasing number of countries reporting degraded air quality, effective air quality monitoring has become a critical issue in today’s world. However, the current air quality observatory systems are often prohibitively expensive, resulting in a lack of observatories in many regions within a country. Consequently, a significant problem arises where not every region receives the same level of air quality information. This disparity occurs because some locations have to rely on information from observatories located far away from their regions, even if they may be the closest available options. To address this challenge, a novel approach that leverages machine learning and deep learning techniques to forecast fine dust concentrations was proposed. Specifically, continuous location features in the form of latitude and longitude values were incorporated into our models. By utilizing a comprehensive dataset comprising weather conditions, air quality measurements, and location properties, various machine learning models, including Random Forest Regression, XGBoost Regression, AdaBoost Regression, and a deep learning model known as Long Short-Term Memory (LSTM) were trained. Our experimental results demonstrated that the LSTM model outperforms the other models, achieving the best score with a root mean squared error of 23.48 in predicting fine dust (PM10) concentrations on an hourly basis. Furthermore, the fact that incorporating location properties, such as longitude and latitude values, enhances the overall quality of the regression models was discovered. Additionally, the implications and contributions of our research were discussed. By implementing our approach, the cost associated with relying solely on existing observatories can be substantially reduced. This reduction in costs can pave the way for economically efficient fine dust observation systems, ensuring more widespread and accurate air quality monitoring across different regions.Keywords
Cite This Article
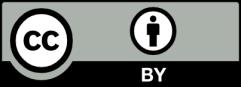
This work is licensed under a Creative Commons Attribution 4.0 International License , which permits unrestricted use, distribution, and reproduction in any medium, provided the original work is properly cited.