Open Access
ARTICLE
Short-Term Prediction of Photovoltaic Power Based on Fusion Device Feature-Transfer
1 College of Electrical Engineering, Liaoning University of Technology, Jinzhou, 121001, China
2 School of Electrical Engineering, Shandong University, Jinan, 250012, China
* Corresponding Author: Zhongyao Du. Email:
Energy Engineering 2022, 119(4), 1419-1438. https://doi.org/10.32604/ee.2022.020283
Received 15 November 2021; Accepted 18 February 2022; Issue published 23 May 2022
Abstract
To attain the goal of carbon peaking and carbon neutralization, the inevitable choice is the open sharing of power data and connection to the grid of high-permeability renewable energy. However, this approach is hindered by the lack of training data for predicting new grid-connected PV power stations. To overcome this problem, this work uses open and shared power data as input for a short-term PV-power-prediction model based on feature transfer learning to facilitate the generalization of the PV-power-prediction model to multiple PV-power stations. The proposed model integrates a structure model, heat-dissipation conditions, and the loss coefficients of PV modules. Clear-Sky entropy, characterizes seasonal and weather data features, describes the main meteorological characteristics at the PV power station. Taking gate recurrent unit neural networks as the framework, the open and shared PV-power data as the source-domain training label, and a small quantity of power data from a new grid-connected PV power station as the target-domain training label, the neural network hidden layer is shared between the target domain and the source domain. The fully connected layer is established in the target domain, and the regularization constraint is introduced to fine-tune and suppress the overfitting in feature transfer. The prediction of PV power is completed by using the actual power data of PV power stations. The average measures of the normalized root mean square error (NRMSE), the normalized mean absolute percentage error (NMAPE), and the normalized maximum absolute percentage error (NLAE) for the model decrease by 15%, 12%, and 35%, respectively, which reflects a much greater adaptability than is possible with other methods. These results show that the proposed method is highly generalizable to different types of PV devices and operating environments that offer insufficient training data.Keywords
Cite This Article
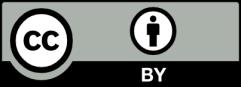