Open Access
ARTICLE
Anomaly Detection and Pattern Differentiation in Monitoring Data from Power Transformers
1 State Grid Hebei Electric Power Research Institute, Shijiazhuang, 050021, China
2 Hebei Provincial Key Laboratory of Power Transmission Equipment Security Defense, North China Electric Power University, Baoding, 071003, China
3 State Key Laboratory of Alternate Electrical Power System with Renewable Energy Sources, North China Electric Power University, Beijing, 102206, China
* Corresponding Author: Quan Wang. Email:
Energy Engineering 2022, 119(5), 1811-1828. https://doi.org/10.32604/ee.2022.020490
Received 26 November 2021; Accepted 15 February 2022; Issue published 21 July 2022
Abstract
Aiming at the problem of abnormal data generated by a power transformer on-line monitoring system due to the influences of transformer operation state change, external environmental interference, communication interruption, and other factors, a method of anomaly recognition and differentiation for monitoring data was proposed. Firstly, the empirical wavelet transform (EWT) and the autoregressive integrated moving average (ARIMA) model were used for time series modelling of monitoring data to obtain the residual sequence reflecting the anomaly monitoring data value, and then the isolation forest algorithm was used to identify the abnormal information, and the monitoring sequence was segmented according to the recognition results. Secondly, the segmented sequence was symbolised by the improved multi-dimensional SAX vector representation method, and the assessment of the anomaly pattern was made by calculating the similarity score of the adjacent symbol vectors, and the monitoring sequence correlation was further used to verify the assessment. Finally, the case study result shows that the proposed method can reliably recognise abnormal data and accurately distinguish between invalid and valid anomaly patterns.Keywords
Cite This Article
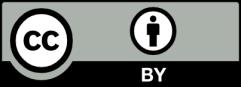