Open Access
ARTICLE
Threshold Parameters Selection for Empirical Mode Decomposition-Based EMG Signal Denoising
1 Department of Biomedical Engineering and Sciences, School of Mechanical and Manufacturing Engineering, National University of Sciences and Technology (NUST), Islamabad, 44000, Pakistan
2 Department of Computer Science and Information, College of Science in Zulfi, Majmaah University, Al-Majmaah, 11952, Saudi Arabia
* Corresponding Author: Asim Waris. Email:
(This article belongs to the Special Issue: Computational Intelligence for Internet of Medical Things and Big Data Analytics)
Intelligent Automation & Soft Computing 2021, 27(3), 799-815. https://doi.org/10.32604/iasc.2021.014765
Received 14 October 2020; Accepted 03 January 2021; Issue published 01 March 2021
Abstract
Empirical Mode Decomposition (EMD) is a data-driven and fully adaptive signal decomposition technique to decompose a signal into its Intrinsic Mode Functions (IMF). EMD has attained great attention due to its capabilities to process a signal in the frequency-time domain without altering the signal into the frequency domain. EMD-based signal denoising techniques have shown great potential to denoise nonlinear and nonstationary signals without compromising the signal’s characteristics. The denoising procedure comprises three steps, i.e., signal decomposition, IMF thresholding, and signal reconstruction. Thresholding is performed to assess which IMFs contain noise. In this study, Interval Thresholding (IT), Iterative Interval Thresholding (IIT), and Clear Iterative Interval Thresholding (CIIT) techniques have been explored for denoising of electromyography (EMG) signals. The effect of different thresholding operators, i.e., SOFT, HARD, and Smoothly Clipped Absolute Deviation (SCAD), on the performance of EMD-based EMG denoising techniques is also investigated. 15 EMG signals, recorded from the upper limb of 5 healthy subjects, were used to identify the best possible combination of thresholding technique and thresholding operator for denoising EMG signals. The performance of denoising techniques is assessed by calculating the Signal to Noise (SNR) ratio of the signals. The results are further evaluated using a two-way Analysis of Variance (ANOVA) statistical test. The results demonstrated that the mean SNR values yielded by the IIT thresholding technique outperform the IT thresholding technique (P-value < 0.05), but there is no significant difference in mean SNR values of IIT and CIIT techniques (P-value = 0.9951). For thresholding operators, there is no significant difference in mean SNR values of the HARD and SOFT operator (P-value = 0.0968), whereas the HARD operator outperforms SCAD (P-value < 0.05). It has also shown that the combination of IIT thresholding with SOFT operator and threshold value equal to half of the universal threshold denoise the EMG signals while preserving the original signal’s characteristics. IIT-based EMD denoising technique with HARD thresholding operator yields the highest SNR, irrespective of the level of noise embedded in the signal. Whereas IIT with SOFT operator provides comparable SNR and successfully preserves the shape of EMG signals. The identified combination of thresholding technique and thresholding operator can eliminate various noises embedded in EMG signals.Keywords
Cite This Article
Citations
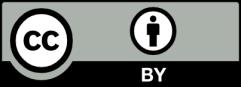