Open Access
ARTICLE
Novel Power Transformer Fault Diagnosis Using Optimized Machine Learning Methods
1 Electrical Engineering Department, College of Engineering, Taif University, Taif, 21944, Saudi Arabia
2 Department of Electrical Power and Machines Engineering, Faculty of Engineering, Tanta University, Tanta, 31511, Egypt
* Corresponding Author: Diaa-Eldin A. Mansour. Email:
Intelligent Automation & Soft Computing 2021, 28(3), 739-752. https://doi.org/10.32604/iasc.2021.017703
Received 05 February 2021; Accepted 08 March 2021; Issue published 20 April 2021
Abstract
Power transformer is one of the more important components of electrical power systems. The early detection of transformer faults increases the power system reliability. Dissolved gas analysis (DGA) is one of the most favorite approaches used for power transformer fault prediction due to its easiness and applicability for online diagnosis. However, the imbalanced, insufficient and overlap of DGA dataset impose a challenge towards powerful and accurate diagnosis. In this work, a novel fault diagnosis for power transformers is introduced based on DGA by using data transformation and six optimized machine learning (OML) methods. Four data transformation techniques are used with the dissolved gasses of transformer oils to reduce the high overlap of dataset samples. The OML methods used for transformer fault diagnosis are decision tree, discriminant analysis, Naïve Bayes, support vector machines, K-nearest neighboring, and ensemble classification methods. The six OML methods are implemented by MATLAB/Software based on 542 dataset samples collected from laboratories and literature. In this regard, 361 dataset samples were used for training, while 181 dataset samples were used for testing. The transformer fault diagnosis based on the OML methods had superior predicting accuracy compared to conventional and artificial intelligence methods.Keywords
Cite This Article
Citations
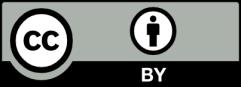