Open Access
ARTICLE
A Multi-Task Network for Cardiac Magnetic Resonance Image Segmentation and Classification
1 Chengdu Institute of Computer Application, University of Chinese Academy of Sciences, Chengdu, 610041, China
2 School of Computer Science, Chengdu University of Information Technology, Chengdu, 610225, China
3 BMSc, Department of Cardiology, West China Hospital, Sichuan University, Chengdu, 610041, China
4 University of Chinese Academy of Sciences, Beijing, 100049, China
5 Department of Experimental Rheumatology, Nijmegen, 6525GA, Netherlands
* Corresponding Author: Zhe Cui. Email:
Intelligent Automation & Soft Computing 2021, 30(1), 259-272. https://doi.org/10.32604/iasc.2021.016749
Received 10 January 2021; Accepted 25 February 2021; Issue published 26 July 2021
Abstract
Cardiomyopathy is a group of diseases that affect the heart and can cause serious health problems. Segmentation and classification are important for automating the clinical diagnosis and treatment planning for cardiomyopathy. However, this automation is difficult because of the poor quality of cardiac magnetic resonance (CMR) imaging data and varying dimensions caused by movement of the ventricle. To address these problems, a deep multi-task framework based on a convolutional neural network (CNN) is proposed to segment the left ventricle (LV) myocardium and classify cardiopathy simultaneously. The proposed model consists of a longitudinal encoder–decoder structure that obtains high- and low-level features at the same time. The encoder employs a feature pyramid module (FPM) and dense atrous convolution (DAC) to extract features from images with variable scales for classification. Meanwhile, the decoder leverages the subpixel layer to recover spatial information caused by downsampling in the encoder for segmentation. The approach was verified using 654 magnetic resonance images. It achieved a Dice similarity coefficient (DSC) metric of 82.14% on segmentation and a classification accuracy of 95.72%, with an area under the receiver operating characteristic curve (AUC) of 97.88%. The proposed method can aid in the segmentation of cardiac magnetic images and improve the classification accuracy of cardiopathy.Keywords
Cite This Article
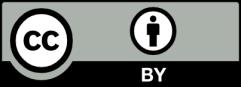