Open Access
ARTICLE
Machine Learning Based Diagnosis for Diabetic Retinopathy for SKPD-PSC
1 Department of Computer Science and Engineering, Erode Sengunthar Engineering College, Perundurai, Erode, 638057, Tamil Nadu, India
2 Department of Information Systems, College of Computer Sciences and Information Technology, King Faisal University, Al Hasa, Saudi Arabia
3 Department of Information Systems, College of Computer and Information Science, Princess Nourah bint Abdulrahman University, P.O. BOX 84428, Riyadh, 11671, Saudi Arabia
4 Department of Computer Science & Engineering, Swami Keshvanand Institute of Technology, Management & Gramothan (SKIT), Jaipur, 302017, Rajasthan, India
* Corresponding Author: M. P. Thiruvenkatasuresh. Email:
Intelligent Automation & Soft Computing 2023, 36(2), 1767-1782. https://doi.org/10.32604/iasc.2023.033711
Received 25 June 2022; Accepted 19 August 2022; Issue published 05 January 2023
Abstract
The study aimed to apply to Machine Learning (ML) researchers working in image processing and biomedical analysis who play an extensive role in comprehending and performing on complex medical data, eventually improving patient care. Developing a novel ML algorithm specific to Diabetic Retinopathy (DR) is a challenge and need of the hour. Biomedical images include several challenges, including relevant feature selection, class variations, and robust classification. Although the current research in DR has yielded favourable results, several research issues need to be explored. There is a requirement to look at novel pre-processing methods to discard irrelevant features, balance the obtained relevant features, and obtain a robust classification. This is performed using the Steerable Kernalized Partial Derivative and Platt Scale Classifier (SKPD-PSC) method. The novelty of this method relies on the appropriate non-linear classification of exclusive image processing models in harmony with the Platt Scale Classifier (PSC) to improve the accuracy of DR detection. First, a Steerable Filter Kernel Pre-processing (SFKP) model is applied to the Retinal Images (RI) to remove irrelevant and redundant features and extract more meaningful pathological features through Directional Derivatives of Gaussians (DDG). Next, the Partial Derivative Image Localization (PDIL) model is applied to the extracted features to localize candidate features and suppress the background noise. Finally, a Platt Scale Classifier (PSC) is applied to the localized features for robust classification. For the experiments, we used the publicly available DR detection database provided by Standard Diabetic Retinopathy (SDR), called DIARETDB0. A database of 130 image samples has been collected to train and test the ML-based classifiers. Experimental results show that the proposed method that combines the image processing and ML models can attain good detection performance with a high DR detection accuracy rate with minimum time and complexity compared to the state-of-the-art methods. The accuracy and speed of DR detection for numerous types of images will be tested through experimental evaluation. Compared to state-of-the-art methods, the method increases DR detection accuracy by 24% and DR detection time by 37.Keywords
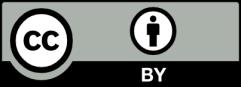