Open Access
ARTICLE
Power Transformer Fault Diagnosis Using Random Forest and Optimized Kernel Extreme Learning Machine
1 School of Electrical Engineering, Xinjiang University, Urumqi, 830047, China
2 School of Mathematics, Xinjiang University, Urumqi, 830047, China
3 Sichuan Energy Internet Research Institute Under Tsinghua University, Cheng Du, 610213, China
* Corresponding Author: Xiaojing Ma. Email:
Intelligent Automation & Soft Computing 2023, 37(1), 691-705. https://doi.org/10.32604/iasc.2023.037617
Received 11 November 2022; Accepted 06 February 2023; Issue published 29 April 2023
Abstract
Power transformer is one of the most crucial devices in power grid. It is significant to determine incipient faults of power transformers fast and accurately. Input features play critical roles in fault diagnosis accuracy. In order to further improve the fault diagnosis performance of power transformers, a random forest feature selection method coupled with optimized kernel extreme learning machine is presented in this study. Firstly, the random forest feature selection approach is adopted to rank 42 related input features derived from gas concentration, gas ratio and energy-weighted dissolved gas analysis. Afterwards, a kernel extreme learning machine tuned by the Aquila optimization algorithm is implemented to adjust crucial parameters and select the optimal feature subsets. The diagnosis accuracy is used to assess the fault diagnosis capability of concerned feature subsets. Finally, the optimal feature subsets are applied to establish fault diagnosis model. According to the experimental results based on two public datasets and comparison with 5 conventional approaches, it can be seen that the average accuracy of the proposed method is up to 94.5%, which is superior to that of other conventional approaches. Fault diagnosis performances verify that the optimum feature subset obtained by the presented method can dramatically improve power transformers fault diagnosis accuracy.Keywords
Cite This Article
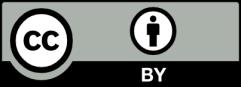