Open Access
ARTICLE
Melanoma Detection Based on Hybridization of Extended Feature Space
DCSA, Panjab University, Chandigarh, 160014, India
* Corresponding Author: Shakti Kumar. Email:
Intelligent Automation & Soft Computing 2023, 37(2), 2175-2198. https://doi.org/10.32604/iasc.2023.039093
Received 10 January 2023; Accepted 11 April 2023; Issue published 21 June 2023
Abstract
Melanoma is a perfidious form of skin cancer. The study offers a hybrid framework for the automatic classification of melanoma. An Automatic Melanoma Detection System (AMDS) is used for identifying melanoma from the infected area of the skin image using image processing techniques. A larger number of pre-existing automatic melanoma detection systems are either commercial or their accuracy can be further improved. The research problem is to identify the best preprocessing technique, feature extractor, and classifier for melanoma detection using publically available MED-NODE data set. AMDS goes through four stages. The preprocessing stage is for noise removal; the segmentation stage is for extracting lesions from infected skin images; the feature extraction stage is for determining the features like asymmetry, border, and color, and the classification stage is to classify the lesion as benign or melanoma. The infected input image for the AMDS may contain impurities such as noise, illumination, artifacts, and hairs. In the proposed methodology an algorithm LePrePro is proposed for the preprocessing stage for denoising and brightness cum contrast normalization and another algorithm LeFET is proposed for extending the feature vector space in the feature extraction stage using a hybrid approach. In the study, a novel approach has been proposed in which different classifiers, feature extractions, and data preprocessing steps of the AMDS are compared. In a conclusion, this comparison revealed that on experimentation using Med-Node and ISIC 2017 Dataset, the best results included Gaussian blur as the best data preprocessing step, Extended feature vector which is the combination of Hue Saturation Value (HSV), and Local Binary Pattern (LBP) was the best feature extraction method, and the ensemble bagged tree was the best classification technique on the Med-Node data sets with 99% Area Under the Receiver Operating Characteristic Curve (AUC), 93.52% accuracy, 90.82% sensitivity, and 98.36% specificity in the proposed automatic melanoma detection system.Keywords
Cite This Article
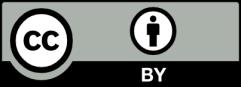