Open Access
ARTICLE
Pure Detail Feature Extraction Network for Visible-Infrared Re-Identification
1 Zhejiang University of Technology, Hangzhou, 310023, China
2 Hubei Key Laboratory of Intelligent Vision Based Monitoring for Hydroelectric Engineering, The College of Computer and Information, China Three Gorges University, Yichang, 443002, China
3 The College of Electrical and Information Engineering, Quzhou University, Quzhou, 324000, China
* Corresponding Author: Sixian Chan. Email:
(This article belongs to the Special Issue: Computer Vision and Machine Learning for Real-Time Applications)
Intelligent Automation & Soft Computing 2023, 37(2), 2263-2277. https://doi.org/10.32604/iasc.2023.039894
Received 22 February 2023; Accepted 15 May 2023; Issue published 21 June 2023
Abstract
Cross-modality pedestrian re-identification has important applications in the field of surveillance. Due to variations in posture, camera perspective, and camera modality, some salient pedestrian features are difficult to provide effective retrieval cues. Therefore, it becomes a challenge to design an effective strategy to extract more discriminative pedestrian detail. Although many effective methods for detailed feature extraction are proposed, there are still some shortcomings in filtering background and modality noise. To further purify the features, a pure detail feature extraction network (PDFENet) is proposed for VI-ReID. PDFENet includes three modules, adaptive detail mask generation module (ADMG), inter-detail interaction module (IDI) and cross-modality cross-entropy (CMCE). ADMG and IDI use human joints and their semantic associations to suppress background noise in features. CMCE guides the model to ignore modality noise by generating modality-shared feature labels. Specifically, ADMG generates masks for pedestrian details based on pose estimation. Masks are used to suppress background information and enhance pedestrian detail information. Besides, IDI mines the semantic relations among details to further refine the features. Finally, CMCE cross-combines classifiers and features to generate modality-shared feature labels to guide model training. Extensive ablation experiments as well as visualization results have demonstrated the effectiveness of PDFENet in eliminating background and modality noise. In addition, comparison experiments in two publicly available datasets also show the competitiveness of our approach.Keywords
Cite This Article
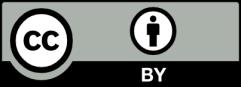