Open Access
ARTICLE
Integrated Transcriptome and Small RNA Sequencing Analyses Reveals Insights into the Molecular Mechanism of Seed Germination in Mung Bean
1 Institute of Crop Germplasm Resources, Shandong Academy of Agricultural Sciences, Jinan, 250100, China
2 State Key Laboratory of Crop Biology, Shandong Agricultural University, Tai’an, 271018, China
3 College of Life Science, Shandong Normal University, Jinan, 250014, China
4 Weifang Academy of Agricultural Sciences, Weifang, 261000, China
* Corresponding Authors: Hanfeng Ding. Email: ; Nana Li. Email:
(This article belongs to the Special Issue: Integrating Agronomy and Plant Physiology for Improving Crop Production)
Phyton-International Journal of Experimental Botany 2023, 92(6), 1793-1812. https://doi.org/10.32604/phyton.2023.026822
Received 27 September 2022; Accepted 03 January 2023; Issue published 11 April 2023
Abstract
During the life cycle of a plant, seed germination is crucial. Upon ingestion of water, the dry seeds resumed energy metabolism and cellular repair. To dissect the complex mechanisms at the very beginning of seed germination, two approaches including transcriptome and small RNA sequencing were conducted during the water imbibition process of mung bean seeds compared with dry seed. The transcriptome sequencing analysis identified 10,108 differentially expressed genes (DEGs) between dry and imbibed mung bean seeds. KEGG enrichment analyses demonstrated numerous DEGs involved in hormone signaling pathways, carbohydrate, and energy metabolism. Out of the total DEGs, 129 genes were investigated to involve in abscisic acid, gibberellin, and ethylene pathways, with 56 genes involved in glycolysis and TCA cycle. Through the analysis of small RNA sequencing among different samples, there were 284 miRNAs found in the imbibed and dried seeds of mung bean containing 213 known and 71 novel miRNAs. From comparison between the two types of seeds, 51 miRNAs were differentially expressed. The integrated analyses of transcriptome and miRNAome data showed that 23 DEGs are the putative target genes of 8 miRNAs including miR156, miR171b-3p, miR166e-3p, miR169-1, etc. QRT-PCR was used to validate several DEGs and miRNAs. The data suggested that the seed imbibition process is regulated by a variety of DEGs and microRNAs. Meanwhile, we hope to gain a deeper insight into how mung bean seeds germinate in order to optimize seed growth.Keywords
Supplementary Material
Supplementary Material FileA significant edible legume crop, mung beans (Vigna radiataL.) are grown on more than 6 million ha worldwide and are consumed by most households in Asia [1,2]. Mung bean genome sequencing provided excellent opportunities to study mung bean transcriptomes during a variety of developmental stages. A transcriptomic and metabolomic analysis of soybean seeds during germination has also been performed, exploring how expression regulation and nutritional factors are regulated during seed germination [3,4]. However, the global transcriptome and small RNA approaches of mung bean seedlings at early stages of seed germination remain poorly understood. This might limit our understanding of molecular mechanisms during mung bean germination.
Germination involves a variety of metabolic and physiological changes, which begin with the imbibition of fertilizer and culminate with embryonic elongation [5]. In seed germination, macromolecular substances, such as polysaccharides, proteins, and lipids, are degraded into small active compounds and energy is produced. This process is regulated by phytohormones, such as abscisic acid (ABA), gibberellin (GA), ethylene, and others [6,7]. Once seeds imbibed water, germination would begin. When dry seeds ingested water, energy metabolism and cellular repair resumed. The plant hormones ABA and GA play a crucial role in seed development, maturation, dormancy, and germination [8]. When dry seeds are imbibed during the early phase of germination (between 6 and 12 h), their endogenous ABA content rapidly declines [9]. A number of ABA signal transduction proteins play a role in seed germination [10,11]. ABA and GA balance determine seed dormancy status. Meanwhile, miRNAs may regulate seed development, dormancy, and germination [12,13]. For example, SPL13 (SQUAMOSA PROMOTER BINDING PROTEIN-LIKE 13) is the target gene of miR156, and the down-regulation of SPL13 by miR156 appears to be essential for the transition to the vegetative-leaf stages. A mutant SPL13 shows resistance to miR156, and this leads to a delay in vegetative leaf development from cotyledon-stage seedlings [14–16].
Early in the development of plants, dry seeds absorb water and reactivate their physiology [17]. Reactivation events, including those encoding enzymes involved in starch degradation, protein synthesis, and DNA/RNA synthesis, play a key role in seed germination. To get deeper insights into the germination mechanisms, as well as to understand functional interactions between dry and imbibed mung bean seeds, we characterized the expression level of transcriptomes and small RNAs. The analysis of these different datasets identified differentially expressed genes (DEGs), miRNAs, and their targets and how these components interact in the imbibition process, providing a systematic view of mung bean germination mechanisms.
2.1 Plant Materials and RNA Extraction
Dry seeds of Mung bean cultivar Weidou8 were soaked in distilled water, wrapped in paper towels and incubated at 25°C for 10 h in dark. Both imbibed seeds and dry seeds were stored at −80°C. Total RNA of 6 samples was extracted using Ethanol precipitation protocol and CTAB-PBIOZOL reagent according to the manual instructions. Agilent 2100 Bioanalyzer (Agilent Technologies, Santa Clara, CA) and Nanodrop 8000 Spectrophotometer (Thermo Fisher Scientific, Waltham, MA) were used to analyze these total RNA samples on a qualitative and quantitative basis. RNA from each sample was used to construct libraries for transcriptome, small RNA, and quantitative real-time PCR (qRT-PCR). BGI-Wuhan, China, constructed and sequenced the library.
2.2 Transcriptome Sequencing and Data Analysis
We constructed libraries from 2 samples, each with 3 duplicates. In the first step, for the purification of poly-A-containing mRNA molecules, oligo (dT) magnetic beads were used. After purification, mRNA was fragmented under elevated temperatures using fragment buffer. A random hexamer primer was used for reverse transcription, followed by the synthesis of cDNA from the second strand. In the next step, the 5′ tails of the double stranded DNA fragments were then phosphorylated, and the 3′ tails were adenine-added. The fragments were then ligated to sequencing adaptors. To construct cDNA libraries, the fragments were amplified by PCR. Lastly, single end 50 base pair reads were generated using the BGI-SEQ-500 platform (BGI-Wuhan, China).
Each cDNA library generated raw reads. In order to obtain clean reads, the sequencing data was filtered with SOAPnuke [18]. During sequencing, raw reads were subjected to quality control steps. Removed reads include: reads with sequencing adapter, low-quality bases (base quality ≤ 5) over 20%, and reads with unknown bases (‘N’ bases) over 5%. All clean reads were mapped to the reference genome of V. radiata L. (https://www.ncbi.nlm.nih.gov/data-hub/genome/?taxon=157791) using HISAT2 program [19]. Bowtie2 aligned the clean reads to the reference coding genes [20], then gene expression levels were determined using RSEM software [21]. Differentially expression analyses between two samples were performed using DEseq2 [22] which is an R package implemented on the negative binomial distribution. To establish significantly differential expression, a fold change ≥ 2.00 and an adjusted p value ≤ 0.001 were used as thresholds. Gene ontology (GO) and kyoto encyclopedia of genes and genomes (KEGG) enrichment analyses were performed to identify DEGs with enriched GO terms and metabolic pathways. Q value was corrected by Bonferroni by a rigorous threshold (Q value 0.05) in order to assess the significance of terms and pathways [23].
2.3 Small RNA Library Construction, Sequencing, and Data Analysis
Each biological replicate’s RNA was isolated and used to construct small RNA libraries for high-throughput sequencing. Purified 18–30 nucleotide RNA bands were recovered from the total RNA samples after denaturing polyacrylamide gel electrophoresis (PAGE) at 15% (w/v). Then the 3′ ends of the selected small RNA molecules were ligated to 5′-adenylated and 3′-blocked single-stranded DNA adaptors. Next, 3′ adaptors were crossed with reverse transcription primers, and 5′ adaptors were added to the 5′ end of the products. After RT-PCR, cDNA with 100–120 bp length was separated by PAGE gel to eliminate primer-dimers. Finally, sequencing was performed using BGISEQ-500 technology as instructed by the manufacturer [24,25].
Following the removal of adaptor impurities and low-quality reads, a comparison was made between clean small RNA reads and non-coding RNA sequences in the Rfam [26] and GenBank databases [27]. Except for tRNA, rRNA, snRNA, and snoRNA, the rest of the sequences were compared with the reference genome of mung bean using Bowtie2 [28]. To identify conserved miRNAs, they were subjected to a BLASTn search against the miRBase database [29]. An unannotated mung bean genome matched sequence was subjected to RNAfold [30] and miReap to predict precursors and secondary structures in order to identify novel miRNAs. Novel miRNAs have stem-loop structures. By counting absolute numbers of molecules with unique molecular identifiers, small RNA expression levels were calculated [31]. The DEGseq [32] was used to determine differential expression of small RNAs, using a Q value ≤ 0.001 and a Log2Ratio threshold ≥ 1.
2.4 MiRNA Targets Prediction and Regulatory Network Construction
To predict targets for conserved and novel miRNAs, we used psRobot [33] and TargetFinder [34]. A Blast2GO pipeline was used to map predicted target genes of miRNAs with different expression levels to GO terms [35]. To identify pathways involved in differentially expressed miRNA target genes, a KEGG pathway enrichment analysis was performed [36].
2.5 Construction of miRNA-mRNA Regulatory Networks
Using DEMs and DEGs, a regulatory network of miRNA-mRNA interactions was constructed based on positive and negative correlations. The pearson correlation coefficient (PCC) was used to evaluate the correlation between miRNA and mRNA expression. High-confidence pairs with PCC ≤ 0.7 and p < 0.05 were selected as negatively co-expressed miRNA-mRNA pairs.
2.6 Validation of DEGs and miRNAs by qRT-PCR
To validate differential expression of miRNA and mRNA, we randomly selected five miRNAs and ten mRNAs. As described above, total RNA was extracted. In order to synthesize first-strand cDNA for mRNA quantification, SuperScript™I reverse transcriptase (Invitrogen, USA) and Oligo (dT) primers were used. For qRT-PCR, a Bio-Rad CFX96 Real-time System was used. In order to quantify miRNA, with the One Step Primer Script® miRNA cDNA Synthesis Kit (Takara) and SuperScript III Reverse Transcriptase (Invitrogen), single-stranded cDNA was synthesized from miRNA extracted from cells. With the miDETECT TractTM miRNA qRT-PCR Starter Kit (RiboBio, Guangzhou, China), three technical replicates were performed for each reaction to verify miRNA expression. Gene-specific primers and miRNA primers were showed in Table S1. We evaluated the relative expression levels of 5 miRNA and 10 mRNA by 3 biological replications and 3 technical replications using the 2 −ΔΔCt method [37].
3.1 Overview of Transcriptome Sequencing
To illustrate the global patterns of DEGs in seed germination of mung bean, six cDNA libraries were constructed in both dry and imbibed seeds with three duplicates. These libraries sequences by BGISEQ-500 platform generated a total of 129.7 million raw reads with an average of 21.6 million raw reads in each library (Table 1). From each library, 21.25 million clean reads were obtained after removing adaptor sequences, low-quality, N-containing reads, with a clean reads rate of 98.3% (Table 1). A total of 20.6 million and 18.96 million clean reads in each dry seeds (DS) and imbibed seeds (GS) library were mapped to the reference genome, with the average mapping ratio of 97% and 89.25%, respectively (Table 1). To study the correlation between gene expression in different libraries, PCC calculated all gene expression values between two libraries. As shown in Fig. S1, correlation analysis results indicated that the DS and GS treatments have a more than 98.1% of the similarity.
3.2 Differentially Expressed Genes during Seed Germination
A total of 25,675 genes were categorized as expressed genes among all the libraries (Cutoff: reads counts >5). The DEGs were identified with a fold change (|log2 (fold change)| ≥ 1) and adjusted p value ≤ 0.001. Among all expressed genes, 10,108 DEGs were identified between DS and GS groups. Compared to dry seeds, imbibed seeds had 8,448 up-regulated genes and 1,662 down-regulated genes.
In the ABA biosynthesis pathway, two (+)-abscisic acid 8′-hydroxylase (CYP707A) genes (LOC106767139 and LOC106777777) were highly expressed in imbibed seeds. These genes were significantly up-regulated in imbibed seeds (FPKM = 249.2, FPKM = 288.3) compared with dry seeds (FPKM = 2.3, FPKM = 0.29) (Table S2). In the GA signaling pathway, some key genes were detected to involve in the process of seed germination, such as DELLA protein and gibberellin receptor (GID1). DELLA (LOC106766362), and GID1 gene (LOC106768735) along with down regulation in imbibed seeds (FPKM = 3.4, FPKM = 177.4) (Table S2).
In plants, transcription factors (TFs) regulate gene expression during growth and development. In this study, 1,843 TFs distributed into 59 families were expressed in imbibed seeds but not in dry seeds. Among these TFs, MYB (253), AP2-EREBP (182), bHLH (160), WRKY (95), NAC (93) and FAR1 (89) were the most over-expressed TF families (Fig. 1). Differential expression of TFs analysis was performed using adjusted p value ≤ 0.001 and |log2 (fold change)| ≥ 1 as the default threshold to judge the significance of expression difference. We noticed that 744 TFs have significant expression differences and 682 TFs showed higher expression in GS than these in the rest ones. These results showed that the seed imbibing water launched various life activities (Table S3). Based on the comparison of GS and DS, 56 TFs were differentially expressed, most of which belonged to the MYB, AP2-EREBP, bHLH, and WRKY families (Table S3). Meanwhile, these TFs indicated higher expression in the germinate seeds than these in dormant seeds.
Figure 1: Transcription factor (TF) family classification of unigenes. The X axis represents the number of unigenes. The Y axis represents the TF family
3.3 Transcriptome Functional Annotation
Among the 10,108 significantly differential expressed genes, 8,072 expressed genes were annotated by GO with a total of 31,204 GO terms. GO enrichment analysis of DEGs shows all expressed genes which mainly involved in the “cellular component” category was the most enriched, followed by the “molecular function” category (Fig. S2). In molecular function category, “binding” and “catalytic activity” were the most abundant terms (Fig. S2). The biological process category was significantly enriched by “metabolic processes”, “cellular processes” and “biological regulation”. The top 20 terms in DS and GS were shown in Fig. 2A.
Figure 2: A bubble diagram showing the top 20 enriched GO terms (A) and KEGG pathways (B) for DEGs in DS and GS. The X-axis represents the Rich Ratio, which is the ratio between the number of genes annotated to a particular item and the total number of genes in the species. Y axis represents GO term (A) and KEGG pathway (B). A bubble’s size indicates the number of genes associated with a GO term and KEGG pathway. And the color represents Q-value of enrichment. A deeper color has a lower Q-value.
Further, the pathway analysis of DEGs was performed to understand KEGG functional classification using KEGG database. These DEGs were detected to involve in 134 pathways. In all five comparisons, the “Metabolism” pathway was the most enriched pathway based on KEGG functional classification analysis. According to the enrichment analysis under water imbibition, plant hormone signal transduction (ko04075), fatty acid elongation (ko00062), MAPK signaling pathway-plant (ko04016), circadian rhythm-plant (ko04712), glycosaminoglycan degradation (ko00531), and glycosphingolipid biosynthesis-ganglio series (ko00604) were the most over-represented pathways (Fig. 2B). GA, ABA, ethylene, and other plant hormones are necessary for growth and development. Meanwhile, glycolysis (ko00010) and citrate cycle (TCA cycle) (ko00020) also played an important role which can provide an amount of energy in the initial stage of seed germination.
3.4 Small RNA Sequencing and Annotation of Mung Bean miRNAs
Six small RNA libraries were constructed and sequenced for dry and imbibed mung bean seeds (imbibed 10 h). As summarized in Table S2, the small RNA sequences from six libraries were analyzed. After sequencing, 203.38 million raw reads were obtained. After quality control, 189.15 million clean reads with an average of 31.5 million per sample were generated (Table S4). In these libraries, most of the sRNAs were 21–24 nt in length, and the 24-nt sRNAs were particularly abundant (Fig. 3). Clean tags were mapped to several sRNA databases, including miRBase, Rfam, siRNA, piRNA, and snoRNA. Using public databases, the unique sRNAs were aligned to identify conserved/known miRNAs. Lastly, 213 conserved/known miRNAs were identified. According to sequence similarity, miRNAs were clustered into 48 families (Table S5). Among them, miR156 family was the largest family with 30 members, with miR171 family containing 22 members as the second one (Table S5). In addition, the hairpin precursors of 71 novel miRNAs were obtained using the method for novel miRNA prediction (Table S5). In Table S5, the sequences of miRNAs and their precursors are detailed. The average GC content of mature miRNA sequences was 49.4%, with lengths ranging from 18 to 25 nt, the most abundant are 20 and 21 nt (Table S5).
Figure 3: Length distribution and amount of small RNA sequences in dry and imbibed mung bean seed
3.5 Differential Expression Profiles of miRNAs
There were 51 (30 up and 21 down) differentially expressed miRNAs in the imbibed seeds compared with the dry seeds in the present study. There are 34 known miRNAs in 16 families among these miRNAs. Out of them, 17 novel miRNAs differentially expressed between imbibed and dry seeds (Table S6). With 6 members, miR166 was one of the largest miRNA families in mung bean which involved in imbibition (Table S6). MiR156, miR171b-3p, and miR166e-3p showed down-regulated, with miR169-1 indicating up-regulated in the imbibed seeds compared with dry seeds.
3.6 Target Prediction of the miRNAs
The online tools psRobot and TargetFinder were used to predict putative targets of miRNAs in imbibed and dry mung bean seeds for functional analysis. Predicted putative targets totaled 2,128 related to 201 miRNAs, with 1,985 and 143 targets relating to known and novel miRNAs, respectively. From the miRNAs functional analysis in dry and imbibed mung bean seeds, a total of 107 target genes relating to 26 different miRNAs were obtained (Table S7). Among them, some miRNAs and their target genes indicated significantly differential expression and involved in the beginning of the seed germination, such as, miR156, miR171b-3p, miR166e-3p, miR169-1, and their target genes squamosa promoter-binding-like protein (SPL), scarecrow-like (SCL), nuclear transcription factor Y subunit A (NF-YA), and homeobox-leucine zipper protein (HD-ZIP) (Table S7).
3.7 Correlation Analysis of miRNAs Expression Profiles and Their Target Genes
In order to determine whether miRNAs mediate the imbibition process of mung bean seeds, the expression of miRNAs (from small RNA-seq) and their target genes (from RNA-seq) was integrated. The correlation analysis of miRNA and their target mRNA expression profiles was performed using PCC. A total of 23 miRNA-mRNA negative interaction pairs were detected across all combinations under DS and GS (Table 2). Nevertheless, miRNA expression profiles corresponding to these targets were not perfectly negative. Among these, 23 of these miRNAs and targets showed negative correlations. Among them miR156, miR171b-3p, miR166e-3p was down regulated and miR169-1 was up-regulated. Their target genes, such as SPL, SCL, NF-YA, HD-ZIP, showed opposite expression trends with their corresponding miRNAs (Table 2).
3.8 Confirmation of Transcriptome and miRNAs Results by qRT-PCR
To further verify the reliability of high-throughput sequencing results, five differentially expressed miRNAs and 10 mRNAs were selected to test their relative expression level using qRT-PCR. The results which close agreement between the two results were showed in Fig. S3 with R2 = 0.86.
The germination and growth of seeds play a key role in the development of plants. During seed germination, many physiological processes are reprogrammed from a quiet dormant state to an active seedling establishment [38]. As dry seeds imbibed water, energy metabolism and cellular repair occurred with gene regulation involving in the reactivation. When dry seeds absorbed water, seeds began to sprout as energy metabolism and cellular repair were restored. This brought an excellent opportunity for us to understand the imbibing process of plant seeds. In mung bean, there is still a knowledge gap regarding interactions between the global transcriptome and miRNAs at the very beginning of seed germination. Here, we combined the genome-wide transcriptome and small RNA sequencing analysis in both genes and miRNAs in DS and GS genotypes to investigate the molecular mechanism of dry and imbibed mung bean seeds during the early stage of the seeding germination. In this project, A total of 10,108 differential expressed genes, 51 miRNAs and 23 miRNA-mRNA negative interaction staffs were detected to involve in the imbibition process.
4.1 Transcriptional Activation of Primary Metabolism Genes during Germination
Upon imbibition, primary genes in catabolic pathways were induced to sustain germination and seedling growth. The TCA cycle of cellular respiration and glycolysis provide most of the energy needed during seed germination [39]. In dry seeds, ATP levels were low, but increased after germination, indicating that these respiratory pathways provide energy for various cellular functions during seed germination. As a result, seeds need more sugar to convert energy during germination [40]. The products of energy reserves were degraded through glycolysis process, after the synthesis of ATP by the TCA cycle and transportation of ATP by the mitochondrial electron. Here, mung bean germination could increase the quantity of sucrose and monosaccharides which included glucose and fructose, respectively. They could produce ATP through the glycolysis and TCA cycle. In this study, KEGG analysis suggested that a lot of genes involved in glycolytic pathway showed a higher expression in germinated seeds than dormant seeds (Fig. 4A). These gene included phosphoglucomutase (PGM), glucose-6-phosphate isomerase (GPI), 6-phosphofructo-2-kinase (PFK), pyrophosphate-fructose 6-phosphate 1-phosphotransferase (PFP), glyceraldehyde-3-phosphate dehydrogenase (GAPDH), glyceraldehyde-3-phosphate dehydrogenase (GAPN), phosphoglycerate kinase (PGK), 2,3-bisphosphoglycerate-dependent phosphoglycerate mutase (PGAM), enolase (ENO), and pyruvate kinase (PK), respectively (Table S6). Thus, seed germination required a large amount of energy, which was primarily provided by glycolysis [41]. A TCA cycle, however, would provide energy for germination under oxygen-rich conditions when glycolysis was not sufficient [42]. According to this study, KEGG pathway analysis identified PDHB as the enzyme catalyzing pyruvate’s oxidative decarboxylation to acetyl-CoA. Since they link aerobic oxidation of sugars to the TCA cycle, those enzymes play a crucial role in energy metabolism [43].
Figure 4: Mung bean seed germination reactivates metabolic pathways and produces energy. Glycolysis (A) and TCA cycle (B) are the commonly used pathways for ATP production. These metabolic pathways are represented by transcript expression patterns. Expression levels are represented by a change in color, red indicating a high expression level, green indicating a low expression level. Table S2 detailed these DEGs
The energy metabolism hub, under aerobic conditions, pyruvate dehydrogenase complex (PDC) and alcohol dehydrogenase (ADH) or lactate dehydrogenase (LDH) transform pyruvate into ethanol or lactate (Fig. 4B). The imbibition application activated genes related to PDCs. Meanwhile, the pyruvate can feed the TCA cycle with acetyl-CoA. In Fig. 4B, the genes of TCA cycle enzymes are shown, including malate dehydrogenase (MDH) in mitochondria, citrate synthase (CSY), isocitrate dehydrogenase (IDH), oxoglutarate dehydrogenase complex (OGDC), succinate-CoA ligase (SCoS) and succinate dehydrogenase (SDH) transcripts. In addition to SDH all genes were up-regulated in germinated seeds. A high level of activity for these genes is also observed in many plants during the early germination stage [44]. We need to investigate further the downregulation of SDH transcripts from germinated seeds in this study.
In the seed germination process, GABA, is a non-protein amino acid, especially when there is a large amount of water absorbed [45,46]. As a result of mitochondrial reparation and generations during seed germination, their level elevation may help break dormancy and release malonaldehyde, hydrogen peroxide and superoxide anion [47]. Moreover, glutamate decarboxylase (GAD) activity correlates with accumulation of GABA in soybean seeds during germinating [48]. In germinating seeds, glutamate decarboxylase (GAD), which is the enzyme that enters the aminobutyrate (GABA) shunt, is active and transcript levels increase [49,50]. During mung bean germination, GAD transcript levels were higher, supporting GABA shunt activity (Fig. 4B; Table S2).
4.2 Hormone Biosynthesis and Signaling Transduction during Germination
Plant hormones play an important role in seed germination as internal mediators of development and environment, meanwhile, catabolism and de novo synthesis balance out the amount of endogenous plant hormones [51,52]. Plant development was regulated by ABA and GA, which controlled seed maturation, inhibited germination, and reduced stomatal aperture [51,52]. ABA regulated the accumulation of plant chemicals and controlled the seed dehydration at a later stage of development [53]. ABA is maintained at a relatively high level in dry seeds, especially during germination after rapid decline [54,55]. In addition, the fully decrease of the endogenous ABA content was the important basis for endosperm entirely germination, because ABA restrained the weakening and rupture of the endosperm [56]. According to previous studies, NCED and CYP707A are key enzymes in ABA biosynthesis and catabolism. Therefore, after the seed imbibition, the content of ABA is relatively low due to inhibiting NECD and activating the CYP707A. After seeds absorb water, NCED catalyzes ABA synthesis, whereas CYP707A degrades ABA [57,58]. Inhibition of seed germination is associated with ABA metabolic gene expression levels [59]. In the current study, we found that two CYP707A genes are highly expressed in imbibed seeds (LOC106767139 and LOC106777777) (Fig. 5A, Table S2). Therefore, our results revealed that the CYP707A genes opposed and cyclic transcription during mung bean maturation and germination. CYP707A genes may function as a system to balance the levels of active ABA.
Figure 5: Expression pattern of DEGs and core signaling pathways of the mung bean germination promoting hormones ABA (A), GA (B) and ethylene (C). The names of key enzymes and proteins are shown (red up-regulated, green down-regulated). Table S2 detailed these DEGs
In plants, GA regulates many developmental processes, such as seed germination and seedling development [60,61]. In order to promote radicle protrusion during seed germination, GA can overcome the mechanical constraints of seed coats [62]. In addition, GA3 can promote seed germination in the process of cell division, which is associated with the synthesis and catabolism of GA3 [63]. GA by reducing some germination inhibition of protein to accelerate germination, such as DELLA [64]. The DELLA protein inhibits the growth of plants, while GID1 binds to the GID1 receptor to degrade the DELLA protein [65]. DELLA and GID1 expression levels were lower in germinating seeds than in dormant seeds in the current study (Fig. 5B, Table S2). In addition, these phenomena increased GA levels and contributed to seed germination.
As well as ABA and GA, ethylene also promotes seed germination [66,67]. Ethylene, on the other hand, the regulation of the imbibition period starts very early, by adjusting the metabolism and signal transduction of ABA promote dormancy release and germination [68,69]. Ethylene insensitive 4 (EIN4), ethylene resistant 1 (ETR1), ETR2, ethylene response sensor 1 (ERS1) and ERS2 are the five types of transmembrane receptors that ethylene can bind to at the endoplasmic reticulum. Ethylene can bind to 5 types of transmembrane receptors located at the endoplasmic reticulum, and they are ethylene insensitive 4 (EIN4), ethylene resistant 1 (ETR1), ETR2, ethylene response sensor 1 (ERS1) and ERS2, respectively [70]. In the process of germination, genes from all these families have been a high level transcribed. Ethylene binds to these receptors and activates CTR1, releasing nuclear effectors such as EIN3, EILS, and ERFs by activating kinase cascades. As a result, ethylene response genes are transcriptionally regulated [71]. Lastly, our results confirm the feedback control in the process of mung bean sprout ethylene signal, some negative regulation of ethylene response factors also had high expression, such as CTR1 and EBF (Fig. 5C, Table S2).
4.3 Candidate TFs Associated with Mung Bean Seed Germination
TFs are the key proteins for the regulation of transcriptional machinery. The significantly expressed differential TFs between two groups belonged to the MYB, AP2-EREBP, bHLH and WRKY families (Table S3), which plays an important role in the seed germination and plant growth. In plants, the MYB gene family is one of the biggest, which plays a role in growth, development, and metabolic processes [71]. In our study, a differential upregulation of MYB44 was also observed in germinated seeds. Moreover, we identified 92 significantly up/down regulated MYB transcription factors (Table S3), and the members of MYB44 showed the same expression in the germinated seeds. Hence, MYB might be an important gene for seed germination.
Previous studies showed that AP2-EREBP family members involved in the regulation of plant water absorption. Additionally, their specific expression pattern during germination may have an important regulatory function during water uptake [72]. In our study, the members of AP2-EREBP indicated high expression level in germinated seeds (Table S3). Thus, in the water-uptake phase of germination, these transcription factors with high expression level might be related to an important regulatory role. Among plant TF gene families, bHLH is also one of the largest [73]. The members of the bHLH gene family are seed germination inhibition factor of light stability, which regulates germination of temperature response [74]. In Arabidopsis and rice, phytochrome-interacting factors participate in the signal transduction of light and GA [75]. Many plant functions are regulated by WRKY TFs, including developmental and metabolic functions [76], growth and senescence [77], and stress response [78]. According to the present study, bHLH and WRKY expression levels showed higher in the germinated seeds than in the dry seeds, indicating that these TFs may contribute to seed germination in some ways (Table S3).
4.4 Potential Roles of miRNAs in Seed Germination
Seed germination is the response process of multiple genes with interaction or cooperation, and these genes interacted with other complex hormone signaling network components, such as miRNAs [79,80]. In this study, a total of 23 miRNA-mRNA with negative interaction were investigated through the expression correlation analysis between miRNAs (from small RNA-seq) and their target genes (from RNA-seq) (Table 2). These miRNAs and their target genes play an important role in the process of seed germination.
By down-regulating SPL, from the cotyledon-stage of seedling development, miR156 is essential for vegetative leaf development in Arabidopsis [81,82]. The miR156 gene is down-regulated during seed germination during the imbibition step in maize [83]. MiR156 expression was down-regulated during germination in this study (Table 2). Four SPL proteins were predicted to be targets of miR156 (Table 2), suggesting that miR156 regulates SPL during mung bean seedling development. The miR171-targeted scarecrow-like SCL15 represses embryonic traits in Arabidopsis seedlings [84]. Under light conditions, SCL6/22 proteins coordinate chlorophyll biosynthesis through GA-DELLA signaling [85]. In the present study, miR171b-3p expression was down-regulated in germinated seeds (Table 2). A homolog of SCL6, SCL15, and SCL22 were predicted as the target of miR171b-3p (Table 2), implying that miR171-SCL module may participate in the regulation of embryo-to-seedling phase transition and finetune the GA-regulated chlorophyll biosynthesis during mung bean seed germination.
There is also an important role for miRNAs in response to stress of all kinds. For example, miR169 repressed by salt in Thellungiella salsuginea, however, rice is induced by high salinity and drought [86,87]. MiR169 targeted NF-YA which can encode a subunit of the NF-Y complex transcription factor. This transcription factor related to root growth, nitrogen starvation response, and the plant response to drought and salt stress [88,89]. At present study, miR169-1 was up-regulated in germinated seeds (Table 2). We supposed that miR169-1 was the target gene of NF-YA transcription factor (Table 2), due to miR169 may play a different role in the process of mung bean germination response to drought and salt stress. In plants, HD-ZIP located in the shoot apical meristem (SAM) and root apical meristem (RAM) stem cells form a pool of undifferentiated cells, constantly provide new cells for late embryonic growth [90]. The miR166/165 by negatively control target HD-ZIP, in maintaining the stem and root tip meristem activity is conserved across species [91,92]. In mung bean, miR166e-3p were down-regulated in germinated seeds comparing to the dry seeds (Table 2). There are six HD-ZIP transcription factors predicted to be targets of miR166e-3p (Table 2). This may correspond to a lower meristem activity in the initial stage of germination in mung bean seeds.
In this study, to dissect the complex mechanisms at the earliest stage of seed germination, two approaches including transcriptome and small RNA sequencing were conducted during the water imbibition process of mung bean seeds compared with dry seeds. Out of total DEGs, 129 genes were investigated to involve in ABA, GA, and ethylene pathways, with 56 genes involved in glycolysis and TCA cycle. The integrated analyses of transcriptome and miRNAome data showed that 23 DEGs are the putative target genes of 8 miRNAs including miR156, miR171b-3p, miR166e-3p, miR169-1, and etc. The comprehensive and integrated analysis of these datasets identified DEGs, miRNAs, their targets and also delineated between all these components in the imbibition process of mung bean seeds. It would provide a systematic view of the molecular mechanisms involved in the germination of mung beans.
Funding Statement: This study was funded by the Agricultural Variety Improvement Project of Shandong Province (Grant Nos. 2019LZGC017 and 2021LZGC025), and Modern Agricultural Industrial Technology System of Shandong Province (Grant No. SDAIT-15-01).
Author Contributions: NL and HD conceived the project. YP and LW prepared the data analysis and drafted manuscript. LL, YS, SX, YC, DW, YG, RT, XC, XZ, and ML revised the manuscript. All authors have read and agreed to the published version of the manuscript.
Availability of Data and Materials: Raw sequence data from RNA-seq and small RNA libraries were respectively deposited in the NCBI Sequence Read Archive (SRA, www.ncbi.nlm.nih.gov/sra) under accession numbers PRJNA and PRJNA.
Conflicts of Interest: The authors declare that they have no conflicts of interest to report regarding the present study.
References
1. Hou, D. Z., Yousaf, L., Xue, Y., Hu, J. Y., Wu, J. H. et al. (2013). Mung bean (Vigna radiata L.Bioactive polyphenols, polysaccharides, peptides, and health benefits. Nutrients, 11(6), 1238. https://doi.org/10.3390/nu11061238 [Google Scholar] [PubMed] [CrossRef]
2. Dahiya, P. K., Linnemann, A. R., van Boekel, M. A. J. S., Khetarpaul, N., Grewal, R. B. et al. (2015). Mung bean: Technological and nutritional potential. Critical Review in Food Science and Nutrition, 55(5), 670–688. https://doi.org/10.1080/10408398.2012.671202 [Google Scholar] [PubMed] [CrossRef]
3. Bellieny-Rabelo, D., de Oliveira, E. A. G., Ribeiro, E. S., Costa, E. P., Oliveira, A. E. A. et al. (2016). Transcriptome analysis uncovers key regulatory and metabolic aspects of soybean embryonic axes during germination. Science Reports, 6(1), 36009. https://doi.org/10.1038/srep36009 [Google Scholar] [PubMed] [CrossRef]
4. Kranthi, K. C., Felix, B. F., Song, Q. Y., Hari, B. K., James, R. S. et al. (2016). Impact of heat stress during seed development on soybean seed metabolome. Metabolomics, 12(2), 28. https://doi.org/10.1007/s11306-015-0941-1 [Google Scholar] [CrossRef]
5. Bewley, J. D. (2016). Seed germination and dormancy. The Plant Cell, 9(7), 1055–1066. https://doi.org/10.1016/j.cub.2017.05.050 [Google Scholar] [PubMed] [CrossRef]
6. Holdsworth, M. J., Bentsink, L., Soppe, W. J. J. (2008). Molecular networks regulating Arabidopsis seed maturation, after-ripening, dormancy and germination. New Phytologist, 179(1), 33–54. https://doi.org/10.1111/j.1469-8137.2008.02437x [Google Scholar] [CrossRef]
7. Miransari, M., Smith, D. L. (2014). Plant hormones and seed germination. Environmental and Experimental Botany, 99, 110–121. https://doi.org/10.1016/j.envexpbot.2013.11.005 [Google Scholar] [CrossRef]
8. Kushiro, T., Okamoto, M., Nakabayashi, K., Yamagishi, K., Kitamura, S. et al. (2004). The Arabidopsis cytochrome P450 CYP707A encodes ABA 8′-hydroxylases: Key enzymes in ABA catabolism. EMBO Journal, 23(7), 1647–1656. https://doi.org/10.1038/sj.emboj.7600121 [Google Scholar] [PubMed] [CrossRef]
9. Chiwocha, S. D. S., Cutler, A. J., Abrams, S. R., Ambrose, S. J., Yang, J. et al. (2005). The etr1-2 mutation in Arabidopsis thaliana affects the abscisic acid, auxin, cytokinin and gibberellin metabolic pathways during maintenance of seed dormancy, moist-chilling and germination. The Plant Journal, 42(1), 35–48. https://doi.org/10.1111/j.1365-313X.02359.x [Google Scholar] [CrossRef]
10. Yamagishi, K., Tatematsu, K., Yano, R., Preston, J., Kitamura, S. et al. (2009). CHOTTO1, a double AP2 domain protein of Arabidopsis thaliana, regulates germination and seedling growth under excess supply of glucose and nitrate. Plant and Cell Physiology, 50(2), 330–340. https://doi.org/10.1093/pcp/pcn201 [Google Scholar] [PubMed] [CrossRef]
11. Kinoshita, N., Berr, A., Belin, C., Chappuis, R., Nishizawa, N. K. et al. (2010). Identification of growth insensitive to ABA3 (gia3a recessive mutation affecting ABA signaling for the control of early post-germination growth in Arabidopsis thaliana. Plant and Cell Physiology, 51(2), 239–251. https://doi.org/10.1093/pcp/pcp183 [Google Scholar] [PubMed] [CrossRef]
12. Martin, R. C., Liu, P. P., Nonogaki, H. (2007). Simple purification of small RNAs from seeds and efficient detection of multiple microRNAs expressed in Arabidopsis thaliana and tomato (Lycopersicon esculentum) seeds. Seed Science Research, 15(4), 319–328. https://doi.org/10.1079/ssr2005220 [Google Scholar] [CrossRef]
13. Martin, R. C., Liu, P. P., Nonogaki, H. (2006). microRNAs in seeds: Modified detection techniques and potential applications. Canadian Journal of Botany, 84(2), 189–198. https://doi.org/10.1139/b05-141 [Google Scholar] [CrossRef]
14. Martin, R. C., Asahina, M., Liu, P. P., Kristof, J. R., Coppersmith, J. L. et al. (2010). The regulation of post-germinative transition from the cotyledon to vegetative-leaf stages by microRNA-targeted SQUAMOSA PROMOTER-BINDING PRO-TEIN LIKE13 in Arabidopsis. Seed Science Research, 20(2), 89–96. https://doi.org/10.1017/s0960258510000073 [Google Scholar] [CrossRef]
15. Martin, R. C., Asahina, M., Liu, P. P., Kristof, J. R., Coppersmith, J. L. et al. (2010). The microRNA156 and microRNA172 gene regulation cascades at post-germinative stages in Arabidopsis. Seed Science Research, 20(2), 79–87. https://doi.org/10.1017/s0960258510000085 [Google Scholar] [CrossRef]
16. Liu, P. P., Montgomery, T. A., Fahlgren, N., Kasschau, K. D., Nonogaki, H. et al. (2007). Repression of AUXIN RESPONSE FACTOR10 by microRNA160 is criticalfor seed germination and post-germination stages. The Plant Journal, 52(1), 133–146. https://doi.org/10.1111/j.1365-313X.2007.03218.x [Google Scholar] [PubMed] [CrossRef]
17. Preston, J., Tatematsu, K., Kanno, Y., Hobo, T., Kimura, M. et al. (2009). Temporal expression patterns of hormone metabolism genes during imbibition of Arabidopsis thaliana seeds: A comparative study on dormant and non-dormant accessions. Plant and Cell Physiology, 50(10), 1786–1800. https://doi.org/10.1093/pcp/pcp121 [Google Scholar] [PubMed] [CrossRef]
18. Li, R., Li, Y., Kristiansen, K., Wang, J. (2008). SOAP: Short oligonucleotide alignment program. Bioinformatics, 24(5), 713–714. https://doi.org/10.1093/bioinformatics/btn025 [Google Scholar] [PubMed] [CrossRef]
19. Kim, D., Langmead, B., Salzberg, S. L. (2015). HISAT: A fast spliced aligner with low memory requirements. Nature Methods, 12(4), 357–360. https://doi.org/10.1038/nmeth.3317 [Google Scholar] [PubMed] [CrossRef]
20. Langmead, B., Steven, L. S. (2012). Fast gapped-read alignment with Bowtie 2. Nature Methods, 9(4), 357–359. https://doi.org/10.1038/nmeth.1923 [Google Scholar] [PubMed] [CrossRef]
21. Li, B., Dewey, C. N. (2011). RSEM: Accurate transcript quantification from RNA-Seq data with or without a reference genome. BMC Bioinformatics, 12(1), 323. https://doi.org/10.1186/1471-2105-12-323 [Google Scholar] [PubMed] [CrossRef]
22. Love, M. I., Huber, W., Anders, S. (2014). Moderated estimation of fold change and dispersion for RNA-seq data with DESeq2. Genome Biology, 15(12), 550. https://doi.org/10.1186/s13059-014-0550-8 [Google Scholar] [PubMed] [CrossRef]
23. Abdi, H. (2007). The bonferonni and Šidák corrections for multiple comparisons. Encyclopedia of Measurement and Statistics, 1, 1–9. [Google Scholar]
24. Mortazavi, A., Williams, B. A., McCue, K., Schaeffer, L., Wold, B. (2008). Mapping and quantifying mammalian transcriptomes by RNA-Seq. Nature Methods, 5(7), 621–628. https://doi.org/10.1038/nmeth.1226 [Google Scholar] [PubMed] [CrossRef]
25. Wang, Z., Gerstein, M., Snyder, M. (2009). RNA-Seq: A revolutionary tool for transcriptomics. Nature Reviews Genetics, 10(1), 57–63. https://doi.org/10.1038/nrg2484 [Google Scholar] [PubMed] [CrossRef]
26. Nawrocki, E. P., Burge, S. W., Bateman, A., Daub, J., Eberhardt, R. Y. et al. (2015). Rfam 12.0: Updates to the RNA families database. Nucleic Acids Research, 43(D1), D130–D137. https://doi.org/10.1093/nar/gku1063 [Google Scholar] [PubMed] [CrossRef]
27. Benson, D. A., Cavanaugh, M., Clark, K., Karsch-Mizrachi, I., Lipman, D. J. et al. (2018). GenBank. Nucleic Acids Research, 46(D1), D41–D47. https://doi.org/10.1093/nar/gkx1094 [Google Scholar] [PubMed] [CrossRef]
28. Langmead, B., Schatz, M. C., Lin, J., Pop, M., Salzberg, S. L. (2009). Searching for SNPs with cloud computing. Genome Biology, 10(11), R134. https://doi.org/10.1186/gb-2009-10-11-r134 [Google Scholar] [PubMed] [CrossRef]
29. Kozomara, A., Griffiths-Jones, S. (2014). miRBase: Annotating high confidence microRNAs using deep sequencing data. Nucleic Acids Research, 42(D1), D68–D73. https://doi.org/10.1093/nar/gkt1181 [Google Scholar] [PubMed] [CrossRef]
30. Hofacker, I. L. (2003). Vienna RNA secondary structure server. Nucleic Acids Research, 31(13), 3429–3431. https://doi.org/10.1093/nar/gkg599 [Google Scholar] [PubMed] [CrossRef]
31. Teemu, K., Anna, V., Kasper, K., Martin, B., Martin, E. et al. (2011). Counting absolute numbers of molecules using unique molecular identifiers. Nature Methods, 9(1), 72–74. https://doi.org/10.1038/nmeth.1778 [Google Scholar] [PubMed] [CrossRef]
32. Wang, L., Feng, Z. X., Wang, X., Wang, X. W., Zhang, X. G. et al. (2010). DEGseq: An R package for identifying differentially expressed genes from RNA-seq data. Bioinformatics, 26(1), 136–138. https://doi.org/10.1093/bioinformatics/btp612 [Google Scholar] [PubMed] [CrossRef]
33. Wu, H. J., Ma, Y. K., Chen, T., Wang, M., Wang, X. J. (2012). PsRobot: A web-based plant small RNA meta-analysis toolbox. Nucleic Acids Research, 40(W1), W22–W28. https://doi.org/10.1093/nar/gks554 [Google Scholar] [PubMed] [CrossRef]
34. Fahlgren, N., Carrington, J. C. (2010). miRNA target prediction in plants. Methods in Molecular Biology, 592, 51–57. https://doi.org/10.1007/978-1-60327-005-2 [Google Scholar] [CrossRef]
35. Gotz, S., Garcia-Gomez, J. M., Terol, J., Williams, T. D., Nagaraj, S. H. et al. (2008). High-throughput functional annotation and data mining with the Blast2GO suite. Nucleic Acids Research, 36(10), 3420–3435. https://doi.org/10.1093/nar/gkn176 [Google Scholar] [PubMed] [CrossRef]
36. Kanehisa, M., Araki, M., Goto, S., Hattori, M., Hirakawa, M. et al. (2008). KEGG for linking genomes to life and the environment. Nucleic Acids Research, 36(suppl_1), D480–D484. https://doi.org/10.1093/nar/gkm882 [Google Scholar] [PubMed] [CrossRef]
37. Livak, K. J., Schmittgen, T. D. (2001). Analysis of relative gene expression data using real-time quantitative PCR and the 2−ΔΔCt method. Methods, 25(4), 402–408. https://doi.org/10.1006/meth.2001.1262 [Google Scholar] [PubMed] [CrossRef]
38. Yu, F., Li, M., He, D., Yang, P. (2021). Advances on post-translational modifications involved in seed germination. Front in Plant Science, 12, 642979. https://doi.org/10.3389/fpls.2021.642979 [Google Scholar] [PubMed] [CrossRef]
39. Botha, F. C., Potgieter, G. P., Botha, A. M. (1992). Respiratory metabolism and gene expression during seed germination. Plant Growth Regulation, 11(3), 211–224. https://doi.org/10.1007/BF00024560 [Google Scholar] [CrossRef]
40. Song, Q., Cheng, S., Chen, Z., Nie, G., Xu, F. et al. (2019). Comparative transcriptome analysis revealing the potential mechanism of seed germination stimulated by exogenous gibberellin in Fraxinus hupehensis. BMC Plant Biology, 19(1), 199. https://doi.org/10.1186/s12870-019-1801-3 [Google Scholar] [PubMed] [CrossRef]
41. Yang, P., Liu, X., Wang, X., Chen, H., Chen, F. et al. (2007). Proteomic analysis of rice (Oryza sativa) seeds during germination. Proteomics, 7(18), 3358–3368. https://doi.org/10.1002/pmic.200700207 [Google Scholar] [PubMed] [CrossRef]
42. Yu, Y., Guo, G., Lv, D., Hu, Y., Li, J. et al. (2014). Transcriptome analysis during seed germination of elite Chinese bread wheat cultivar Jimai 20. BMC Plant Biology, 14(1), 20–39. https://doi.org/10.1186/1471-2229-14-20 [Google Scholar] [PubMed] [CrossRef]
43. Li, A., Zhang, Y., Zhao, Z., Wang, M., Zan, L. (2016). Molecular characterization and transcriptional regulation analysis of the bovine PDHB gene. PLoS One, 11(7), e0157445. https://doi.org/10.1371/journal.pone.0157445 [Google Scholar] [PubMed] [CrossRef]
44. Weitbrecht, K., Müller, K., Leubner-Metzger, G. (2011). First off the mark: Early seed germination. Journal Experimental Botany, 62(10), 3289–3309. https://doi.org/10.1093/jxb/err030 [Google Scholar] [PubMed] [CrossRef]
45. Rosental, L., Nonogaki, H., Fait, A. (2014). Activation and regulation of primary metabolism during seed germination. Seed Science Research, 24(1), 1–15. https://doi.org/10.1017/s0960258513000391 [Google Scholar] [CrossRef]
46. Wang, Y., Wu, X., An, Y., Xie, H., Hao, F. et al. (2020). Quantitative metabonomic phenotypes in different structures of mung-bean (Vigna radiata) seeds and their germination-associated dynamic changes. Journal Proteome Research, 19(8), 3352–3363. https://doi.org/10.1021/acs.jproteome.0c00236 [Google Scholar] [PubMed] [CrossRef]
47. Wu, X., Wang, Y., Tang, H. (2020). Quantitative metabonomic analysis reveals the germination-associated dynamic and systemic biochemical changes for mung-bean (Vignaradiata) seeds. Journal Proteome Research, 19(6), 2457–2470. https://doi.org/10.1021/acs.jproteome.0c00181 [Google Scholar] [PubMed] [CrossRef]
48. Guo, Y. X., Yang, R. Q., Chen, H., Song, Y., Gu, Z. X. (2012). Accumulation of gamma-aminobutyric acid in germinated soybean (Glycine max L.) in relation to glutamate decarboxylase and diamine oxidase activity induced by additives under hypoxia. European Food Research and Technology, 234(4), 679–687. https://doi.org/10.1007/s00217-012-1678-y [Google Scholar] [CrossRef]
49. Koster, K. L., Leopold, A. C. (1988). Sugars and desiccation tolerance in seeds. Plant Physiology, 88(3), 829–832. https://doi.org/10.1104/pp.88.3.829 [Google Scholar] [PubMed] [CrossRef]
50. Pracharoenwattana, I., Cornah, J. E., Smith, S. M. (2005). Arabidopsis peroxisomal citrate synthase is required for fatty acid respirationand seed germination. Plant Cell, 17(7), 2037–2048. https://doi.org/10.1105/tpc.105.031856 [Google Scholar] [PubMed] [CrossRef]
51. Wang, H., Guo, X., Li, Q., Lu, Y., Huang, W. et al. (2020). Integrated transcriptomic and metabolic framework for carbon metabolism and plant hormones regulation in Vigna radiata during post-germination seedling growth. Scientific Reports, 10(1), 3745. https://doi.org/10.1038/s41598-020-60771-3 [Google Scholar] [PubMed] [CrossRef]
52. Tuan, P. A., Kumar, R., Rehal, P. K., Toora, P. K., Ayele, B. T. (2018). Molecular mechanisms underlying abscisic acid/gibberellin balance in the control of seed dormancy and germination in cereals. Front in Plant Science, 9, 668. https://doi.org/10.3389/fpls.2018.00668 [Google Scholar] [PubMed] [CrossRef]
53. Tunnacliffe, A., Wise, M. J. (2007). The continuing conundrum of the LEA proteins. Naturwissenschaften, 94(10), 791–812. https://doi.org/10.1007/s00114-007-0254-y [Google Scholar] [PubMed] [CrossRef]
54. Hermann, K., Meinhard, J., Dobrev, P., Linkies, A., Pesek, B. et al. (2007). 1-Aminocyclopropane-1-carboxylic acid and abscisic acid during the germination of sugar beet (Beta vulgaris L.A comparative study of fruits and seeds. Journal Proteome Research, 58(11), 3047–3060. https://doi.org/10.1093/jxb/erm162 [Google Scholar] [PubMed] [CrossRef]
55. Zhu, G., Ye, N., Zhang, J. (2009). Glucose-induced delay of seed germination in rice is mediated by the suppression of ABA catabolism rather than an enhancement of ABA biosynthesis. Plant and Cell Physiology, 50(3), 644–651. https://doi.org/10.1093/pcp/pcp022 [Google Scholar] [PubMed] [CrossRef]
56. Nambara, E., Annie, M. P. (2005). Abscisic acid biosynthesis and catabolism. Annual Review Plant Biology, 56(1), 165–185. https://doi.org/10.1146/annurev.arplant.56.032604.144046 [Google Scholar] [PubMed] [CrossRef]
57. Hwang, S. G., Chen, H. C., Huang, W. Y., Chu, Y. C., Shii, C. T. et al. (2010). Ectopic expression of rice OsNCED3 in Arabidopsis increases ABA level and alters leaf morphology. Plant Science, 178(1), 12–22. https://doi.org/10.1016/j.plantsci.2009.09.014 [Google Scholar] [CrossRef]
58. Lee, H. G., Lee, K., Seo, P. J. (2015). The Arabidopsis MYB96 transcription factor plays a role in seed dormancy. Plant Molecular Biology, 87(4–5), 371–381. https://doi.org/10.1007/s11103-015-0283-4 [Google Scholar] [PubMed] [CrossRef]
59. Sun, J., Wang, P., Zhou, T., Rong, J., Jia, H. et al. (2017). Transcriptome analysis of the effects of shell removal and exogenous gibberellin on germination of Zanthoxylum seeds. Science Reporter, 7(1), 8521–8531. https://doi.org/10.1038/s41598-017-07424-0 [Google Scholar] [PubMed] [CrossRef]
60. Sun, T. P., Gubler, F. (2004). Molecular mechanism of gibberellin signaling in plants. Annual Review Plant Biology, 55(1), 197–223. https://doi.org/10.1146/annurev.arplant.55.031903.141753 [Google Scholar] [PubMed] [CrossRef]
61. Stirk, W. A., Novák, O., Zižková, E., Motyka, V., Strnad, M. et al. (2005). Changes in endogenous cytokinins during germination and seedling establishment of Tagetes minuta L. Plant Growth Regulation, 47(1), 1–7. https://doi.org/10.1146/annurev.arplant.55.031903.141753 [Google Scholar] [CrossRef]
62. Richards, D. E., King, K. E., Tahar, A., Harberd, N. P. (2001). How gibberellin regulates plant growth and development: A molecular genetic analysis of gibberellin signaling. Annual Review Plant Physiology Plant Molecular Biology, 52(1), 67–88. https://doi.org/10.1146/annurev.arplant.52.1.67 [Google Scholar] [PubMed] [CrossRef]
63. Fabian, T., Lorbiecke, R., Umeda, M., Sauter, M. (2000). The cell cycle genes cycA1;1 and cdc2Os-3 are coordinately regulated by gibberellin in planta. Planta, 211(3), 376–383. https://doi.org/10.1007/s004250000295 [Google Scholar] [PubMed] [CrossRef]
64. Hussain, A., Cao, D., Peng, J. (2007). Identification of conserved tyrosine residues important for gibberellin sensitivity of Arabidopsis RGL2 protein. Planta, 226(2), 475–483. https://doi.org/10.1007/s00425-007-0497-z [Google Scholar] [PubMed] [CrossRef]
65. Fu, X., Richards, D. E., Tahar, A., Hynes, L. W., Ougham, H. et al. (2002). Gibberellin-mediated proteasome-dependent degradation of the barley DELLA protein SLN1 repressor. Plant Cell, 14(12), 3191–3200. https://doi.org/10.1105/tpc.006197 [Google Scholar] [PubMed] [CrossRef]
66. Steber, C. M., McCourt, P. (2001). A role for brassinosteroids in germination in Arabidopsis. Plant Physiology, 125(2), 763–769. https://doi.org/10.1104/pp.125.2.763 [Google Scholar] [PubMed] [CrossRef]
67. Gianinetti A., Laarhoven L. J. J., Persijn S. T., Harren F. J. M., Petruzzelli L. (2007). Ethylene production is associated with germination but not seed dormancy in red rice. Annals of Botany, 99(4), 735–745. https://doi.org/10.1093/aob/mcm008 [Google Scholar] [PubMed] [CrossRef]
68. Beaudoin, N., Serizet, C., Gosti, F., Giraudat, J. (2000). Interactions between abscisic acid and ethylene signaling cascades. Plant Cell, 12(7), 1103–1115. https://doi.org/10.1105/tpc.12.7.1103 [Google Scholar] [PubMed] [CrossRef]
69. Corbineau, F., Xia, Q., Bailly, C., El-Maarouf-Bouteau, H. (2014). Ethylene, a key factor in the regulation of seed dormancy. Frontiers in Plant Science, 5, 539. https://doi.org/10.3389/fpls.2014.00539 [Google Scholar] [PubMed] [CrossRef]
70. Waites, R., Selvadurai, H. R., Oliver, I. R., Hudson, A. (1998). The PHANTASTICA gene encodes a MYB transcription factor involved in growth and dorsoventrality of lateral organs in Antirrhinum. Cell, 93(5), 779–789. https://doi.org/10.1016/s0092-8674(00)81439-7 [Google Scholar] [PubMed] [CrossRef]
71. Nguyen, X. C., Hoang, M. H., Kim, H. S., Lee, K., Liu, X. M. et al. (2012). Phosphorylation of the transcriptional regulator MYB44 by mitogen activated protein kinase regulates Arabidopsis seed germination. Biochemical and Biophysical Research Communications, 423(4), 703–708. https://doi.org/10.1016/j.bbrc.2012.06.019 [Google Scholar] [PubMed] [CrossRef]
72. Howell, K. A., Narsai, R., Carroll, A., Ivanova, A., Lohse, M. et al. (2009). Mapping metabolic and transcript temporal switches during germination in rice highlights specific transcription factors and the role of RNA instability in the germination process. Plant Physiology, 149(2), 961–980. https://doi.org/10.1104/pp.108.129874 [Google Scholar] [PubMed] [CrossRef]
73. Toledo-Ortiz, G., Huq, E., Quail, P. H. (2003). The Arabidopsis basic/helix-loop-helix transcription factor family. Plant Cell, 15(8), 1749–1770. https://doi.org/10.1105/tpc.013839 [Google Scholar] [PubMed] [CrossRef]
74. Penfield, S., Josse, E. M., Kannangara, R., Gilday, A. D., Halliday, K. J. et al. (2005). Cold and light control seed germination through the bHLH transcription factor SPATULA. Current Biology, 15(22), 1998–2006. https://doi.org/10.1016/j.cub.2005.11.010 [Google Scholar] [PubMed] [CrossRef]
75. Leivar, P., Monte, E. (2014). PIFs: Systems integrators in plant development. Plant Cell, 26(1), 56–78. https://doi.org/10.1105/tpc.113.120857 [Google Scholar] [PubMed] [CrossRef]
76. Kato, N., Dubouzet, E., Kokabu, Y., Yoshida, S., Taniguchi, Y. et al. (2007). Identification of a WRKY protein as a transcriptional regulator of benzylisoquinoline alkaloid biosynthesis in Coptis japonica. Plant and Cell Physiology, 48(1), 8–18. https://doi.org/10.1093/pcp/pcl041 [Google Scholar] [PubMed] [CrossRef]
77. Miao, Y., Laun, T., Zimmermann, P., Zentgraf, U. (2004). Targets of the WRKY53 transcription factor and its role during leaf senescence in Arabidopsis. Plant Moleculer Biology, 55(6), 853–867. https://doi.org/10.1007/s11103-004-2142-6 [Google Scholar] [PubMed] [CrossRef]
78. Zhou, Q. Y., Tian, A. G., Zou, H. F., Xie, Z. M., Lei, G. et al. (2008). Soybean WRKY type transcription factor genes, GmWRKY13, GmWRKY21, and GmWRKY54, confer differential tolerance to abiotic stresses in transgenic Arabidopsis plants. Plant Biotechnol Journal, 6(5), 486–503. https://doi.org/10.1111/j.1467-7652.2008.00336.x [Google Scholar] [PubMed] [CrossRef]
79. Das, S. S., Karmakar, P., Nandi, A. K., Sanan-Mishra, N. (2015). Small RNA mediated regulation of seed germination. Front in Plant Science, 6, 828. https://doi.org/10.3389/fpls.2015.00828 [Google Scholar] [PubMed] [CrossRef]
80. Martin, R. C., Liu, P. P., Goloviznina, N. A., Nonogaki, H. (2010). microRNA, seeds, and Darwin?: Diverse function of miRNA in seed biology and plant responses tostress. Journal Experimental Botany, 61(9), 2229–2234. https://doi.org/10.1093/jxb/erq063 [Google Scholar] [PubMed] [CrossRef]
81. Hiroyuki, N. (2008). Repression of transcription factors by microRNA during seed germination and postgerminaiton. Plant Signaling & Behavior, 3(1), 65–67. https://doi.org/10.4161/psb.3.1.4918 [Google Scholar] [PubMed] [CrossRef]
82. Madiha, K., Laura, R., Paul, T., Brenda, C. S., Steven, C. et al. (2015). Repression of lateral organ boundary genes by PENNYWISE and POUND-FOOLISH is essential for meristem maintenance and flowering in Arabidopsis. Plant Physiology, 169(3), 2166–2186. https://doi.org/10.1104/pp.15.00915 [Google Scholar] [PubMed] [CrossRef]
83. Li, D., Wang, L., Liu, X., Cui, D., Chen, T. et al. (2013). Deep sequencing of maize small RNAs reveals a diverse set of microRNA in dry and imbibed seeds. PLoS One, 8(1), e55107. https://doi.org/10.1371/journal.pone.0055107 [Google Scholar] [PubMed] [CrossRef]
84. Gao, M. J., Li, X., Huang, J., Gropp, G. M., Gjetvaj, B. et al. (2015). SCARECROW-LIKE15 interacts with HISTONE DEACETYLASE19 and is essential for repressing the seed maturation programme. Nature Communications, 6, 7243. https://doi.org/10.1038/ncomms8243 [Google Scholar] [PubMed] [CrossRef]
85. Ma, Z., Hu, X., Cai, W., Huang, W., Zhou, X. et al. (2014). Arabidopsis miR171-targeted scarecrow-like proteins bind to GT cis-elements and mediate gibberellin-regulated chlorophyll biosynthesis under light conditions. PLoS Genetics, 10(8). https://doi.org/10.1371/journal.pgen.1004519 [Google Scholar] [PubMed] [CrossRef]
86. Zhao, B., Liang, R., Ge, L., Li, W., Xiao, H. et al. (2007). Identification of drought-induced microRNAs in rice. Biochemical Biophysical Research Communications, 354(2), 585–590. https://doi.org/10.1016/j.bbrc.2007.01.022 [Google Scholar] [PubMed] [CrossRef]
87. Zhang, Q., Zhao, C., Li, M., Sun, W., Liu, Y. et al. (2013). Genome-wide identification of Thellungiella salsuginea microRNAs with putative roles in the salt stress response. BMC Plant Biology, 13(1), 180. https://doi.org/10.1186/1471-2229-13-180 [Google Scholar] [PubMed] [CrossRef]
88. Sorin, C., Declerck, M., Christ, A., Blein, T., Ma, L. et al. (2014). A miR169 isoform regulates specific NF-YA targets and root architecture in Arabidopsis. New Phytologist, 202(4), 1197–1211. https://doi.org/10.1111/nph.12735 [Google Scholar] [PubMed] [CrossRef]
89. Ni, Z., Hu, Z., Jiang, Q., Zhang, H. (2013). GmNFYA3, a target gene of miR169, is a positive regulator of plant tolerance to drought stress. Plant Molecular Biololgy, 82(1–2), 113–129. https://doi.org/10.1007/s11103-013-0040-5 [Google Scholar] [PubMed] [CrossRef]
90. Wenig, U., Meyer, S., Stadler, R., Fischer, S., Werner, D. et al. (2013). Identification of MAIN, a factor involved in genome stability in the meristems of Arabidopsis thaliana. Plant Journal, 75, 469–483. https://doi.org/10.1111/tpj.12215 [Google Scholar] [PubMed] [CrossRef]
91. Williams, L., Grigg, S. P., Xie, M., Christensen, S., Fletcher, J. C. (2005). Regulation of Arabidopsis shoot apical meristem and lateral organ formation by microRNA miR166g and its at HD-ZIP target genes. Development, 132(16), 3657–3668. https://doi.org/10.1242/dev.01942 [Google Scholar] [PubMed] [CrossRef]
92. Singh, A., Singh, S., Panigrahi, K. C., Reski, R., Sarkar, A. K. (2014). Balanced activity of microRNA166/165 and its target transcripts from the class III homeodomain leucine zipper family regulates root growth in Arabidopsis thaliana. Plant Cell Reports, 33(6), 945–953. https://doi.org/10.1007/s00299-014-1573-z [Google Scholar] [PubMed] [CrossRef]
Supplementary Materials
Figure S1: The correlation heatmap of each sample of GS and DS. The X and Yaxes represent each sample. The color represents the PCC (the darker the color, the higher the correlation; the lighter the color, the lower the correlation)
Figure S2: GO classification of DEGs in GS and DS. The X-axis represents the number of genes annotated into the GO terms, and the Y-axis represents the functional classification. Green indicating molecular_function, red indicating cellular_component, blue indicating biology_process
Figure S3: Correlation analysis between qRT-PCR and small RNA/mRNA sequencing data based on log2fold change of 15 selected miRNAs and mRNAs
Table S1: Primers used in this study
Table S2: Key DEGs related to seed germination in Mung bean
Table S3: The different expression TFs between DS and GS
Table S4: Summary of small RNA sequencing data generated from 6 small RNA libraries
Table S5: Details of identified miRNAs (known and novel) and their precursors
Table S6: The different miRNAs between DS and GS
Table S7: The targets of different miRNAs
Cite This Article
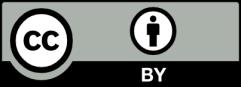