Open Access
ARTICLE
Fruit Ripeness Prediction Based on DNN Feature Induction from Sparse Dataset
1 Department of Statistics, Chonnam National University, Gwangju, 61186, Korea
2 Department of Electronics Eng., Mokpo National University, Muan, 58557, Korea
3 National Program of Excellence in Software Centre, Chosun University, Gwangju, 61452, Korea
* Corresponding Author: In Seop Na. Email:
Computers, Materials & Continua 2021, 69(3), 4003-4024. https://doi.org/10.32604/cmc.2021.018758
Received 20 March 2021; Accepted 03 May 2021; Issue published 24 August 2021
Abstract
Fruit processing devices, that automatically detect the freshness and ripening stages of fruits are very important in precision agriculture. Recently, based on deep learning, many attempts have been made in computer image processing, to monitor the ripening stage of fruits. However, it is time-consuming to acquire images of the various ripening stages to be used for training, and it is difficult to measure the ripening stages of fruits accurately with a small number of images. In this paper, we propose a prediction system that can automatically determine the ripening stage of fruit by a combination of deep neural networks (DNNs) and machine learning (ML) that focus on optimizing them in combination on several image datasets. First, we used eight DNN algorithms to extract the color feature vectors most suitable for classifying them from the observed images representing each ripening stage. Second, we applied seven ML methods to determine the ripening stage of fruits based on the extracted color features. Third, we propose an automatic prediction system that can accurately determine the ripeness in images of various fruits such as strawberries and tomatoes by a combination of the DNN and ML methods. Additionally, we used the transfer learning method to train the proposed system on few image datasets to increase the training speed. Fourth, we experimented to find out which of the various combinations of DNN and ML methods demonstrated excellent classification performance. From the experimental results, a combination of DNNs and multilayer perceptron, or a combination of DNNs and support vector machine or kernel support vector machine generally exhibited excellent classification performance. Conversely, the combination of various DNNs and statistical classification models shows that the overall classification rate is low. Second, in the case of using tomato images, it was found that the classification rate for the combination of various DNNs and ML methods was generally similar to the results obtained for strawberry images.Keywords
Cite This Article
Citations
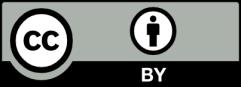