Open Access
ARTICLE
Predicting and Curing Depression Using Long Short Term Memory and Global Vector
1 School of Computer Science and Engineering, Vellore Institute of Technology, Chennai, 600127, India
2 School of Creative Technologies, University of Bolton, Bolton, UK
* Corresponding Author: J. Christy Jackson. Email:
Computers, Materials & Continua 2023, 74(3), 5837-5852. https://doi.org/10.32604/cmc.2023.033431
Received 16 June 2022; Accepted 21 September 2022; Issue published 28 December 2022
Abstract
In today’s world, there are many people suffering from mental health problems such as depression and anxiety. If these conditions are not identified and treated early, they can get worse quickly and have far-reaching negative effects. Unfortunately, many people suffering from these conditions, especially depression and hypertension, are unaware of their existence until the conditions become chronic. Thus, this paper proposes a novel approach using Bi-directional Long Short-Term Memory (Bi-LSTM) algorithm and Global Vector (GloVe) algorithm for the prediction and treatment of these conditions. Smartwatches and fitness bands can be equipped with these algorithms which can share data with a variety of IoT devices and smart systems to better understand and analyze the user’s condition. We compared the accuracy and loss of the training dataset and the validation dataset of the two models namely, Bi-LSTM without a global vector layer and with a global vector layer. It was observed that the model of Bi-LSTM without a global vector layer had an accuracy of 83%, while Bi-LSTM with a global vector layer had an accuracy of 86% with a precision of 86.4%, and an F1 score of 0.861. In addition to providing basic therapies for the treatment of identified cases, our model also helps prevent the deterioration of associated conditions, making our method a real-world solution.Keywords
Cite This Article
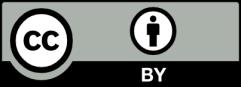