Open Access
ARTICLE
Deep Learning-Enhanced Brain Tumor Prediction via Entropy-Coded BPSO in CIELAB Color Space
1 Department of Computer Engineering, Bahauddin Zakariya University, Multan, 60000, Pakistan
2 Department of Computer Science, Kansas State University, Manhattan, KS, 66506, USA
3 Department of Computer Science, University of Management and Technology, Lahore, 54000, Pakistan
4 Centre for Smart Systems, AI and Cybersecurity, Staffordshire University, Stoke-on-Trent, ST4 2DE, UK
5 Industrial Engineering Department, Collage of Engineering, King Saud University, PO Box 800, Riyadh, 11421, Saudi Arabia
* Corresponding Authors: Muhammad Imran Sharif. Email: ; Hafiz Tayyab Rauf. Email:
Computers, Materials & Continua 2023, 77(2), 2031-2047. https://doi.org/10.32604/cmc.2023.043687
Received 10 July 2023; Accepted 26 September 2023; Issue published 29 November 2023
Abstract
Early detection of brain tumors is critical for effective treatment planning. Identifying tumors in their nascent stages can significantly enhance the chances of patient survival. While there are various types of brain tumors, each with unique characteristics and treatment protocols, tumors are often minuscule during their initial stages, making manual diagnosis challenging, time-consuming, and potentially ambiguous. Current techniques predominantly used in hospitals involve manual detection via MRI scans, which can be costly, error-prone, and time-intensive. An automated system for detecting brain tumors could be pivotal in identifying the disease in its earliest phases. This research applies several data augmentation techniques to enhance the dataset for diagnosis, including rotations of 90 and 180 degrees and inverting along vertical and horizontal axes. The CIELAB color space is employed for tumor image selection and ROI determination. Several deep learning models, such as DarkNet-53 and AlexNet, are applied to extract features from the fully connected layers, following the feature selection using entropy-coded Particle Swarm Optimization (PSO). The selected features are further processed through multiple SVM kernels for classification. This study furthers medical imaging with its automated approach to brain tumor detection, significantly minimizing the time and cost of a manual diagnosis. Our method heightens the possibilities of an earlier tumor identification, creating an avenue for more successful treatment planning and better overall patient outcomes.Keywords
Cite This Article
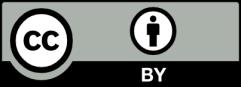