Open Access
ARTICLE
A Novel Soft Clustering Approach for Gene Expression Data
1 A Constituent College of Anna University, University College of Engineering, Villupuram, 605103, India
2 A Constituent College of Anna University, University College of Engineering, Pattukkottai, 614701, India
3 Department of Electronics and Communication Engineering, Dr. N.G.P Institute of Technology, Coimbatore, 641048, India
4 Department of Computer Science Engineering, Faculty of Engineering and Technology, JAIN (Deemed to be University), Bangalore, 562112, India
* Corresponding Author: E. Kavitha. Email:
Computer Systems Science and Engineering 2022, 43(3), 871-886. https://doi.org/10.32604/csse.2022.021215
Received 27 June 2021; Accepted 26 August 2021; Issue published 09 May 2022
Abstract
Gene expression data represents a condition matrix where each row represents the gene and the column shows the condition. Micro array used to detect gene expression in lab for thousands of gene at a time. Genes encode proteins which in turn will dictate the cell function. The production of messenger RNA along with processing the same are the two main stages involved in the process of gene expression. The biological networks complexity added with the volume of data containing imprecision and outliers increases the challenges in dealing with them. Clustering methods are hence essential to identify the patterns present in massive gene data. Many techniques involve hierarchical, partitioning, grid based, density based, model based and soft clustering approaches for dealing with the gene expression data. Understanding the gene regulation and other useful information from this data can be possible only through effective clustering algorithms. Though many methods are discussed in the literature, we concentrate on providing a soft clustering approach for analyzing the gene expression data. The population elements are grouped based on the fuzziness principle and a degree of membership is assigned to all the elements. An improved Fuzzy clustering by Local Approximation of Memberships (FLAME) is proposed in this work which overcomes the limitations of the other approaches while dealing with the non-linear relationships and provide better segregation of biological functions.Keywords
Cite This Article
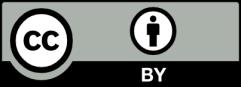